Abstract
A large number of the population faces mortality as an effect of tuberculosis (TB). The line of treatment in the management of TB faces a jolt with ever-increasing multi-drug resistance (DR) cases. Further, the drugs engaged in the treatment of TB are associated with different toxicities, such as renal and hepatic toxicity. Different combinations are sought for effective anti-tuberculosis (anti-TB) effects with a decrease in toxicity. In this regard, drug repurposing has been very promising in improving the efficacy of drugs by enhancement of bioavailability and widening the safety margin. The success in drug repurposing lies in specified binding and inhibition of a particular target in the drug molecule. Different drugs have been repurposed for various ailments like cancer, Alzheimer’s disease, acquired immunodeficiency syndrome (AIDS), hair loss, etc. Repurposing in anti-TB drugs holds great potential too. The use of whole-cell screening assays and the availability of large chemical compounds for testing against Mycobacterium tuberculosis poses a challenge in this development. The target-based discovery of sites has emerged in the form of phenotypic screening as ethionamide R (EthR) and malate synthase inhibitors are similar to pharmaceuticals. In this review, the authors have thoroughly described the drug repurposing techniques on the basis of pharmacogenomics and drug metabolism, pathogen-targeted therapy, host-directed therapy, and bioinformatics approaches for the identification of drugs. Further, the significance of repurposing of drugs elaborated on large databases has been revealed. The role of genomics and network-based methods in drug repurposing has been also discussed in this article.
Keywords
Genomics, drug repurposing, tuberculosis, methodologies, bioinformaticsIntroduction
In 2021, World Health Organization (WHO) reported that 1.3 million persons worldwide lost their lives to tuberculosis (TB). The development of multi drug-resistant-TB (MDR-TB) and extensively drug-resistant-TB (XDR-TB) is preventing the fight against the TB epidemic from progressing, and it is suggested that new tools are urgently required to address this worldwide public health issue. Currently, MDR/XDR patients might take up to two years to recover, and more than half of them do not respond to the available therapeutic options [1]. Resistance is an evolutionary process that is seen with anti-tubercular (anti-TB) medications as well as medications created to combat other diseases. Additionally, the current anti-TB medications are hazardous (hepatotoxicity and renal toxicity are, respectively, shown by fluoroquinolones (FLQ) and aminoglycosides), which discourages adherence and results in subpar treatment outcomes [2]. In order to find new drug combinations for TB that have a shorter regimen, higher efficacy, and lower toxicity, efforts are being done. It is crucial to note that TB medication therapy is a type of combination therapy (CBT) that aims to boost therapeutic effectiveness and fight drug resistance (DR). However, it is challenging to evaluate the bioavailability of medications in CBT due to the intricate interplay of drug-drug interactions. According to studies, rifampicin lowers the bioavailability of a number of other medications when used in a treatment regimen. For instance, it has been shown that the presence of rifampicin lowers the plasma concentrations of bedaquiline [3]. The new TB drug pipeline states that bedaquiline, delamanid, and rifapentine are undergoing market approval studies [4]. There are other different medications and treatment plans in various stages of development. However, the majority of them have shown signs of resistance, indicating the need for the development of modern techniques for the discovery of fresh anti-TB compounds that are drug-like and effective combinations.
