Abstract
Aim:
Breast cancer (BC) is the most common malignancy among women and a leading cause of cancer-related mortality. Early detection and prediction are crucial for prognosis and targeted therapy selection. This study investigates differences in BC gene expression between European and Asian populations by analysing differentially expressed genes (DEGs) and identifying potential biomarkers for diagnosis and treatment.
Methods:
This study analyzed gene expression datasets from the NCBI Gene Expression Omnibus (GEO), including GSE15852 (Malaysia), GSE29044 (Saudi Arabia), GSE89116 (India), GSE61304 (Singapore), GSE29431 (Spain), GSE21422 (Germany), and GSE42568 (Ireland). DEGs were identified using GEO2R, with significance thresholds set at p < 0.05 and logFC > 2.0. Protein-protein interaction (PPI) networks were constructed using STRING and analyzed in Cytoscape, helping in identification of highly upregulated biomarker (HUB) genes. Functional enrichment was conducted using Enrichr-KG and GeneMANIA to explore pathway associations.
Results:
Two common HUB genes, cluster of differentiation 36 (CD36) and leptin (LEP), were identified across five datasets, suggesting their universal relevance in BC. Additionally, caveolin-1 (CAV1) and perilipin 1 (PLIN1) were significant in the Asian datasets, while CAV1, insulin-like growth factor 1 (IGF1), apolipoprotein B (APOB), and peroxisome proliferator-activated receptor gamma (PPARG) were HUB genes in European datasets. Functional pathway analysis revealed that these genes are primarily involved in cholesterol metabolism, adipocytokine signaling, AMP-activated protein kinase (AMPK) regulation, and fatty acid metabolism, highlighting their role in BC progression.
Conclusions:
CD36 and LEP are universal biomarkers with potential diagnostic and prognostic significance in BC. Region-specific HUB genes emphasize the need for precision medicine in treatment. Their role in cholesterol metabolism and adipocytokine signaling suggests potential therapeutic targets. CD36 and LEP could be used in liquid biopsy screening, and their metabolic function supports further investigation into CD36 inhibitors, LEP antagonists, and PPARG modulators. Future studies should focus on large-scale validation and multi-omics approaches for personalized BC management.
Keywords
Breast cancer, differentially expressed genes (DEGs), Asian, European, biomarkers, highly upregulated biomarker (HUB) genesIntroduction
Breast cancer (BC) is the most frequently diagnosed cancer and leading cause of cancer-related mortality among women globally, with over 2.3 million new cases [1]. Despite significant advances in diagnostic and therapeutic lifestyle, and environmental factors, this heterogeneity poses a challenge for developing universally effective treatments and underscores the need for region-specific studies [2].
Several factors can increase the risk of developing BC, including aging, obesity, excessive alcohol consumption, family history of BC, exposure to radiation, reproductive history (such as the age of menstruation onset and age at first pregnancy), tobacco use, and postmenopausal hormone therapy. Interestingly, around half of BC cases develop in women who have no identifiable risk factors other than being female and over 40 [2].
BC is classified based on the affected breast cells. Ductal carcinoma in situ (DCIS) is a non-invasive cancer with abnormal cells in the duct lining that haven’t spread. Invasive ductal carcinoma (IDC) is the most common type, where cancer cells spread beyond the ducts into other breast tissues. Invasive lobular carcinoma (ILC) begins in the lobules and spreads to surrounding tissues. Triple-negative BC lacks estrogen, progesterone, and human epidermal growth factor receptor 2 (HER2) receptors, making it harder to treat. HER2-positive BC is characterized by high levels of the HER2 protein, which promotes cancer cell growth, but can be treated with targeted therapies [3].
Genetic biomarkers like breast cancer gene 1/2 (BRCA1/2), phosphatidylinositol-4,5-bisphosphate 3-kinase catalytic subunit alpha (PIK3CA), GATA binding protein 3 (GATA3), tumor protein p53 (TP53), mitogen-activated protein kinase (MAPK) kinase kinase 1 (MAP3K1), partner and localizer of BRCA2 (PALB2), and BRCA1 interacting protein C-terminal helicase 1 (BRIP1) genes provide insights into the origins and treatment of BC [4]. Risk factors for BC include increasing age, family history, genetic mutations [particularly in BRCA1, BRCA2, and checkpoint kinase 2 (CHEK2)], exposure to female hormones, early menstruation, a previous BC diagnosis, and certain non-cancerous breast conditions. Lifestyle factors such as being overweight, insufficient physical activity, and alcohol consumption can also increase risk slightly. Differential gene expression (DGE) is crucial in cancer research as it helps identify genes uniquely expressed in cancer versus normal tissues, understand tumor biology, develop targeted therapies, predict treatment responses, and uncover mechanisms of drug resistance [4, 5].
While the basic ingredients in European diet and Asian diet remain the same they differ in terms of preparation with Asian cuisine focusing more on spices and aromatics. South Asians also tend to eat fewer meals per day and later in the evening than Europeans [6]. Asian populations have lower body mass index (BMI), but have higher total and central adiposity for a given body weight when compared with a matched white population. They also have 3 to 5 percent higher total body fat compared to European populations. Obesity is a major risk factor in BC and changes in obesity patterns may provide an insight into BC risks in the two populations [7].
Materials and methods
Workflow: Figure 1. Such as Figure 1 shows the workflow followed in the paper.
Retrieval of datasets and extraction of differentially expressed genes
The NCBI-Gene Expression Omnibus (GEO) [8] is a publicly accessible database that houses microarray data. It is extensively utilized for gene expression datasets and platform records. For this BC, we obtained gene expression datasets from NCBI-GEO and analyzed them using GEO2R online tool. Differentially expressed genes (DEGs) were identified using Benjamini & Hochberg’s false discovery rate (FDR), considering genes with p < 0.05 and logFC > 2.0 as significantly upregulated, data available in the Supplementary material.
Construction of protein-protein interaction network
We constructed protein-protein interaction (PPI) networks using STRING [9] and analyzed them in Cytoscape (v3.10.2) using Molecular Complex Detection (MCODE) and CytoHUBba plugins. We selected genes with at least three overlapping algorithms to identify highly upregulated biomarker (HUB) genes.
