Abstract
The cardiovascular system remains vulnerable to a multitude of threats, including infections, inflammation, and oxidative stress. These factors contribute significantly to the development and progression of cardiovascular diseases, a pressing global health concern. Historically, research into natural medicines has primarily focused on isolated compounds. However, complex natural entities like essential oils, rich in diverse chemical constituents, offer superior therapeutic potential. We discuss here how their inherent variability can lead to unpredictable synergistic and antagonistic effects, hindering consistent therapeutic outcomes. This inconsistency is particularly problematic in addressing the rising incidence of perioperative infections and the need to combat radical-mediated damage and subclinical inflammation. This scoping review departs from the hypothesis “Can we leverage complex natural products for the discovery of new clinical approaches to cardiovascular diseases encompassing inflammation and infection?”. Therefore, we delve into the potential of complex natural products, particularly essential oils, in preventing cardiovascular infections and mitigating oxidative damage, emphasizing the crucial role of artificial neural networks (ANNs) in advancing this field. The review emphasizes the need for multidisciplinary collaboration to generate quality data for effective ANN analysis, envisioning future integration of omics technologies with ANNs for more precise predictions of natural product activities. It addresses challenges in translating AI-designed essential oils to clinical practice, including intellectual property protection and standardization issues due to regional variability, suggesting a potential role for the World Health Organization in establishing guidelines for essential oil specifications to ensure consistent efficacy while enabling global accessibility. The author concludes by stressing the need to address ethical considerations at the intersection of technology, science, and clinical practice, particularly regarding the proprietary status of essential oils versus making them freely available worldwide.
Keywords
Cardiovascular diseases, natural products, essential oils, artificial intelligence, antimicrobials, antioxidants, computational chemistryIntroduction
Heart and circulatory disease, also known as cardiovascular disease (CVD), is a major cause of death and disability. The World Health Organization (WHO) predicts that 22.2 million people will die from CVD in 2030 [1]. In the UK, it affects around seven million people and is considered the single biggest condition where lives can be saved by the National Health System (NHS) over the next 10 years [2].
The high-risk factors in cardiovascular health include infections, inflammation, and oxidative stress. These elements can significantly contribute to the development and progression of CVD, posing significant challenges to global health. Infection and chronic inflammatory diseases, such as rheumatoid arthritis, systemic lupus erythematosus, and psoriasis are associated with an increased risk of CVD: they induce the acute-phase response, leading to multiple alterations in lipid and lipoprotein metabolism thus leading to atherogenesis [3]. Several infectious agents such as Staphylococcus aureus, Escherichia coli, and Candida albicans are responsible for the most common life-threatening infections in the cardiovascular system. One such is endocarditis, one of the fourth most common life-threatening infections together with sepsis, pneumonia, and intraabdominal abscess, with S. aureus being the most frequently identified pathogen [4]. While this shift is partially attributable to an increased rate of i.v. drug abuse but healthcare-associated and nosocomial infections have become increasingly common, representing about 30% of all endocarditis cases [5], such as dental invasive procedures [6] or heart implants and transplants [7, 8]. Many even argue that oral bacteria-related infective endocarditis is likely to result from daily activities (e.g., tooth brushing, flossing, and chewing), particularly in those with poor oral hygiene [6]. Infective endocarditis caused by E. coli is a relatively rare condition, but its incidence is on the rise, particularly among older women. Moreover, it carries a higher mortality rate compared to endocarditis caused by the Haemophilus-Aggregatibacter-Acardiobacterium-Eikenella (HACEK) group bacteria [9]. E. coli is also relevant in the epidemiology of bloodstream infections caused by hematopoietic stem cell transplantation [10]. Research also suggests a strong link between infections, particularly bacteraemia and sepsis, and the development of endothelial dysfunction, which can lead to CVD-like atherosclerosis caused by a range of microorganisms, such as E. coli and C. albicans [11]. The latter is mostly associated with naturally- or chemically- immunoexpressed patients in the aftermath of transplants and cardiac prosthetic surgery [12]. All three above-mentioned bacteria were also found associated with ventilator-associated pneumonia, which is the most common and serious nosocomial infection that threatens patients who have undergone cardiac surgery [13].
The interplay between infections and oxidative stress is a complex one. Infections can trigger oxidative stress, as the immune system generates ROS to combat invading pathogens. Conversely, oxidative stress can weaken the immune system, making individuals more susceptible to infections [14, 15]. This vicious cycle can perpetuate CVD and make it difficult to treat. The delicate balance between pro- and anti-inflammatory mediators can be disrupted, leading to an increase in oxidative stress [16]. This oxidative stress can directly target lipids, particularly low-density lipoprotein (LDL). Oxidized LDL, in turn, becomes a potent trigger for inflammation, further exacerbating the cycle. Moreover, inflammation can impair the function of high-density lipoprotein (HDL), a protective factor that helps remove excess cholesterol from the arteries. This “assault” on both LDL and HDL can contribute to the formation of atherosclerotic plaques, ultimately increasing the risk of heart disease and stroke. Experiments in hamsters and mice have shown that blocking the production of leukotrienes or using antioxidants can effectively reduce the adhesion of white blood cells to blood vessel walls caused by oxidized low-density lipoprotein (oxLDL) [17]. Thus, understanding the intricate connections between inflammation, infection, and lipid oxidation (illustrated in Figure 1) is crucial for developing effective strategies to prevent and manage CVD [18].