Drug repurposing
Identifying new clinical indications/applications for existing drugs have turned out to be a very successful process and become popular in industry and academia. The process of finding new uses outside the scope of the original medical indication for existing drugs is defined as repurposing [5]. Repurposing of drugs reduces the expense of the drug discovery program and enables us to circumvent clinical phase I which is conducted for ensuring safety. Akinpelu et al. [6] defined repurposing with a broader perspective as identifying new indications for existing drugs and failed (demonstrated to have efficacy for a particular indication during phase II or III trials but have no major safety concerns), abandoned, or yet-to-be-pursued as clinical candidates to new disease areas. Drug repurposing is the process of identifying novel medicinal-target interactions of well-established drug therapies with the goal of using them to treat various ailments. Pharmaceutical corporations and academic researchers are both interested in this tactic again because of its potential to lower costs, de-risk clinical trials, and cut down on the time needed to launch a medicine for a new use [7]. However, identifying new clinical use for molecules that fails during the clinical development stage for its original goal (efficacy for a specific clinical condition) is referred to as drug rescue [8]. Techniques for drug discovery and repurposing primarily rely on high-throughput chemical screens. However, a repurposing screen and a drug discovery screen differ significantly from one another. Small libraries of failed or authorized compounds with some understanding of their safety or mechanism of action (MoA) are used for experimental screening to find a repurposing candidate. On the other hand, drug discovery screens look for fresh candidates (hits) utilizing specialized screening equipment and compound libraries comprising millions of compounds [9]. In contrast to conventional methodologies, in silico approaches and high-performance computing offer an interesting alternative to finding new or repurposed candidates given the high development costs and high attrition rates [10]. Pharmaceutical corporations and academic researchers are both interested in drug repurposing because of its potential to lower costs, de-risk clinical trials, and cut down on the time needed to launch a medicine for a new use. To date, numerous medications have been effectively repurposed. One of these was thalidomide, which was initially approved for the treatment of multiple myeloma and has since been repurposed for the treatment of leprosy. Thalidomide was first used as a morning sickness medication with terrible outcomes. It has also been effective in treating severe side effects of treatment in children with TB meningitis.
Repurposing of drugs: success stories
Repurposing of known drugs has been widely used in identifying new clinical applications for various diseases including cancer (metformin), Alzheimer’s disease (galantamine), Parkinson’s disease (ropinirole), acquired immunodeficiency syndrome (AIDS; zidovudine), erectile dysfunction (sildenafil) hair loss (finasteride), stress urinary incontinence (duloxetine), etc. [11]. Repurposing of drugs has also benefited the treatment of TB, and many more drugs are being investigated, and appreciating the potential use of repurposing, government agencies viz., National Institute of Health (USA) and Medical Research Council (UK) have launched collaborative research to identify new indications for selected compounds from private pharmaceutical compounds [12].
The basic mechanism of drugs, “specifically binding and inhibiting a particular target” which is well known by the metaphor of drugs, acting as “magic bullets”; however, recent findings and understanding about drugs which modulate more than one target led to the concept of polypharmacology [13]. Though the promiscuous nature of drugs would cause unexpected adverse reactions, rational selection and evaluation of drugs would help us in identifying safe and novel use for the existing drugs for other clinical conditions [14]. Therefore, polypharmacology is the basic principle behind the repurposing of drugs for other clinical conditions. Interest has grown to adopt the repurposing approach for TB as several drugs have already been repurposed successfully. Lead compounds do, however, lose a lot of ground when they move from preclinical to phase I clinical investigations. Because of this, there aren’t as many unique compounds available for future research and inclusion in effective anti-TB therapies [15]. Among them, FLQ (levofloxacin, moxifloxacin, gatifloxacin, and ofloxacin) are an important group of repurposed drugs for the treatment of MDR-TB; clofazimine, meropenem, and clavulanate have been repurposed successfully against XDR-TB. Several more drugs including statins [15], metformin [16], doxycycline [17], and valproic acid [18] have been identified to have potential use in TB in the early phases of research, which indicate the promising applications of repurposing of drugs for TB.