In total, 456 upregulated DEGs were extracted from the four datasets, and were utilized to construct the PPI network for the Asian population, and 2,950 upregulated DEGs were extracted from the three datasets, and were utilized to construct the PPI network for the European population.
Visualization and analysis of the network
Further analysis and visualization of the network were conducted using Cytoscape software (v3.10.2) [10]. Respective STRING networks were analyzed on Cytoscape using MCODE and CytoHUBba plugins. The networks were analyzed by MCODE and densely connected regions (clusters) within large PPI networks were identified. CytoHUBba was used to identify important nodes and subnetworks [11]. All 12 algorithms in cytoHUBba were used for analysis, namely: Degree Centrality, Betweenness Centrality, Closeness Centrality, Stress Centrality, Eccentricity, Radiality, BottleNeck, Edge Percolated Component (EPC), Maximum Neighborhood Component (MNC), Density of MNC (DMNC), Clustering Coefficient, and Maximal Clique Centrality (MCC).
Functional analysis
Gene set enrichment was performed using Enrichr-KG and GeneMANIA online bioinformatic tools to explore associations with known metabolic pathways, particularly cholesterol metabolism, adipocytokine signaling, and AMP-activated protein kinase (AMPK) regulation. Pathways with an adjusted p-value of less than 0.005 were considered [12, 13].
Results
GEO dataset processing to extract common DEGs
For BC, four GEO datasets with accession numbers GSE29044, GSE15852, GSE89116, and GSE61304 for the Asian population and three GEO datasets with accession numbers GSE42568, GSE21422, and GSE29431 for the European population were retrieved from the freely accessible NCBI-GEO database. GSE15852 https://www.ncbi.nlm.nih.gov/geo/query/acc.cgi?acc=GSE15852 (Malaysia), GSE29044 https://www.ncbi.nlm.nih.gov/geo/query/acc.cgi?acc=GSE29044 (Riyadh, Saudi Arabia), GSE89116 https://www.ncbi.nlm.nih.gov/geo/query/acc.cgi?acc=GSE89116 (New Delhi, India), and GSE61304 https://www.ncbi.nlm.nih.gov/geo/query/acc.cgi?acc=GSE61304 (Singapore) datasets were chosen for BC analysis of Asian population and GSE29431 https://www.ncbi.nlm.nih.gov/geo/query/acc.cgi?acc=GSE29431 (Barcelona, Spain), GSE21422 https://www.ncbi.nlm.nih.gov/geo/query/acc.cgi?acc=GSE21422 (Berlin, Germany), and GSE42568 https://www.ncbi.nlm.nih.gov/geo/query/acc.cgi?acc=GSE42568 (Dublin, Ireland) were chosen for the European population. Samples were taken from patients of different age groups and at different stages of cancer progression, DGE analysis results of these datasets provide HUB genes whose expression in BC could be dependent on environmental factors, diet, genetic history, and lifestyle disorders. Set of images in Figure 2a graphical representation of DEGs in Asian datasets, and set of images in Figure 2b graphical representation of DEGs in European datasets.
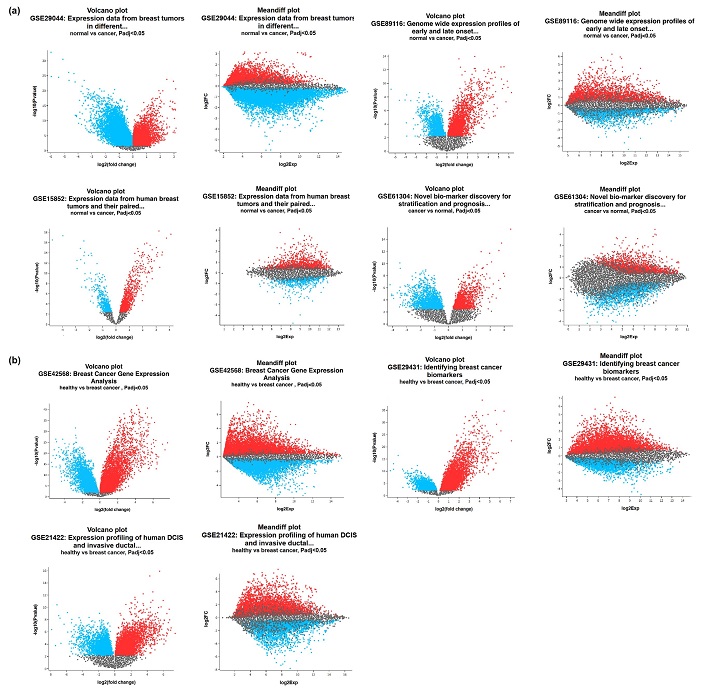
Graphical representation of DEGs. (a) Graphical representation of DEGs in Asian dataset; (b) graphical representation of DEGs in European dataset. DCIS: ductal carcinoma in situ; DEGs: differentially expressed genes; Exp: gene expression value; FC: fold change. Red points represent genes that are significantly upregulated in breast cancer. Blue points represent genes that are significantly upregulated in healthy tissue. Gray points represent genes that are not significantly different in expression between the two conditions
PPI network
In total, 2,950 upregulated DEGs were extracted from the three datasets, and were utilized to construct the PPI network for the European population and 456 upregulated DEGs were extracted from the four datasets, and were utilized to construct the PPI network for the Asian population. The PPI networks obtained from STRING [9] are shown in supplementary figures: Figures S1–7.
Common gene selection
The common genes were shortlisted using Bioinformatics & Evolutionary Genomics Venn diagram generator tool [14]. In selected BC datasets, we selected the genes which had minimum of 3 common algorithms in that particular dataset, for each (intra-dataset comparison), files are attached in the Supplementary material under CytoHUBba algorithms and CytoHUBba analysis. Followed by, every dataset’s selected gene list to check for common genes within them (inter-dataset comparison) to get HUB genes. The lists are mentioned in Table 1.