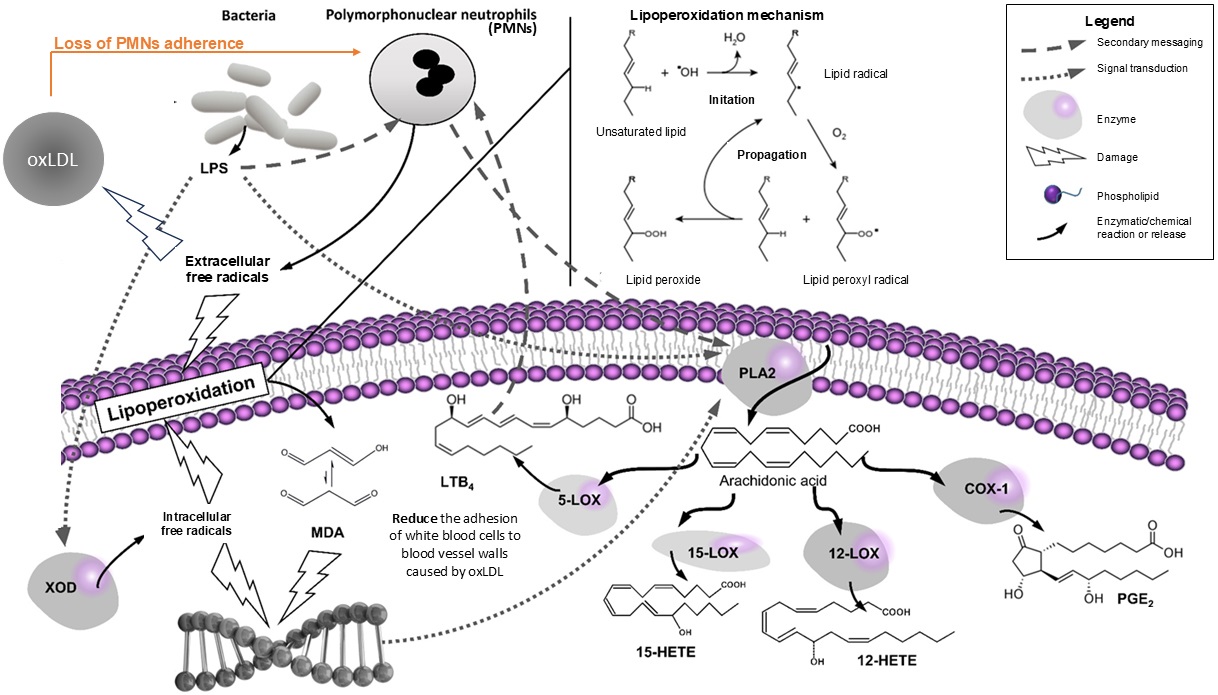
Interplay of inflammation, infection, and lipid oxidation in CVD. LOX: lipoxygenase; LPS: lipopolysaccharide; oxLDL: oxidized low-density lipoprotein; XOD: xanthine oxidase; MDA: malondialdehyde; LTB4: leukotriene B4; PGE2: prostaglandin E2; ROS: radical oxygen species
In terms of therapeutic interventions, antibiotic therapy can be used to treat infections, while antioxidant supplements and medications can help combat oxidative stress and indirectly stop the vicious loop of chronic or sub-clinic inflammation illustrated in Figure 1. However, the increased resistance to existing antibiotics [19] and the mixed results of the use of antioxidant supplements [20] calls for further research to determine the optimal use of these interventions.
Methodology
This is a scoping review to test the hypothesis “Can we leverage complex natural products (NPs) for the discovery of new clinical approaches to CVD diseases encompassing inflammation and infection?”. The search in PubMed and Google Scholar was run with the keywords [“cardiovascular” AND (“Natural Product” OR “ESSENTIAL OIL”)]. Only articles that met the requirement to address the pros- and cons- of the hypothesis were retained.
Natural products in CVD
The NPs in modern medicine and drug discovery is indispensable. They keep being a source for lead compounds, with approximately one-third of the current drug armamentarium directly or indirectly originating from nature [21]. Therefore, they may well be the source of new approaches to address the challenges posed by infections and oxidative stress in CVD. In fact, a recent review highlighted that modern cardiovascular therapies include NPs sourced from a variety of origins, including 53 from plants, 33 from animals, and 17 from microorganisms. Importantly, they found that over 20 molecules isolated from or based on natural sources are in current development. Plants remain the primary source of NPs, primarily targeting conditions like hypertension, heart failure, heart failure with myocardial hypertrophy, thrombosis, and arrhythmias. These NPs encompass diverse structures, such as alkaloids, cardiac glycosides, mucopolysaccharide sulphates, flavonoids, esters, lactones, and others. Among these, alkaloids and cardiac glycosides are the most prevalent [22].