TB drug repurposing
The discovery of TB targets and medication repurposing strategies have already been the subject of numerous techniques and resources. The methods recently established for identifying drug candidates for TB are the main topic of this review. In order to find new anti-TB medications, either phenotypic or target-based screens are used [19]. The first method relies on the creation of whole-cell screening assays and the availability of a library of chemical compounds for use against Mycobacterium tuberculosis (Mtb) that is both reproducing and not growing. Purification of the target protein, further compound screening assays against the target, and in vivo target validation are all steps in the target-based screening approach. Target-based drug discovery has had less success than phenotypic screening, with a few examples include the discovery of ethionamide R (EthR) and malate synthase inhibitors that are similar to pharmaceuticals. Auranofin, nitazoxanide, clofazamine, the bedaquiline + pretomanid + linezolid (BPaL) and pretomanid + moxifloxacin + pyrazinamide (PaMZ) combos, and a few other TB medications now under development are repurposed prospects. These medications were first discovered for a different indication than TB. For instance, the antirheumatic drug auranofin has demonstrated a wide range of activities, including anti-TB activity. The antiprotozoal drug nitazoxanide is also used to treat TB. Numerous medications, including beta-lactams (meropenem and clavulanate), FLQ (gatifloxacin and moxifloxacin), rifamycins (rifapentine and clofazimine), oxazolinones (linezolid and sutezolid), and rifamycins (rifapentine and clofazimine), have been repurposed to treat MDR [20]. Metronidazole and linezolid have finished phase II clinical trials, while gatifloxacin has finished phase III testing [21]. Cephalosporins have lately demonstrated promising synergistic effect when used with rifampicin and other anti-TB medications including bedaquline and delamanid [22]. In addition to the anti-infectives, other substances have also been applied to TB. Thioridazine and chlorpromazine are medications used to treat psychoses, whereas nonsteroidal anti-inflammatory drugs (NSAIDs) including diclofenac, ibuprofen, and carprofen also have anti-TB activity [23]. Entacapone and tolcapone, which work as adjuncts to the therapy of Parkinson’s disease, are also included [24]. Several computer techniques have also been developed that have found prospective drug repurposing candidates for TB in order to systematically examine the drug-target space. As previously indicated, researchers found employing a virtual screening strategy based on docking, to identify drug candidates against Mtb murine extensive tuberculosis (MurE) and protein kinase B (PknB). Additionally, it has been reported the identification of polypharmacological medicines as well as repurposing possibilities against Mtb proteins [25]. The three-dimensional (3D) structure of the target and/or ligand must be available for these drug repurposing techniques to be effective, though. To predict drug-target interactions (DTIs), network pharmacology-based methods such as pharmaco-chemical-genomics, random walk, gene expression, and network-based analysis have been utilized [26]. The network-based inference (NBI) technique was more recently employed to predict DTIs, however, it has not been used to find candidates for TB repurposing [27].
Pharmacogenomics and drug metabolism in TB
The emergence of drug-resistant-TB (DR-TB) is influenced by numerous factors. These can be ascribed to the Mtb resistance mechanisms or may alternatively be brought on by a variety of host variables [28]. Pathogenesis results from interactions between hosts and pathogens and depends on the genetic makeup of both [29]. There is a wealth of information available regarding various treatment outcomes depending on an individual’s genetic makeup. Individuals can be classified as slow or rapid metabolizers based on their genetic variants, which have a substantial impact on drug-metabolizing enzymes such as cytochrome P450s (CYP). For instance, polymorphisms in the N-acetyltransferase-2 (NAT2) enzyme (rs1801280, rs1799929, rs1208, rs1041983, and rs1799930) are known to indicate genotypes of slow acetylators that are more likely to experience unfavourable drug reactions [30]. Additionally, differences in solute carrier organic anion transporter 1B1 (SLCO1B1) associated with lower rifampicin bioavailability were more evident in African subjects than in USA and Spanish participants [31]. The development of more efficient treatment regimens depends critically on the identification of differences that influence drug metabolism in various populations.
Current status of repurposing of drugs for TB
Encouraged by the success of the repurposing of drugs in various clinical conditions, interest has grown globally to adopt the principle of the repurposing. Though the repurposing of drugs for TB is not new, researchers have taken effort to evaluate the usefulness of other drugs for TB with much more interest recently. Potential exists for using this approach to treat TB, particularly DR-TB. A number of medications that have been approved for clinical use or clinical studies, including linezolid, vancomycin, and celecoxib, have been discovered to have anti-TB properties recently [32]. The basic principle of repurposing of drugs for TB could be divided into two broad categories, (1) pathogen-targeted therapy and (2) host-directed therapy (HDT) as these drugs target proteins of either the pathogen or modulate the host-target respectively [33].
Pathogen-targeted therapy
The primary mechanism of drugs used to treat infectious diseases including TB is that these drugs inhibit proteins encoded by the specific pathogen, target its critical biological function and subsequently cause the lethal effect to the pathogen. Currently, there are about 28 different drugs used in various combinations for the treatment of TB [34]. Interestingly, all of them are known to target only genes/proteins encoded by Mtb.