Selected gene list of all four Asian and European datasets
Asian | European | |||||
---|---|---|---|---|---|---|
Malaysia (GSE15852) | New Delhi (GSE89116) | Riyadh (GSE29044) | Singapore (GSE61304) | Dublin (GSE42568) | Barcelona (GSE29431) | Berlin (GSE21422) |
LEP (leptin) | PLIN1 (perilipin 1) | LEP | PPM2 | PPARG (peroxisome proliferator-activated receptor gamma) | PPARG | PPARG |
LPL (lipoprotein lipase) | IGF1 (insulin-like growth factor 1) | ALAS2 (5’-aminolevulinate synthase 2) | CDK1 (cyclin-dependent kinase 1) | EGFR (epidermal growth factor receptor) | IGF1 | LEP |
CD36 (cluster of differentiation 36) | PPARG | HBB (hemoglobin subunit beta) | AURKA (aurora kinase A) | LEP | CDH5 (cadherin-5) | CD34 |
GPD1 (glycerol-3-phosphate dehydrogenase 1) | CD36 | HBD (hemoglobin subunit delta) | NUF2 | CCL2 [chemokine (C-C motif) ligand 2] | LEP | IGF1 |
ACACB (acetyl-CoA carboxylase beta) | LEP | FCG3B (Fc gamma receptor IIIb) | EXO1 (exonuclease 1) | IGF1 | EGFR | VWF (von willebrand factor) |
PLIN1 | IL6 (interleukin 6) | PTG2 | TOP2A (DNA topoisomerase II alpha) | CAV1 (caveolin-1) | CD36 | FGF2 (fibroblast growth factor 2) |
PCK1 (phosphoenolpyruvate carboxykinase 1) | APOB (apolipoprotein B) | CD36 | COMP (cartilage oligomeric matrix protein) | APOB | FGF2 | CXCL12 (C-X-C motif chemokine ligand 12) |
CFD (complement factor D) | FGF2 | HBM (hemoglobin subunit mu) | COL11A1 (collagen type XI alpha 1 chain) | PTGS2 (prostaglandin-endoperoxide synthase 2) | APOB | LPL |
ANGTPL4 | ADIPOQ (adiponectin, C1Q and collagen domain containing) | SNCA (synuclein alpha) | FN1 (fibronectin 1) | TLR4 (toll-like receptor 4) | VWF | APOB |
RBP4 (retinol binding protein 4) | FABP4 (fatty acid-binding protein 4) | PPBP (pro-platelet basic protein) | FOXM1 (forkhead box M1) | CD36 | PPARA (peroxisome proliferator activated receptor alpha) | FABP4 |
CAV1 | CAV1 | KLF1 [Kruppel-like factor 1 (erythroid)] | CCNA2 (cyclin A2) | - | FOXO1 (forkhead box protein O1) | ADIPOQ |
TF (transferrin) | LIPE (hormone-sensitive lipase) | SLC25A37 (solute carrier family 25 member 37) | DLGAP5 (DLG associated protein 5) | - | FABP4 | FOXO1 |
ADH1B (alcohol dehydrogenase 1B) | KIT (KIT proto-oncogene, receptor tyrosine kinase) | OXTR (oxytocin receptor) | STAT1 (signal transducer and activator of transcription 1) | - | CAV1 | CAV1 |
- | PCK | ADRB2 (adrenoceptor beta 2) | MMP9 (matrix metallopeptidase 9) | - | FOS (Fos proto-oncogene, AP-1 transcription factor subunit) | CD36 |
- | PNPLA2 (patatin-like phospholipase domain containing 2) | - | TPX2 (TPX2 microtubule nucleation factor) | - | - | - |
- | - | - | ANLN (anillin actin binding protein) | - | - | - |
- | - | - | BIRC5 (baculoviral IAP repeat containing 5) | - | - | - |
-: no data
Cluster of differentiation 36 (CD36), leptin (LEP) were found to be common genes among GSE29044, GSE89116, and GSE15852 datasets and Singapore consisted of cyclin-dependent kinase 1 (CDK1), aurora kinase A (AURKA), DNA topoisomerase II alpha (TOP2A), and baculoviral IAP repeat containing 5 (BIRC5) (Asian). Caveolin-1 (CAV1), insulin-like growth factor 1 (IGF1), CD36, apolipoprotein B (APOB), peroxisome proliferator-activated receptor gamma (PPARG), LEP were found to be common genes among GSE42568, GSE21422 and GSE29431 datasets (European).
Venn diagram analysis
Venn diagram of GSE29044, GSE89116, GSE15852, and GSE61304 datasets (Asian population) and Venn diagram of GSE42568, GSE21422, and GSE29431 datasets (European population) is shown in Figure 3.
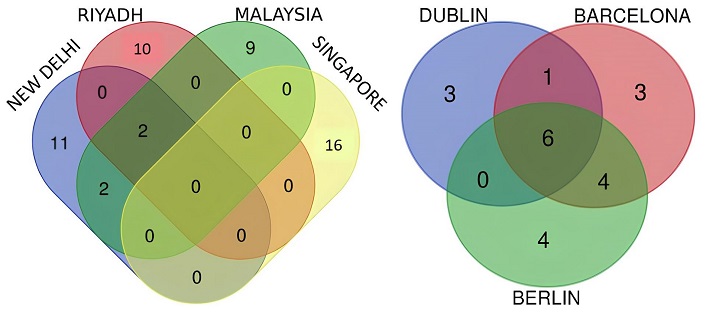
Venn diagram result of the HUB genes identified from each individual Asian and European datasets. HUB: highly upregulated biomarker
Table 2 contains tabulated data of Venn diagram of Asian and European Population. From Venn diagram Asian results we observe that there are no genes common between all four Asian datasets but CD36 and LEP were found as HUB genes between GSE29044, GSE89116, and GSE15852 datasets, and CAV1 and perilipin 1 (PLIN1) were found as secondary HUB genes common to GSE89116 and GSE15852. The Singapore data set consisting specifically of breast adenocarcinoma did not have any common HUB gene, with the other selected datasets as the other datasets were not inclusive only of breast adenocarcinoma. From Venn diagram European results we observe that CAV1, IGF1, CD36, APOB, PPARG, and LEP are common HUB genes found in European population.