But the examples above are what we call “single chemical entities” (i.e. single molecules). In this paper, we are going to focus on the potential of “complex chemical entities” (i.e. extracts or fractions from NPS containing dozens of components). Complex NPs have the advantage of a multi-targeted action, thus potentially overcoming resistance from infectious agents for longer than isolated molecules [23]. Components of complex NPs often exhibit synergistic, antagonistic, or additive effects, as extensively reviewed [24, 25], and in particular here is good evidence for synergies playing a big role on their antimicrobial [26] and antioxidant [27] activities.
There are already antecedents of the clinical use of complex NPs even for critical conditions despite the challenges in unravelling their full chemical complexity and the difficulty of assigning their pharmacological effects to a single compound. A case in point is how the U.S. Food and Drug Administration (FDA) opened their use as long as the composition can be defined to a certain extend and its efficacy can be ascertained in clinical trials [28]. Two examples of botanical drugs approved by the FDA are sinocatechins—a concentrated extract of green tea standardised in catechins [29] and crofelemer—a fraction of the sap of the Dragon’s blood tree (Croton leichlerii) standardised in condensed tannins [30] for the treatment of genital warts and acquired immunodeficiency syndrome (AIDS)-associated non-infectious diarrhoea, respectively.
This paper wants to focus on volatile NPs fractions [commonly known as “essential oils (EOs)”] which (despite its recognised therapeutic potential) is an underrepresented class in the CVD armamentarium so far [31]. For this we build up our pioneering work on the application of artificial intelligence (AI) in the modelling of their antioxidant [32] and antimicrobial [33, 34] activities. EOs present unique analytical, pharmacokinetic, and pharmacological advantages: their composition can be ascertained up to 90–99% by exploiting gas-chromatography mass-spectrometry (GC-MS) [35] contrarily with other types of extracts, they readily pass biological membranes as wells as being endowed with powerful bioactivities [36].
Why do complex NPs need to leverage AI to become a clinical option?
The evaluation of the antimicrobial activity of NPs is complicated by the fact that their activity often cannot be attributed to a single compound. Instead, the overall effect may result from complex interactions between multiple components, including synergistic and antagonistic effects [37]. This complexity makes it challenging to fully understand and predict the antimicrobial activity of EOs through traditional laboratory experiments.
In fact, EOs exert their effects through a combination of specific and non-specific mechanisms that are not fully understood [38]. The antimicrobial activity of EOs is influenced by a complex interplay of chemical properties and mechanisms of action. Different monoterpenes, the primary components of many EOs, can penetrate cell membranes at varying rates, disrupting their structure and function and compromising their ability to maintain ion gradients [39]. It might be expected that Gram-positive bacteria, with their simpler cell wall structure, would be more susceptible to EOs than Gram-negative bacteria. However, membrane permeability is just one factor, and the specific mode of action can vary across different microorganisms. For example, tea tree oil is known to increase membrane permeability thus inducing ion leakage in E. coli and S. aureus but also inhibits microbial respiration [40].
Studies over the past 20 years have also demonstrated the potential of EOs and their compounds to combat CVD risk factors like hypertension, dyslipidemia, and diabetes, primarily through direct effects, modulation of related targets, and general cellular protection. Monoterpenes are prominent in hypotensive and anti-dyslipidemic/antidiabetic effects, while phenylpropanoids excel in anti-platelet activity. However, clinical trials are limited, and factors like volatility and chemical variability necessitate careful consideration for effective clinical application [41, 42]. Despite the medical community’s critical stance towards complementary therapies, there is often preliminary evidence suggesting the beneficial effects of aromatherapy in CVD patients [43, 44], thus fuelling ongoing debates questioning their true efficacy [45, 46] without reaching a clinical consensus.
AI offers a promising approach to modeling such complex, poorly characterized processes. By focusing on the input and output, artificial neural networks (ANNs) can effectively capture the relationship between EOs and their antimicrobial activity, without requiring a detailed understanding of the underlying mechanisms [47, 48]. Moreover, it has been demonstrated that they can also link pharmacokinetic and pharmacodynamic profiles of NPs with multiple cardioprotective mechanisms, as well as providing a simple method for identifying and ranking the relative contribution to the multiple therapeutic effects of the natural drugs [49].
AI has been shaping our lives for a while before the public became fully aware of its existence with the introduction of chatbots and virtual assistants. They are based on ANNs which have been also applied in many disparate areas of medical science including the prediction of bioactivities in toxicology and pharmacology [50], microbiology [51], or the optimization of drug design [47].