Those antibiotics which are useful to treat multiple diseases are referred to as broad-spectrum. In general, broad-spectrum drugs target orthologous genes/proteins, which are present in many species but perform similar biochemical/molecular functions. Therefore, orthologous proteins serve as the common drug-target for broad-spectrum drugs. For example, FLQ (levofloxacin, moxifloxacin, ofloxacin, gatifloxacin) are broad-spectrum drugs effectively used in the treatment of TB, and different Gram-positive and Gram-negative bacterial diseases. FLQ were initially discovered for malaria in 1962. Later only in the 1980s, quinolones were demonstrated to be useful for the treatment of TB [35]. A new generation of FLQ, ciprofloxacin, and moxifloxacin have also been reported to be useful for MDR-TB. The basic mechanism of FLQ is that it inhibits the DNA gyrase of the pathogen. In Mtb, DNA gyrase is encoded by DNA gyrase subunit A (gyrA; Rv0005) and DNA gyrase subunit B (gyrB; Rv0004). The FLQ causes bactericidal effects in Mtb. FLQ are used as a second-line drug in the treatment of TB, and they are crucial in the treatment of MDR-TB.
Beta-lactam based drugs are broad-spectrum and used in the treatment of various bacterial diseases. However, it was not used for TB since Mtb encodes beta-lactamase enzyme, which degrades the beta-lactam based drugs. But, an effective strategy was employed recently to use the beta-lactam based drugs against TB. It was demonstrated that in combination with clavulanate, meropenem inhibited the penicillin-binding protein (PBP) and mycobacterial growth. In this case, clavulanate was used to inhibit mycobacterial beta-lactamase enzyme, thus protecting the beta-lactam drug, meropenem from degradation. Therefore, meropenem-clavulanate combination is used along with other anti-TB drugs in the treatment of DR-TB [36]. Linezolid, a synthetic oxazolidinone derivative initially used for nosocomial pneumonia and skin infections caused by Gram-positive bacteria was successfully repurposed for the treatment of MDR-TB and XDR-TB as it is listed as group 5 drugs for TB by WHO [37]. Linezolid is reported to inhibit protein synthesis of Mtb by binding to the ribosomal peptidyl transferase center (PTC) [38]. Although clofazamine was originally identified for the use of TB, due to observed toxic profile and availability of better drugs then, it was not utilized for TB; however, it was found useful in the treatment of leprosy. Due to the emerging DR-TB, researchers revisited clofazimine and reinvestigated its use successfully for DR-TB [39]. Clofazimine has been found effective in treating DR-TB, and it is listed in the group 5 of the drugs for TB by WHO. The exact mechanism and target gene of clofazimine in TB is not known. However, a possible mechanism proposed is that the activated clofazamine leads to the release of reactive oxygen species (ROS) causing bacterial death [40]. Another proposed mechanism is that clofazimine acts as a membrane-destabilizing agent leading to the dysfunction of membrane transporters [41].
HDT
The major mechanism of HDT is that the drugs enhance the host immune response against pathogen and/or reduce the pathology of the disease. Furthermore, some of the repurposed drugs, including verapamil and metformin exhibit synergistic effects with anti-TB drugs and accelerate the killing of the bacteria [42]. Thus, HDT holds several advantages including potential to reduce the treatment duration for TB and prevent the emergence of DR as the HDT does not target directly the pathogen [43]. Statins are the first choice of treatment in lowering the 48 cholesterol among patients with cardiovascular disease events [44]. Statins (simvastatin and rosuvastatin) have been demonstrated to reduce cholesterol levels within phagosomal membranes and enhance autophagy and phagosome maturation resulting in significant reduction in Mtb growth [45]. Later, simvastatin was found to increase the TB activity of isoniazid in the J774 macrophages cell line and its inclusion in the regimen of rifampicin, isoniazid, and pyrazinamide increased bacterial killing and formation of colony-forming units in mice [46].