Tabulated data of Venn diagram of Asian and European Population
Asian | European | |||
---|---|---|---|---|
Name | Genes | Names | Total | Elements |
Malaysia, New Delhi, Riyadh | CD36 (cluster of differentiation 36), LEP (leptin) | Barcelona, Berlin, and Dublin | 6 | CAV1 (caveolin-1), IGF1 (insulin-like growth factor 1), CD36, APOB (apolipoprotein B), PPARG (peroxisome proliferator-activated receptor gamma), LEP |
Malaysia, New Delhi | CAV1, PLIN1 (perilipin 1) | Barcelona, Dublin | 1 | EGFR (epidermal growth factor receptor) |
New Delhi | KIT (KIT proto-oncogene, receptor tyrosine kinase), FABP4 (fatty acid-binding protein 4), PPARG, PNPLA2 (patatin-like phospholipase domain containing 2), LIPE (hormone-sensitive lipase), PCK (phosphoenolpyruvate carboxykinase), IGF1, FGF2 (fibroblast growth factor 2), APOB, IL6 (interleukin 6), ADIPOQ (adiponectin, C1Q and collagen domain containing) | Barcelona, Berlin | 4 | FABP4, FGF2, VWF (von Willebrand factor), FOXO1 (forkhead box protein O1) |
Riyadh | ALAS2 (5’-aminolevulinate synthase 2), HBD (hemoglobin subunit delta), SLC25A37 (solute carrier family 25 member 37), HBM (hemoglobin subunit mu), FCG3B (Fc gamma receptor IIIb), KLF1 [Kruppel-like factor 1 (erythroid)], SNCA (synuclein alpha), PPBP (pro-platelet basic protein), OXTR (oxytocin receptor), ADRB2 (adrenoceptor beta 2) | Dublin | 3 | CCL2 [chemokine (C-C motif) ligand 2], TLR4 (toll-like receptor 4), PTGS2 (prostaglandin-endoperoxide synthase 2) |
Malaysia | TF (transferrin), PCK1, RBP4 (retinol binding protein 4), ACACB (acetyl-CoA carboxylase beta), ADH1B (alcohol dehydrogenase 1B), LPL (lipoprotein lipase), CFD (complement factor D), ANGPTL4 (angiopoietin like 4), GPD1 (glycerol-3-phosphate dehydrogenase 1) | Barcelona | 3 | PPARA (peroxisome proliferator activated receptor alpha), FOS (Fos proto-oncogene, AP-1 transcription factor subunit), CDH5 (cadherin-5) |
Singapore | RRM2 (ribonucleotide reductase regulatory subunit M2), CDK1 (cyclin-dependent kinase 1), AURKA (aurora kinase A), EXO1 (exonuclease 1), TOP2A (DNA topoisomerase II alpha), COMP (cartilage oligomeric matrix protein), COL11A1 (collagen type XI alpha 1 chain), FN1 (fibronectin 1), FOXM1 (forkhead box M1), CCNA2 (cyclin A2), DLGAP5 (DLG associated protein 5), STAT1 (signal transducer and activator of transcription 1), MMP9 (matrix metallopeptidase 9), TPX2 (TPX2 microtubule nucleation factor), ANLN (anillin actin binding protein), BIRC5 (baculoviral IAP repeat containing 5) | Berlin | 4 | LPL, CD34, CXCL12 (C-X-C motif chemokine ligand 12), ADIPOQ |
CD36 and LEP were found to be common HUB genes in both populations, while CAV1 and PLIN1 were prevalent in Asian datasets, and CAV1, IGF1, APOB, and PPARG were identified in European datasets (Table 1).
Functional enrichment analysis
Functional analysis by Enrichr-KG of Asian population
HUB genes common to GSE29044, GSE89116, and GSE15852 were found to be CD36 and LEP from the Venn diagram results. The CD36, LEP, CAV1, and PLIN1 genes were inputted in Enrichr-KG to obtain the following results shown in Figure 4. Table 3 represents gene table of Enrichr-KG pathways of Asian datasets.
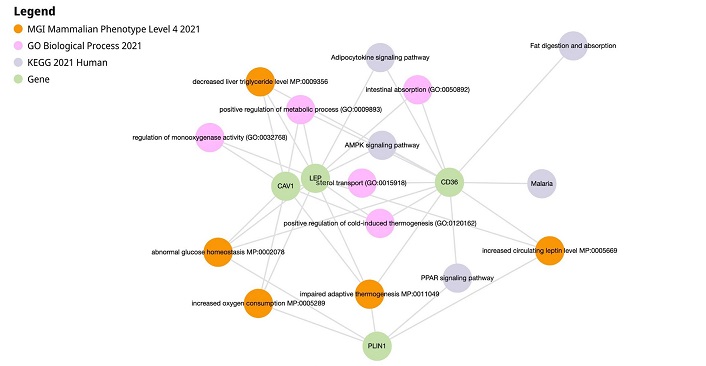
Enrichr-KG results of CD36, LEP, CAV1, and PLIN1. AMPK: AMP-activated protein kinase; CAV1: caveolin-1; CD36: cluster of differentiation 36; LEP: leptin; PLIN1: perilipin 1; PPAR: peroxisome proliferator-activated receptor
Enrichr-KG pathways and associated gene table (Asian)
Pathway | Associated genes | Role in breast cancer (BC) |
---|---|---|
Cholesterol metabolism | CD36 (cluster of differentiation 36), LEP (leptin) | Influences BC progression, aggressiveness, and drug resistance |
Impaired adaptive thermogenesis | CAV1 (caveolin-1), LEP, CD36, PLIN1 (perilipin 1) | Promote cancer cell survival and growth |
Adipocytokine signaling pathway | LEP, CD36 | Influence BC cell survival, growth, invasion, and metastasis |
Positive regulation of mitogen-activated protein kinase (MAPK) cascade | LEP, CD36 | Key in cell proliferation and death |
AMP-activated protein kinase (AMPK) signaling pathway | CD36, LEP | Functions as a tumor suppressor |
Increased oxygen consumption | CAV1, LEP, PLIN1 | Increase tumor proliferation |
Abnormal glucose homeostasis | CAV1, LEP, PLIN1, CD36 | Increases the proliferation of BC cells |
GeneMANIA results of CD36, LEP, CAV1, and PLIN1 are shown in Figure 5. LEP receptor (LEPR), hydroxysteroid 11-beta dehydrogenase 1 (HSD11B1), fatty acid-binding protein 4 (FABP4), PLIN1, PLIN2, PLIN4, PLIN5, abhydrolase domain containing 5 (also known as CGI-58) (ABHD5), scavenger receptor class B member 2 (SCARB2), histone deacetylase 6 (HDAC6), CAV2, low-density lipoprotein receptor-related protein 6 (LRP6), nitric oxide synthase 3 (NOS3), NOS trafficking (NOSTRIN), and potassium voltage-gated channel subfamily H member 2 (KCNH2) are associated genes that play roles in BC as biomarkers, inhibitors and promoters in Asian datasets based on GeneMANIA results.