The concept of predicting the bioactivity of any NP based on its unique chemical composition gained traction among scientists in the late 20th century, but systematic exploration was limited due to the experimental challenges of characterizing the intricate interactions between numerous components [37]. Machine learning offers significant advantages in predicting NP bioactivity based on their chemical composition. Unlike traditional statistical methods, machine learning models, particularly ANNs, can effectively detect complex non-linear relationships between variables, identify all possible interactions without relying on complex equations, and operate with less stringent statistical expertise. However, ANNs also have limitations. Their “black box” nature, where the internal mechanisms of the process remain largely unknown, necessitates large training datasets to accurately estimate the numerous weights within the model.
The applications of ANNs in this field encompass classification (pattern recognition), prediction, and modelling [52]. Therefore, the use of ANNs may overcome these difficulties thus becoming a convenient computational tool allowing the pharmaceutical industry to select herbal extracts or EOs with optimal chemical activities (such as antioxidant), antimicrobial properties, and/or pharmacological activities (such as anti-inflammatory properties, absorption, metabolism, distribution, and excretion) [50, 53]. This is not trivial, as NPs are notoriously complex in terms of chemical composition, which may significantly vary depending on the batch and the supplier. This variability implies a constant use of laboratory analysis. ANNs able to model and predict such properties would result in savings and enhanced consistency of the final product. The use of such computational models holds the potential to overcome (and take into account) all the possible (bio) chemical interactions, synergisms, and antagonisms between the numerous components of active natural ingredients.
The use of modern metabolomics analytical technologies to unravel the full complexity of NPs [liquid-chromatography mass-spectrometry (LC-MS), GC-MS], high-throughput screenings (HTS) to ascertain their experimental bioactivities and the use of ANNs to model how different compositions lead to different results seems to be the rational way forward to accelerate drug discovery as illustrated by Figure 2.
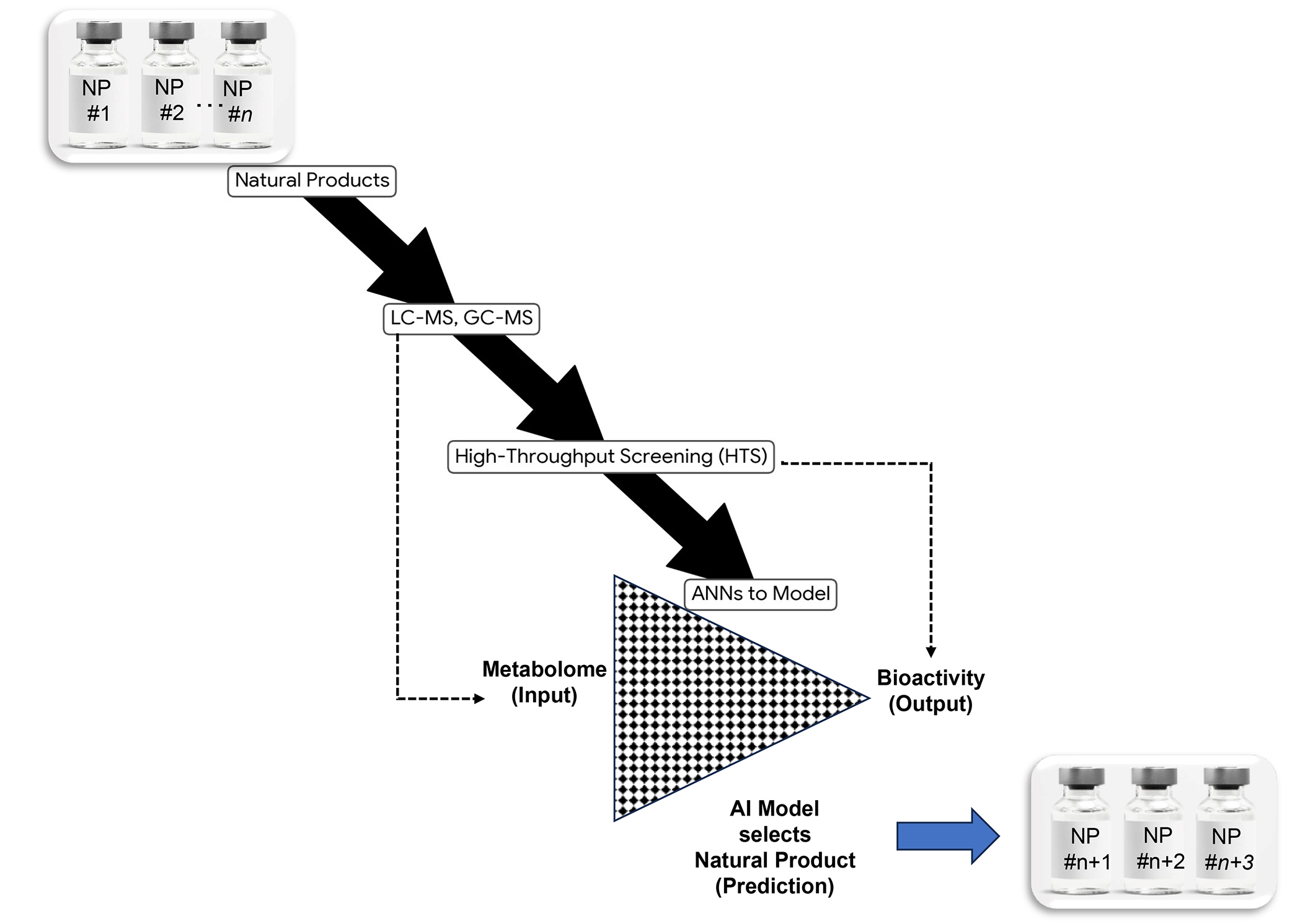
A modern workflow to ensure consistent pharmacological efficacy of complex natural products (= complex chemical entities) considering synergies, antagonisms and additivities between all compounds (AI assisted bio standardisation). NP: natural product; AI: artificial intelligence; ANNs: artificial neural networks; LC-MS: liquid-chromatography mass-spectrometry; GC-MS: gas-chromatography mass-spectrometry
Basic concepts in ANNs
To enhance accessibility for non-specialist readers, this section provides a simplified overview of the core concepts underlying ANNs. For a more in-depth exploration of these topics, we encourage readers to consult the insightful works of Krogh [54], Dohnal et al. [47], and Zupan and Gasteiger [55]. These are listed in order of increasing complexity for a smooth progression. For a more specific application to the modelling and prediction of antimicrobial activities, the reader can refer to Najjar and co-workers [48].
The programming of an artificial neurone (AN) fully mimics the functions of a biological neuron. Each AN receives a certain number of inputs, each of them with its own “weight”, thus indicating its importance among other inputs. The AN will sum up all weighted inputs and if the resulting value exceeds a “threshold” (also known as “bias” or “noise”) the sum is then processed using a “transfer function” and the result is distributed through the output to the next AN.