Bioinformatics approaches for identification of drugs for repurposing
Various approaches are employed for the identifications of drugs for repurposing including, in silico based virtual screening, in vitro screening/testing of drugs against selected clinical conditions, knowledge or mechanism based screening of drugs. Here, the authors have tried to focus on in silico based repurposing, basic principles of bioinformatics approaches which helps to identify drugs with potential for repurposing. Cross-reactivity of drugs between different target families was explored among complex protein-ligand interaction networks on a proteome-wide scale with the help of a systematic computational approach; the whole process could be summarized as comparison of the binding site of a commercially available drugs with that of ligand binding sites of the proteins of the Mtb, molecular docking (protein-ligand docking) and subsequently shortlist the drugs with potential for repurposing. By employing this identified entacapone and tolcapone, which are primarily used in the treatment of Parkinson’s disease, to have in vitro activity against Mtb [47]. Further, they constructed a proteome-wide drug-target network-based on the comparison of the binding sites of the Food and Drug Administration (FDA) approved drugs with the entire structural proteome of the Mtb and identified new drug-targets (TB-drugome). Subsequently, they performed molecular docking for each identified drug-target pair and shortlisted 123 FDA-approved drugs as having the potential for repurposing for TB [48]. Later on, more studies have demonstrated the usefulness of drugs for repurposing by employing different protocols based on the principle of similarities between binding sites of known drug-targets and proteins of Mtb. For example, the researchers [49] revisited the TB drug for analysis using updated protein structural information and found that tamoxifen, which is used in the treatment and prevention of estrogen receptor-positive breast cancer, to has in vitro activity against Mtb. Structural details of the binding sites at a genome-scale (pocketome) of Mtb and drug binding sites of approved drugs combined with molecular docking were used to identify 27 drugs as the potential for repurposing [50]. Evolutionary conservation at the level of sequence and structure of targets of FDA-approved drugs after excluding drugs known to act on human proteins, and proteins from Mtb and subsequent docking was employed to identify 130 drugs as promising candidates for repurposing for TB [51]. These studies indicate that various bioinformatics approaches can be used successfully to identify and/or prioritize known drugs with the potential for repurposing for TB.
Repurposing drugs with big data
With the accumulation of the large volumes of “omics” data, in silico approaches play an increasingly important role in the discovery of novel antibiotics as well as the repurposing of known drugs for new indications. Over time, biological and chemical information has been generated at an ever-increasing pace, indicating the entrance of the so-called “big data” era [52]. There are many in silico tools that may analyse the structural and physico-chemical characteristics of compounds that are in the pre-clinical stage and identify potential uses for them thanks to the rapidly growing discipline of cheminformatics [53]. Protein-protein interactions, gene expression data, and data extracted from literature using text-mining [54]. Among other sources to identify hidden associations between biological entities. Several in silico techniques are used and being developed to take advantage of the constantly growing target and inhibitor space for the discovery of potential drug repurposing candidates. Additionally, efforts are made to broaden the target and chemical space, which may enable the discovery of novel compounds that fit the intended target product profile.
Strategies for drug repurposing based on genomics
The principle of polypharmacology, in which medications bind to several targets, provides the foundation for drug repurposing. Therefore, it is crucial to establish new drug-disease (and/or target) connections before exploring new target and chemical space. It is crucial that one has a thorough understanding of genotype-phenotype relationships in this situation. To this end, the collection of genes expressing druggable targets provides a wealth of information to investigate novel relationships and foretell probable future associations. A wide range of connected data from high-throughput microarray and next-gen sequencing investigations is available through resources like the National Center for Biotechnology Information Gene Expression Omnibus (NCBI-GEO). It is a global repository with 2,467,298 samples and 4,348 datasets that can be searched for raw, processed, or meta-data that may be used to identify drug-target connections [55]. CMAP technology has been utilised more recently to find shared disease-related gene expression profiles. There are 27,927 perturbagens in the CMAP repository whose profiles produced 476,251 expression signatures [56].
Thereafter, drug directionality map (DMAP), which is a drug-protein CMAP with a total of 438,004 chemical-to-protein effect relationships, was constructed [57]. These resources are used for predicting drug repurposing candidates against cancer cell lines (DeSigN, ksRepo), Parkinson’s disease, asthma, hypertension, inflammatory diseases, etc. [58]. The available genomics based resources available for drug repurposing is listed in Table 1.