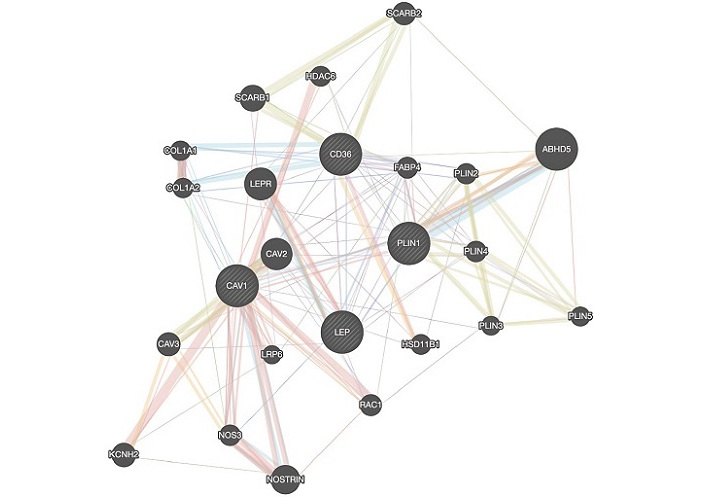
GeneMANIA results of CD36, LEP, CAV1, and PLIN1. ABHD5: abhydrolase domain containing 5; CAV1: caveolin-1; CD36: cluster of differentiation 36; COL1A1: collagen type I alpha 1 chain; FABP4: fatty acid-binding protein 4; HDAC6: histone deacetylase 6; HSD11B1: hydroxysteroid 11-beta dehydrogenase 1; KCNH2: potassium voltage-gated channel subfamily H member 2; LEP: leptin; LEPR: leptin receptor; LRP6: low-density lipoprotein receptor-related protein 6; NOS3: nitric oxide synthase 3; NOSTRIN: nitric oxide synthase trafficking; PLIN2: perilipin 2; RAC1: Rac family small GTPase 1; SCARB2: scavenger receptor class B member 2
Functional analysis by Enrichr-KG of European population
HUB genes common to GSE42568, GSE21422, and GSE29431 were found to be CAV1, IGF1, CD36, APOB, PPARG, and LEP from the Venn diagram results. The six genes were inputted in Enrichr-KG to obtain the following results shown in Figure 6. Table 4 represents gene table of Enrichr-KG pathways of European datasets.
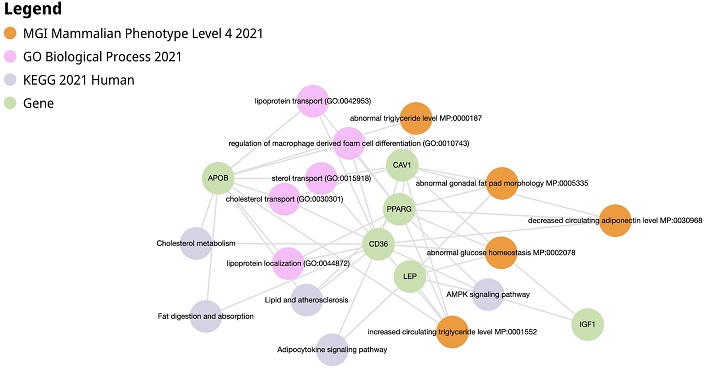
Enrichr-KG results of CAV1, IGF1, CD36, APOB, PPARG, and LEP. AMPK: AMP-activated protein kinase; APOB: apolipoprotein B; CAV1: caveolin-1; CD36: cluster of differentiation 36; IGF1: insulin-like growth factor 1; LEP: leptin; PPARG: peroxisome proliferator-activated receptor gamma
Enrichr-KG pathways and associated gene table (European)
Pathway | Associated genes | Role in breast cancer (BC) |
---|---|---|
Cholesterol metabolism | CD36 (cluster of differentiation 36), APOB (apolipoprotein B) | Influences BC progression, aggressiveness, and drug resistance |
Decreased circulating adiponectin level | CAV1 (caveolin-1), CD36, PPARG (peroxisome proliferator-activated receptor gamma) | Contribute to breast tumor development and progression |
Adipocytokine signaling pathway | LEP, CD36 | Influence BC cell survival, growth, invasion, and metastasis |
AMP-activated protein kinase (AMPK) signaling pathway | CD36, LEP, PPARG, IGF1 (insulin-like growth factor 1) | Functions as a tumor suppressor |
Increased circulating triglyceride level | CAV1, LEP, PPARG, APOB, CD36 | Influence cancer cell growth and survival |
Abnormal glucose homeostasis | CAV1, LEP, IGF1, CD36, PPARG | Increases the proliferation of BC cells |
GeneMANIA results of CAV1, IGF1, CD36, APOB, PPARG, and LEP are shown in Figure 7. HSD11B1, CAV2, FABP4, IGF binding protein 2 (IGFBP2), APOB, aquaporin 7 (AQP7), PLIN4, APOA1, PLIN1, cell death inducing DFFA like effector a (CIDEA), glycerol-3-phosphate dehydrogenase 1 (GPD1), hormone-sensitive lipase (LIPE), adiponectin, C1Q and collagen domain containing (ADIPOQ), early B-cell factor 1 (EBF1), CIDEC, EBF3, palmdelphin (PALMD), semaphorin 3G (SEMA3G), IGFBP6, oxidized low-density lipoprotein receptor 1 (OLR1), solute carrier family 19 member 3 (SLC19A3) are associated genes that play roles in BC based on GeneMANIA results for European datasets.