Each single AN works in a team of “ANNs” thus also mimicking the biological pattern of a neural network (NN) of interconnected neurons in a living organism. The “anatomy” of an ANN is defined by many factors such as the number and arrangements of layers of neurons, their interconnections, etc. Two of these layers are connected to the “outside world”: the “input layer” where data is presented, and the “output layer”, where the prediction value is obtained. All the other layers (hidden layers) are made up by neurons connected to each other, usually excluding neurons of the same layer.
ANNs may be arranged in various “anatomies” or “topologies”. Here, only the multilayer feed-forward ANN (MLF-ANN) is described in detail as it is one the most preferred when a prediction of discrete numbers measuring bioactivities or chemical properties is needed. Others, such as Kohonen self-organising network, are popularly used for classification problems. MLF-ANNs consist of multiple layers of interconnected nodes, or neurons. Information flows in a single direction, from the input layer through one or more hidden layers to the output layer. This simple yet powerful architecture makes MLF-ANNs well-suited for tasks like predicting numerical values, such as bioactivity or chemical properties. These are particularly useful to create multivariate models on science. There are no cycles or loops in the network.
Human intelligence is the most important factor in creating a successful AI model, as the ANNs are trained with data that are collected and curated by human researchers. A partial or irrelevant dataset will result in a seriously biased or noisy AI model, respectively. Part of the data (input/variables and output/results) are selected to train the ANN. In a “supervised” model, the algorithm then calculates the output with current weights and biases using a series of “cycles”. Each one tries to approach the output, and the resulting value is compared with the real output to inform the algorithm on how to adjust the weights and biases. This cycle is repeated until the difference between targeted and calculated values is as close as it can get. The most applied supervised algorithms are based on gradient methods [for example ‘back propagation’ and genetics (genetic algorithms)]. While the supervised learning algorithm requires the knowledge of output values, the unsupervised does not need them. It produces its own output which needs further evaluation by humans.
Once the ANN has completed its training process, it’s crucial to assess its performance. This involves testing the network’s ability to predict outcomes on a separate validation dataset. By providing only the input values to the network, we can compare its calculated outputs to the actual known outputs. This comparison, often visualized graphically by linear regressions, allows us to evaluate the network’s accuracy.
Limitations of AI approaches
The above claims about the capabilities and benefits of AI modelling to leverage the bioactivity of complex NPs in CVD have to be put into the right context to understand its limitations in the field of complex biological interactions. By definition, the algorithm of a NN relies on the weights of every interaction between the individual ANs and these are set by trial and error after training with a dataset which is always large but not exhaustive and even could contain noise or be biased as previously explained by Krogh [54], Dohnal et al. [47], and Zupan and Gasteiger [55]. Therefore, it is not based on a true understanding of the underlying biological processes. This “true” understanding is only achieved using other methods such as “systems biology” or “network biology” using tools such as Cytoscape [56]. They rely on “computational biological networks” which have been built with current scientific mechanistic understanding [57]. Another similar powerful tool is “gene network”, as highlighted in our recent approach to understanding the cytotoxic effects of NPs against melanoma cells [58]. In short, whilst ANNs are powerful, their reliance on high quality training data is critical and their predictions warrant caution and constant validation with other approaches.