Genomics based platforms for drug repurposing
No. | Tools | Description |
---|---|---|
1 | National Center for Biotechnology Information Gene Expression Omnibus | Provides microarray, NGS, and other functional genomic data. |
2 | Connectivity map | Resource for genome-wide transcriptional expression data from cultured human cells treated with bioactive small molecules to identify connections between drugs, genes, and diseases. |
3 | Gene2Drug | Integrates drug-gene expression signatures and information from pathway databases. |
4 | DeSigN | Predicts drug efficacy against cancer cell line using gene expression. |
5 | DSigDB | Resource that maps the drugs to their targets for GSEA. |
6 | ksRepo | Universal computational tools for drug repurposing. |
7 | DiseaseConnect | Resource to identify disease-disease relationships using gene expression data. |
8 | Cogena | Framework for co-expression analysis of gene expression signatures and GSEA of co-expressed genes. |
9 | SPIEDw | Web tool designed to facilitate fast and simple quantitative querying of publicly available gene expression data. |
10 | NFFinder | Uses transcriptomic data to find relationships between drugs, diseases, and a phenotype of interest. |
11 | PharmGKB | Pharmacogenomics resource for adjusting drug treatments. |
No.: number; CMAP: connectivity map; NGS: next generation sequencing; DsigDB: drug signatures database; GSEA: gene set enrichment analysis; SPIEDw: searchable platform-independent expression database web version; PharmGKB: pharmacogenomics knowledgebase
Network-based methods for repurposing drugs
There is a lot of use of network-based algorithms to find new DTIs. Based on network topology similarity, a drug repurposing algorithm ligand Enrichment of Network Topological Similarity (ligENTS) was created to find novel DTIs [59, 60]. Similar to this, Sambarey et al. [61] used a multi-layered network to find candidates for undiagnosed disorders. Utilizing the similarity between chemicals’ two-dimensional (2D) fingerprints, the first layer is produced. Nodes representing the proteins from 221 pathogens and non-pathogen species make up the second layer. The nodes in the third layer are shown as protein families (Pfam) domains and ortholog groups. include metabolic processes. Sequence analysis is used to establish the relationships between layer 2 and layer 3 whereas bioactivity data is used to build the links between layer 2 and layer 1. This work was used as a case study to find possibilities for drug repurposing as well as druggable homologs in Plasmodium falciparum (P. falciparum). A weighted drug-disease heterogeneous network employing known disease-gene and drug-target networks was published by Jin et al. [60] in a different study. Network clustering was used to find novel disease-drugs modules. Networks that are used to anticipate new DTIs can be created using other resources like STITCH [62], a database resource that gives data on chemical-protein interactions. Other network-based algorithms may be tailored to combat infectious diseases but are restricted to drug repurposing in human diseases. As an illustration, employed the NBI method to predict DTIs that can be used to discover candidates for repurposing against human targets and infectious disease targets as well [61, 62].
Since, most network-based algorithms predict a binary state of interaction and determine whether a drug interacts with a protein or not, providing additional information about DTIs, such as “direct or indirect interaction” or “activation or inhibition” can help with the prediction of new DTIs. In this context, the authors applied a machine-learning approach known as the limited Boltzmann machine to create a multidimensional DTI network [restricted Boltzmann machine (RBM)] [63]. Similar to this, also created a medication repurposing framework based on support vector machines (SVM) using integrated data and forecasted drug repositioning candidates for several therapeutic classes [64]. A selection of resources that use network-based techniques that can be used for drug repurposing is provided in Table 2.
Network-based resources for drug repurposing
No. | Tools | Description |
---|---|---|
1 | MANTRA | Tool to identify DR candidates by analyzing the MoA |
2 | STITCH | Database for physical and functional associations between chemical and targets |
3 | DGIdb | Resource for browsing, searching, and filtering information on drug-gene interactions and the druggable genome |
4 | PDOD | DR resource for predicting drugs with opposite effects on altered states of disease genes |
5 | DeMAND | DR resource for MoA analysis |
6 | DMAP | CMAP of drug-protein effects |
DR: drug-resistant; DGIdb: drug-gene interaction database; PDOD: pulsed dose oxygen delivery; DeMAND: detecting mechanism of action by network dysregulation
Drug repurposing methods based on structural proteomics
If the binding sites of two targets are similar, a medication may bind to both of them. A variety of computational techniques have been created to analyze DTIs and gauge how similar binding sites are in proteins. By comparing nearly 90,000 putative binding pockets found in 3,700 human proteins, Luo et al. [65] showed how the discovery of similar binding sites expands the space of possibilities for logical drug design. They also identified 23,000 protein pairs that had at least one similar cavity where similar ligands can bind.