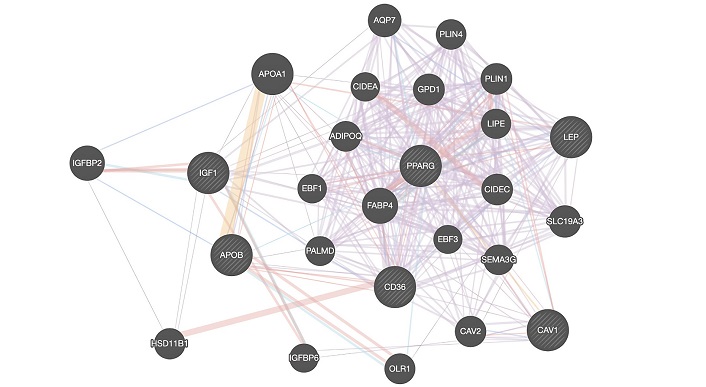
GeneMANIA results of CAV1, IGF1, CD36, APOB, PPARG, and LEP. ADIPOQ: adiponectin, C1Q and collagen domain containing; APOA1: apolipoprotein A1; AQP7: aquaporin 7; CAV2: caveolin-2; CD36: cluster of differentiation 36; CIDEA: cell death inducing DFFA like effector a; EBF1: early B-cell factor 1; FABP4: fatty acid-binding protein 4; GPD: glycerol-3-phosphate dehydrogenase; HSD11B1: hydroxysteroid 11-beta dehydrogenase 1; IGF1: insulin-like growth factor 1; IGFBP2: insulin-like growth factor binding protein 2; LEP: leptin; LIPE: hormone-sensitive lipase; OLR1: oxidized low-density lipoprotein receptor 1; PALMD: palmdelphin; PLIN4: perilipin 4; PPARG: peroxisome proliferator-activated receptor gamma; SEMA3G: semaphorin 3G; SLC19A3: solute carrier family 19 member 3
Comparison of gene expression across all seven datasets
A collective HUB gene analysis across all datasets reinforced the significance of CD36, LEP, and PPARG as key molecular regulators in BC progression.
Discussion
Comparing DEGs between European and Asian BC datasets provides crucial insights into population-specific molecular mechanisms and pathways. These comparisons highlight genetic diversity and reveal unique tumor biology shaped by distinct genetic backgrounds and environmental influences. Universal biomarkers such as CD36 and LEP emerge as common elements across populations, while region-specific genes like CAV1 and PLIN1 in Asian cohorts or IGF1 and APOB in European cohorts underline molecular differences that can inform tailored diagnostic and therapeutic strategies. In volcano plot and mean difference plot: red points represent genes that are significantly upregulated in BC compared to healthy tissue. Blue points represent genes that are significantly downregulated in BC compared to healthy tissue. Gray points represent genes that are not significantly different in expression between the two conditions. In Asian datasets: GSE29044: a larger number of blue points in comparison to red points indicates that the number of downregulated genes is higher than that of upregulated genes. GSE89116: a larger number of red points in comparison to blue points indicates that the number of upregulated genes is higher than that of downregulated genes. GSE15852: An almost equal number of blue and red points are observed indicating that the number of upregulated and downregulated genes are around the same. GSE61304: the number of grey points is higher than that of both blue and red points indicating that these genes are not different in expression to that of healthy tissues and downregulated and upregulated genes are around the same number. In European datasets: GSE21422, GSE29431, and GSE42568 a larger number of red points were observed in comparison to blue points indicating that the number of upregulated genes is higher than that of downregulated genes. This comparative approach enhances our understanding of BC heterogeneity and supports the advancement of personalized medicine.
Lifestyle and environmental factors, such as dietary habits and adiposity patterns, significantly influence key pathways like cholesterol metabolism and adipocytokine signaling [15]. Aberrant cholesterol metabolism can trigger carcinogenic pathways, such as the Hedgehog signaling system, which aids in tumor growth and the survival of cancer stem cells [16]. The development of BC is significantly influenced by adipocytokine signaling, especially when obesity is present. While lower levels of protective adipokines like adiponectin may raise the risk of developing cancer, higher levels of specific adipocytokines, such as LEP, can encourage tumor growth, invasion, and metastasis [17]. Dysregulated fatty acid metabolism sustains BC cell proliferation and survival by providing essential bioenergetic and biosynthetic resources. Enhanced lipogenesis, fatty acid uptake, and altered β-oxidation support membrane synthesis, energy production, and redox balance. Additionally, lipid signaling influences oncogenic pathways, promoting cell cycle progression, apoptosis resistance, and metastasis [18].
The development of BC is significantly influenced by adipocytokine signaling, especially when obesity is present. While lower levels of protective adipokines like adiponectin may raise the risk of developing cancer, higher levels of specific adipocytokines, such as LEP, can encourage tumor growth, invasion, and metastasis. Asian populations exhibit higher central adiposity despite lower BMI, while European cohorts show different lipid profiles influenced by dietary fat consumption [19]. These differences impact DEGs and associated pathways, emphasizing the need for region-specific prevention and treatment strategies. By bridging knowledge gaps in global BC research, this study ensures inclusivity for underrepresented populations and contributes to identifying robust biomarkers for precision diagnostics and targeted therapies.