Methodological aspects for the modelling of antioxidant and antimicrobial volatiles in EOs
The key aspects of the dataset curation are the data retrieval and the selection of inputs. The dataset for the antimicrobial volatiles in EOs was made of scientific reports using the National Committee for Clinical Laboratory Standards standardized method for zone diameter measurements [59]. Only those reporting similar inhibitory values for the same antibiotics of reference were retained in order to minimise the “noise” of the dataset. The selected EOs comprised 180 different compounds (inputs). To reduce the complexity of the NN, two strategies were considered to reduce the number of inputs: (1) retain the compounds with evidence of antimicrobial properties only or (2) discard the compounds without known antimicrobial activity and/or present at very low percentages (≤ 5%). The first strategy resulted in 22 inputs (Table 1), while the second 75 (the 22 principal antimicrobial agents present in the first one plus other major compounds characteristic of the EOs with unknown or non-significant antimicrobial activity). The second dataset was not better than the first in the predictions. In this case, the value of the inhibition was normalised between 0 and 100. The criteria to classify the accuracy of the predictions was based on their deviations from the reported (“real”) inhibitory diameters (ΔID): ΔID ≤ 5 mm was considered as very accurate, ΔID ≤ 10 mm represented accurate predictions, and ΔID > 10 mm would mean mistaken predictions [33].
Volatile compounds selected as inputs for the ANN modelling of antioxidant activity on in vitro models of lipoperoxidation and antimicrobial activity against Staphylococcus aureus, Escherichia coli, Candida albicans, and Clostridium perfringens
Volatile compound | Antioxidant [32] | Antimicrobial [33] |
---|---|---|
(E)-Anethole | ||
syn-7-Hydroxy-7-anisylnorbornene | - | |
Borneol | ||
Bornyl acetate | - | |
Camphene | - | |
Camphor | ||
Carvacrol | ||
Carvone | - | |
Caryophyllene | - | |
p-Cymene | ||
Eucalyptol | ||
Eugenol | - | |
Geijerene | - | |
Limonene | ||
Linalool | ||
Linalool oxide | - | |
Menthone | ||
Myrcene | - | |
Myrtanol | - | |
Nerolidol (or peruviol) | - | |
Ocimene | - | |
1-Octen-3-ol | - | |
α-Pinene | ||
β-Pinene | ||
Piperitone | - | |
Pregeijerene | - | |
Pulegone | ||
Sabinene | - | |
α-Terpinene | - | |
γ-Terpinene | ||
Terpinolene | - | |
Terpinen-4-ol | ||
α-Terpineol | ||
α-Thujene | - | |
Thymol | ||
Thymol methyl ether | - |
Grey cells indicate volatiles chosen as inputs for the antioxidant and/or antimicrobial activity prediction by artificial neural networks (ANNs). White cells with “-” signify that the compound was not selected as an input for the activity prediction
To reduce noise data within the antioxidant activity dataset articles reporting similar results (±10%) for the positive control butylhydroxytoluene (BHT) in order as much as possible. This resulted in 80 inputs of which only 30 volatiles (Table 1) with the highest antioxidant activity were selected as inputs [32].
ANNs were developed and run on a personal computer using fast artificial neural network software ver. 1.2.0-1. This package was downloaded from its original repository (http://leenissen.dk/fann/) and installed following the guidelines provided by the developers. The design was always feed forward, multilayer, and with back-propagation. The selection of function set at Sigmoid and other settings optimised for each type of output.
Harnessing the power of EOs against S. aureus, E. coli, C. albicans
S. aureus
Coagulase-negative staphylococci and S. aureus are the major causative pathogens of infections associated with CVD. These instances are increasing and are a cause of significant morbidity and mortality [60]. S. aureus is a type of bacteria that can be both harmless and harmful to humans. Around 30% of people carry this bacterium on their bodies without experiencing any ill effects. However, S. aureus is also a major cause of various infections, chiefly bloodstream infections and heart valve infections, and infections associated with medical devices [61]. S. aureus endocarditis is debilitating and requires prompt diagnosis and management for enhanced positive outcomes. To further complicate matters, the rise of methicillin-resistant S. aureus (MRSA) valvular endocarditis is causing greater morbidity and mortality rates requiring antibiotic regimens frequently limited by toxicities [52, 61]. The development of new antibiotic agents is one of the strategies sought by the experts to overcome such caveats [62]. In this sense, numerous EOs from well-known and sustainable plant species have been reported to be superior to current antibiotics against MRSA in experimental conditions: nigella [63], eucalyptus, and peppermint [64] have minimum inhibitory concentration (MICs) in the order of 1–15 μg/mL but cinnamon, thyme, clove, citronella, and eucalyptus are active at 0.1–0.4 μg/mL [64].