The possibility of poly-pharmacology resulting from ligand interactions with completely different residues in the off-target was examined [66]. In 116 human protein-ligand complexes, where the protein pairings were unrelated by fold but bound the same ligand, they looked at the structures of 59 ligands. They deduced that recognizing binding sites in unrelated proteins that bind identical ligands does not require a single pattern-matching “code”, and that identifying various ligand groups only requires a modest pattern of recognition sites. In order to create new therapies to combat medication resistance, it is essential to comprehend how mutations alter ligand binding to targets. To assess how mutations affect the evolution of DR, many platforms are being explored. Graph-based signatures are used by DynaMut to forecast and analyze the effects of mutations on the stability of proteins. Similar to this, mCSM-lig uses graph-based structural signatures to forecast how single-point missense mutations may affect molecule binding affinity [66].
Docking and inverse docking are two structure-based methods for discovering new DTIs. The construction of ligands and the production of many conformers that are each docked to the binding pocket of the target are the key components of the docking process. As demonstrated in the instance of Mtb MurE, where three medications, lymecycline, acarbose, and desmopressin, were identified as prospective repurposed candidates from 1,554 FDA-approved drugs, this approach may be utilized systematically for predicting drug repurposing prospects [67]. On the other side, inverse docking looks at how a known medicine binds to a panel of known targets [68].
A single target’s inhibition may have an impact on numerous cellular processes. However, effective biological network shutdown is frequently accomplished through polypharmacology by focusing on numerous proteins [69]. Understanding off-target and polypharmacological pathways at the system level can open up new possibilities for medication repurposing. This idea has been used in numerous researches to locate potential targets for Mtb target proteins [70]. To find antiviral medications like atazanavir, indinavir, and lopinavir against Mtb proteins (Plipastatin synthase subunit E) PpsE (Rv2935), Rv2842c, Rv2689c, and MurE (Rv2158c), one of these strategies used structural proteomics [71]. The publicly available sources of drug repurposing are represented in Figure 1.
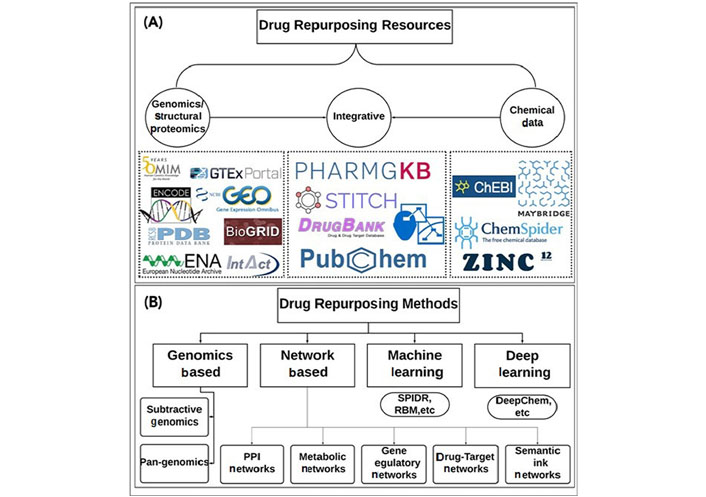
Publicly available data sources and tools for drug repurposing. (A) Depicts the available public resources that provide genomics and structural proteomics data [e.g., NCBI-GEO, Palm DataBase (PDB), Online Mendelian Inheritance in Man (OMIM), Genotype-Tissue Expression (GTEx), IntAct Molecular Interaction Database (IntAct), etc.], Chemical structure data [e.g., ChemSpider, Zinc12, chebi Chemical Entities of Biological Interes (ChEBI), Maybridge (Thermo Scientific™), etc.], and integrative data (e.g., DrugBank, PharmGKB, STITCH, Concept Maps Cmap, Pubchem, etc.); (B) depicts the drug repurposing methods that use the data from the resources mentioned in (A) and apply different strategies to extend either the target space or the chemical space for new DTIs; the methods can roughly be divided into genomics based methods (e.g., subtractive genomics, pan-genomics), network-based methods (e.g., protein-protein interaction (PPI) networks, metabolic networks, gene regulatory networks, drug-target interaction networks, semantic link networks, etc), machine learning methods (e.g., SPIDR, RBM, etc.), and deep learning methods (e.g., DeepChem, etc.).