Gene ontology
Gene ontology for Asian populations
The datasets GSE29044 (Riyadh), GSE15852 (Malaysia), GSE89116 (New Delhi), and GSE61304 (Singapore) consist of breast carcinoma and healthy tissue samples. HUB genes identified include CAV1, CD36, LEP, and PLIN1, with additional key proteins such as LEPR, PTPN1, SOC3, HSD11B1, CEBPA, PTK2, FABP4, integrin alpha-6 (ITGA6), clusterin (CLU), cartilage oligomeric matrix protein (COMP), ghrelin and obestatin prepropeptide (GHRL), PPARG, and SCARB2. These genes play significant roles as potential biomarkers and therapeutic targets.
ABHD5 suppresses cancer cell proliferation via the ABHD5/ATGL pathway, while KCNH2 promotes epithelial-mesenchymal transition (EMT), facilitating metastasis [20]. Elevated SCARB2 levels are linked to advanced cancer stages and poor prognosis, and overexpression of HDAC6 enhances metastasis through heat shock factor 1 (HSF1) activation [21]. CAV1 regulates critical signaling pathways, including estrogen receptor (ER), epidermal growth factor receptor (EGFR), and transforming growth factor beta (TGF-β), while LRP6 is a biomarker for poor prognosis via Wnt signaling pathway/β-catenin activation [22]. LEP and its receptor LEPR drive proliferation and angiogenesis through janus kinase 2/signal transducer and activator of transcription 3 (JAK2/STAT3) and Extracellular signal-Regulated Kinase (ERK) pathways [23]. Suppressor of cytokine signaling 2 (SOCS2) and SOCS3 regulate STAT pathways, influencing proliferation and angiogenesis [24]. FABP4 and ITGA6 enhance invasive properties and establish a link between obesity and cancer risk [25]. COMP, identified in the Singapore cohort, is associated with cancer stem cell properties [26]. These findings underscore the roles of lipid metabolism, signaling pathways, and the tumor microenvironment in BC progression, with CD36, LEP, and PPARG highlighted as key therapeutic targets.
Gene ontology for European populations
The datasets GSE42568 (Dublin), GSE21422 (Berlin), and GSE29431 (Barcelona) include breast carcinoma and healthy tissue samples. HUB genes identified include CAV1, IGF1, CD36, APOB, PPARG, and LEP. Additional genes such as IGFBP2, APOB, HSD11B1, AQP7, APOA1, GPD1, ADIPOQ, PPARG, EBF3, PALMD, SEMA3G, IGFBP6, OLR1, and SLC19A3 serve as biomarkers, inhibitors, or promoters in BC. IGFBP2 promotes tumor proliferation, migration, and angiogenesis, while APOB mutations are linked to aggressive BC, especially in postmenopausal women [27]. HSD11B1 induces EMT, enhancing metastasis, and AQP7 correlates with better survival [28]. APOA1 suppresses apoptosis and supports tumor growth [29], and GPD1 inhibits proliferation via the phosphatidylinositol 3-kinase/protein kinase B (PI3K/AKT) pathway [30]. ADIPOQ polymorphisms influence serum adiponectin levels and BC risk [31]. PPARG regulates angiogenesis and apoptosis in ER + BC and is a potential target for natural treatments like quercetin [32]. EBF3 induces cell cycle arrest, and PALMD inhibits tumor growth by blocking the PI3K/AKT pathway [33]. SEMA3G promotes angiogenesis and metastasis, while IGFBP6 downregulation increases metastasis risk [34]. OLR1 upregulation indicates poor prognosis due to immune evasion [35]. LIPE promotes lipolysis, providing metabolic substrates for tumor growth.
Common gene ontology across populations
CD36 and LEP were common HUB genes identified in both European and Asian datasets, with FABP4, HSD11B1, and PLIN family genes emerging as associated genes. CD36 enhances fatty acid uptake, lipid metabolism, cancer proliferation, and EMT, marking it as a potential cancer stem cell marker [36]. LEP activates pathways like MAPK, PI3K/AKT, and JAK2/STAT, driving proliferation, migration, and angiogenesis across BC subtypes [5]. PLIN1 regulates lipid droplets in aggressive tumors, while FABP4 links obesity to BC by promoting lipolysis and inflammation [37]. HSD11B1 induces EMT, facilitating metastasis. Upregulated genes such as LIPE, AQP7, CD36, and PLIN1 in epithelial and stromal compartments highlight enhanced fatty acid metabolism and transport, supporting tumor growth [28].
Pathways and gene function in BC
Asian populations
CD36-mediated signaling pathways involving Src-family kinases, MAPKs, and the ERK-1/2 pathway regulate cell proliferation and survival in BC [38]. Elevated MAPK activity is observed in approximately half of breast tumors [39]. LEP, CD36, and CAV1 contribute to thermogenesis, fostering a pro-tumor immune microenvironment by reducing cytotoxic T-cell activity and increasing immunosuppressive cells. Enhanced oxygen consumption by LEP, CAV1, and PLIN1 creates hypoxic conditions that promote tumor progression and therapy resistance [40].
European populations
CD36 and APOB drive oncogenic activity through scavenger receptors like Scavenger Receptor Class B Type 1 (SR-BI), promoting tumor proliferation and migration via MAPK and PI3K pathways [36]. IGF1, CD36, CAV1, and APOB regulate hemostatic pathways, contributing to tumor progression by enhancing coagulation and angiogenesis. Toll-like receptor (TLR) signaling, influenced by CD36 and APOB, promotes chronic inflammation and chemoresistance [41]. LEP, PPARG, and CD36 modulate white adipocyte differentiation, creating cancer-associated adipocytes (CAAs) that support tumor aggressiveness.
Common pathways across populations
CD36 and LEP regulate cholesterol metabolism, influencing fatty acid uptake, lipid storage, and inflammation. LEP drives tumor proliferation and migration via MAPK and JAK2/STAT pathways, while CD36 interacts with AMPK to suppress tumorigenic metabolism and induce cell-cycle arrest. AMPK plays a pivotal role in regulating metabolic pathways essential for tumorigenesis and cancer progression. It influences key cellular processes that govern cancer cell growth, survival, and proliferation while also modulating pathways involved in glucose, lipid, and protein metabolism [42]. Enhanced lipid metabolism, driven by APOB, FABP4, and PPARG, supports tumor growth and immune evasion [43]. Elevated triglyceride levels and dysregulated lipid metabolism are crucial for tumor proliferation, migration, and resistance to therapy [44].