Challenges to the use of complex chemical entities include variable efficacy and difficulty in ascribing antibiotic activity to a single component. For example, coriander EO (34 components) and its component linalool (accounting for 70% of the oil) inhibited bacterial growth with MIC values of 5.44 and 5.36 μg/mL, respectively, thus clearly implying that the latter is responsible for all the activity of the oil [65]. However, the activity of the whole coriander oil from other origins against methicillin-susceptible S. aureus (MSSA) was in the same range of MICs against MRSA [66, 67] or even double [68, 69]. In the case of tea tree oil, no correlation could be established between the antibiotic activity of the oil and its putative bioactive component 4-terpineol on MRSA. Moreover, the same authors reported that only 4 out of 10 similar tea tree oil batches were active against MRSA [70], thus highlighting the issue of natural variability of the sources [71].
The synergies between certain EOs or some of their components with antibiotics are also reported. EOs from coriander, ivy, and onion combinations exhibited synergistic activity with cefotaxime against S. aureus clinical isolates [68]. Cumin, parsley, coriander, origanum, chamomile, basil, leek, peppermint, and spearmint also killed 100% of MRSA when in combination with cefotaxime [68], and the EO of Chinese ginger (Boesenbergia rotunda) potentiated cloxacillin [72]. Turpentine EO boosted the effectiveness of norfloxacin and gentamicin against both Gram-negative and Gram-positive bacteria. It also amplified penicillin’s activity against Gram-negative bacteria [73].
We demonstrated that ANNs take into account the myriad of synergies, antagonisms, additive effects and the variability of the composition from batch to batch and still make an accurate prediction of the antibiotic effect of EOs upon MRSA [33] as seen in Figure 3.
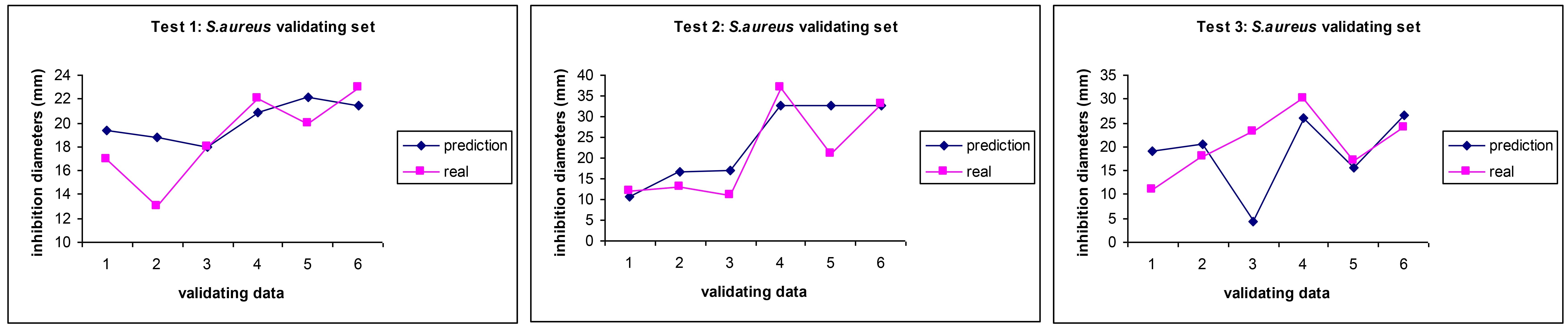
Comparison between experimental values (pink squares) and AI prediction (blue diamonds) for the antibiotic activity (MICs) of essential oils on MRSA in three independent AI predictions. Reprinted from [33]. CC BY. MICs: minimum inhibitory concentration; MRSA: methicillin-resistant S. aureus; ANN: artificial neural network; AI: artificial intelligence
E. coli
E. coli is one of the most evasive bacteria causing endocarditis and sepsis due to its innate propensity to mutations and prolific exchange of plasmids carrying antibiotic resistance genes [74]. Therefore, finding new agents able to either kill it or enhance the efficacy of antibiotics is a research priority. In fact, a search for EOs with E. coli antibiotic effects will yield over 1,800 results in PubMed, most of them are screenings of EOs against one or more E. coli strains, highlighting the importance of this infective agent in NPs discovery and justifying the impossibility to cover all this wealth of knowledge. But in recent years, a significant number of them have been directed towards understanding the synergies between the EOs to reduce MICs values. To this end, researchers are using advanced multivariate strategies or mathematical models of synergy, from chequerboard methods to more refined measures of synergy such as the one Chou-Talalay REF. For example, to enhance the antibacterial efficacy of EOs derived from Eucalyptus camaldulensis and Mentha pulegium, against E. coli, Kachkoul and co-workers employed a polynomial model developed using an augmented centroid simplex mixture design to identify optimal synergistic combinations of these EOs [75]. The individual EOs demonstrated significant inhibitory effects against E. coli, but their binary combinations exhibited a synergistic effect, surpassing the individual activities of the constituent EOs and yielding the lowest MICs against E. coli [75]. This method was also exploited by finding enhanced antimicrobial activity for three of the EOs from Thymus satureioides (“Azkuni thyme”), Myrtus communis (Mirtle), and Artemisia herba alba (white wormwood) against E. coli, S. aureus, and C. tropicalis [76].