Nanotechnology based approaches for DR in TB
MDR-TB is able to originate and spread swiftly because of insufficient or inconsistent therapy. DR-TB patients now struggle to survive since, the treatment takes over two years and is so complicated, expensive, and hazardous. Numerous novel anti-TB medications are in development, but they have a number of significant problems, including poor research, high cost, difficulties in targeting MDR and dormant bacteria, and drug toxicity. These are the factors that motivate the search for novel, distinctive alternative medicines for therapeutic purposes. In the recent decade, nanoparticle-based therapy may be superior to traditional therapy for treating TB since it has the potential to reduce medication requirements and boost patient compliance [72]. Additionally, nanoparticulate systems have inherent antimycobacterial activity and operate as a delivery method for different anti-TB medications, helping to decrease the frequency of treatment and the accompanying adverse effects [73]. A suitable nanotechnology approach has the potential to enhance the tolerability of toxic chemotherapy, provide prolonged and regulated drug release, and eventually increase bioavailability and reduce drug resistance and emergence. Nanoparticles are frequently taken up by a variety of mechanisms, including (1) transcytosis through M-cells, (2) intracellular uptake and transport via intestinal mucosal epithelial cells, and (3) uptake by Peyer’s patches [74]. Drugs released from nanoparticles are stable and released slowly, making oral administration possible. The effectiveness of anti-TB medications, when taken orally, has been confirmed by Pandey et al. [75]. Rifampin, isoniazid, and pyrazinamide, the three effective anti-TB medications, were used to treat TB. These medications were made using the solvent evaporation method and the double emulsion procedure, and poly (lactide-co-glycolide) nanoscale particles (PLG NPs) were used to encapsulate them [75]. After a single oral injection of drug-loaded PLG NPs, drug levels were kept above the least inhibitory concentration (MIC90) in mice for 6 days to 9 days in the plasma and were able to reduce the emergence of resistance in active TB strain [75]. Moreover, copper (II) ion was observed as a carrier for capreomycin and observed the efficacy of about 250 folds inhibition against DR strains of TB as compared to capreomycin alone [76].
Conclusions
The progress in the generation of different databases has led to the advanced management of diseases as far as the pharmacological therapy is concerned. The use of drugs proven in specific diseases has been restructured while targeting other ailments. The additional benefit resides in the reduction in toxicity profiles and improved therapeutic benefits. In TB, the restructured system relies on pathogen-targeted and host-directed therapies as the anti-TB agent influences the protein chains of the affected pathogen or alters the host cells. In silico bioinformatics too plays an important role in restructuring anti-TB treatment. Further, pharmacogenomics and metabolism of drugs decide the fate of restructured therapy. The engagement of genomic tools like Gene Expression Omnibus (GEO) and DeSigN plays a significant role in the prediction of drug efficacy against cancer cell lines using gene expression. Similarly, network-based resources like MANTRA provides information to identify DR candidates by analyzing the MoA. On a similar platform, gene regulatory networks, drug-target networks, and semantic link networks provide a public access to drug restructuring. There is a vast interest in further research of drug restructuring while drawing great benefits in upcoming future.
Abbreviations
anti-TB: | anti-tubercular |
CMAP: | connectivity map |
DR: | drug resistance |
DR-TB: | drug-resistant-tuberculosis |
DTIs: | drug-target interactions |
FDA: | Food and Drug Administration |
FLQ: | fluoroquinolones |
HDT: | host-directed therapy |
MDR-TB: | multi drug-resistant-tuberculosis |
MoA: | mechanism of action |
Mtb: | Mycobacterium tuberculosis |
MurE: | murine extensive tuberculosis |
TB: | tuberculosis |
WHO: | World Health Organization |
XDR-TB: | extensively drug-resistant-tuberculosis |
Declarations
Acknowledgments
The authors are highly thankful to ISF College of Pharmacy, Moga for providing necessary facilities and encouragement.
Author contributions
PAC: Conceptualization, Writing—original draft. DS: Writing—original draft. AS: Writing—original draft. All authors read and approved the submitted version.
Conflicts of interest
The authors declare that they have no conflicts of interest.
Ethical approval
Not applicable.
Consent to participate
Not applicable.
Consent to publication
Not applicable.
Availability of data and materials
Not applicable.
Funding
Not applicable.
Copyright
© The Author(s) 2023.