AMPK and PPAR pathways, activated by adiponectin, provide metabolic regulation, while salt-inducible kinase 2 (SIK2) suppresses tumor progression by inhibiting the PI3K/AKT and Ras/ERK pathways [45]. Collectively, these pathways underscore the metabolic reprogramming central to BC progression.
Future directions
This study highlights CD36 and LEP as promising therapeutic targets, warranting further wet lab validation and clinical trials. Additionally, integrating multi-omics approaches and AI-driven predictive models could refine precision oncology frameworks.
Conclusion
BC exhibits significant molecular heterogeneity influenced by genetic, dietary, environmental, and epigenetic factors. This study identified CD36 and LEP as universal HUB genes consistently upregulated across both Asian and European BC datasets, indicating their potential as global diagnostic and prognostic biomarkers. Additionally, CAV1, IGF1, APOB, and PPARG were identified as key regulatory genes in European populations, while PLIN1 and CAV1 were prominent in Asian populations, suggesting region-specific molecular variations that may influence tumor progression and therapeutic response.
Functional enrichment analysis highlighted the involvement of these genes in critical pathways, including cholesterol metabolism, adipocytokine signaling, AMPK regulation, and lipid metabolism, emphasizing their role in BC pathophysiology. The observed differences in gene expression patterns underscore the necessity for personalized medicine approaches, incorporating molecular profiling to optimize early detection, risk stratification, and targeted treatment strategies.
Clinically, CD36 and LEP may serve as potential biomarkers for non-invasive liquid biopsy screening, enabling early detection and tumor stratification. Their role in metabolic regulation also suggests potential therapeutic applications, with CD36 inhibitors and LEP antagonists warranting further exploration in obesity-associated BC. Furthermore, PPARG agonists and lipid metabolism modulators may serve as promising candidates for targeted interventions, particularly in hormone receptor-positive BC subtypes. Given the ethnic and regional variations in HUB gene expression, incorporating population-specific molecular profiling into treatment regimens could enhance therapeutic efficacy and minimize adverse effects.
Future research should focus on large-scale cohort validation and experimental studies to confirm the clinical utility of these biomarkers and evaluate their therapeutic potential. Additionally, the integration of multi-omics approaches, AI-driven predictive models, and metabolic interventions could refine precision oncology strategies, ensuring more effective, patient-centered treatment paradigms. CD36 and LEP emerge as promising targets for advancing BC diagnostics, prognostics, and therapeutics, paving the way for more precise and individualized management strategies in breast oncology.
Abbreviations
ABHD5: | abhydrolase domain containing 5 |
ADIPOQ: | adiponectin, C1Q and collagen domain containing |
AMPK: | AMP-activated protein kinase |
APOB: | apolipoprotein B |
AQP7: | aquaporin 7 |
BC: | breast cancer |
BMI: | body mass index |
BRCA1/2: | breast cancer gene 1/2 |
CAV1: | caveolin-1 |
CD36: | cluster of differentiation 36 |
COMP: | cartilage oligomeric matrix protein |
DEGs: | differentially expressed genes |
DGE: | differential gene expression |
EBF1: | early B-cell factor 1 |
EMT: | epithelial-mesenchymal transition |
ER: | estrogen receptor |
ERK: | Extracellular signal-Regulated Kinase |
FABP4: | fatty acid-binding protein 4 |
GEO: | Gene Expression Omnibus |
GPD1: | glycerol-3-phosphate dehydrogenase 1 |
HDAC6: | histone deacetylase 6 |
HER2: | human epidermal growth factor receptor 2 |
HSD11B1: | hydroxysteroid 11-beta dehydrogenase 1 |
HUB: | highly upregulated biomarker |
IGF1: | insulin-like growth factor 1 |
IGFBP2: | insulin-like growth factor binding protein 2 |
ITGA6: | integrin alpha-6 |
JAK2: | janus kinase 2 |
KCNH2: | potassium voltage-gated channel subfamily H member 2 |
LEP: | leptin |
LEPR: | leptin receptor |
LIPE: | hormone-sensitive lipase |
LRP6: | low-density lipoprotein receptor-related protein 6 |
MAPK: | mitogen-activated protein kinase |
MCODE: | Molecular Complex Detection |
MNC: | Maximum Neighborhood Component |
OLR1: | oxidized low-density lipoprotein receptor 1 |
PALMD: | palmdelphin |
PI3K/AKT: | phosphatidylinositol 3-kinase/protein kinase B |
PLIN1: | perilipin 1 |
PPARG: | peroxisome proliferator-activated receptor gamma |
PPI: | protein-protein interaction |
SCARB2: | scavenger receptor class B member 2 |
SEMA3G: | semaphorin 3G |
SLC19A3: | solute carrier family 19 member 3 |
SOCS2: | suppressor of cytokine signaling 2 |
STAT1: | signal transducer and activator of transcription 1 |
Supplementary materials
The supplementary figures for this article are available at: https://www.explorationpub.com/uploads/Article/file/1001320_sup_1.pdf. Other supplementary material for this article is available at: https://www.explorationpub.com/uploads/Article/file/1001320_sup_2.xlsx.
Declarations
Author contributions
PM: Project administration, Conceptualization, Validation, Supervision, Writing—review & editing. SS: Conceptualization, Validation, Data curation, Methodology, Writing—review & editing. SR: Data curation, Methodology, Writing—original draft.
Conflicts of interest
The author declares that there are no conflicts of interest.
Ethical approval
Not applicable.
Consent to participate
Not applicable.
Consent to publication
Not applicable.
Availability of data and materials
All datasets (generated/analyzed) for this study are included in the manuscript and the supplementary files.
Funding
Not applicable.
Copyright
© The Author(s) 2025.
Publisher’s note
Open Exploration maintains a neutral stance on jurisdictional claims in published institutional affiliations and maps. All opinions expressed in this article are the personal views of the author(s) and do not represent the stance of the editorial team or the publisher.