There is also an increase in recent works dealing with the study of how individual volatile components may act in synergy as reported for and eugenol, cinnamaldehyde, thymol, and carvacrol against E. coli [77].
There is less abundance of studies in vivo. A study in point explored the potential of thyme EO dominated by thymol and 9,12-octadecadi(Z,Z)enoic acid to counteract the harmful effects of E. coli O157:H7 infection in rats. When rats were infected with E. coli O157:H7, they experienced significant changes in their blood, including increased red blood cell count, haematocrit, haemoglobin, white blood cell count, and platelet count. Additionally, the infection led to elevated levels of liver and kidney function markers, as well as increased levels of inflammatory cytokines and immunoglobulins. The infection also caused visible damage to the colon, liver, and kidneys. However, when the infected rats were treated with thyme EO, these harmful effects were mitigated. The oil helped to restore normal blood parameters, improve liver and kidney function, reduce inflammation, and lessen tissue damage [78].
In our works applying ANNs to predict the antibiotic activity of EOs against bacteria, we found that the results mirroring the difficulty and variability of therapies against the E. coli as revealed by a higher discrepancy in the prediction vs. real values in some cases (Figure 4), although in average the ANNs managed to be able to be as good as the disk inhibition model, which shows a lack of finesse and reproducibility when dealing with multiresistant and highly mutable bacteria [33]. We also suspect a lack of standardisation of the methods in many of the works published and we noticed a high variability in the choice of standard antibiotic by the research community, thus making the curation of the dataset very challenging and therefore increasing the noise of the model. We therefore call for a stricter adherence to standard operating procedures and a more careful identification of the strains used in research.
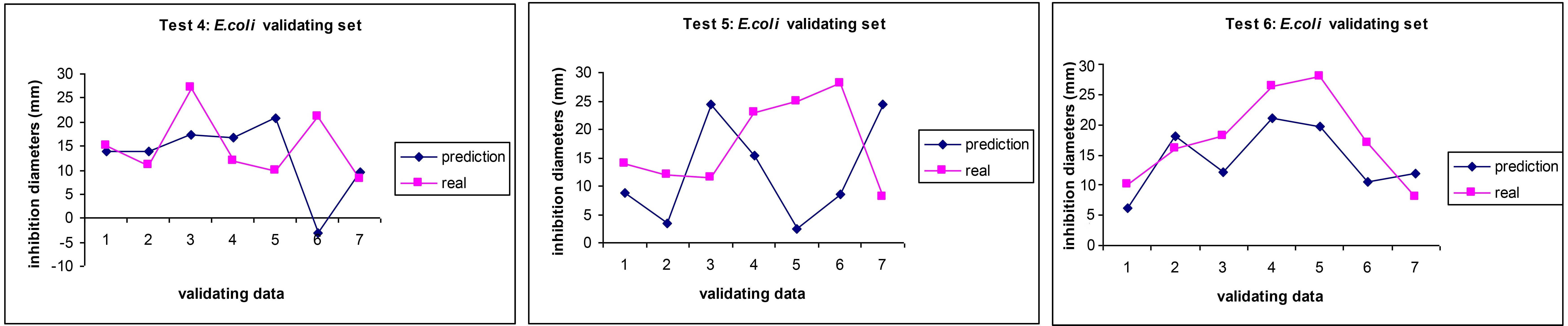
Comparison between experimental values (pink squares) and AI prediction (blue diamonds) for the antibiotic activity (MICs) of essential oils on E. coli in three independent AI predictions. Adapted from [33]. CC BY. MICs: minimum inhibitory concentration; ANN: artificial neural network; AI: artificial intelligence
C. albicans
Candida spp. belongs to the kingdom fungi. The close evolutionary relationship between fungi and humans makes it difficult to identify drug targets that selectively inhibit fungal growth without harming human cells, resulting in limited therapeutic options and a high rate of drug development failures [79]. Furthermore, the emergence of drug-resistant fungal strains, complex clinical trial designs, and a lack of reliable diagnostic tools for accurately identifying fungal infections further complicated antifungal drug development until now [80]. As a result, therapies against Candida spp. are difficult to be as specific and effective as against bacteria, vastly relaying on azole antifungals which target the exclusive use of ergosterol by fungi [81]. As commented in the previous section, there is an enormous number of works screening EOs against one or more Candida species, with a higher focus in recent years on EOs-EOs or EOs-antibiotics synergies [82]. Kamble and Phadke [83] described the use of the checkerboard approach to unveil a synergy between the EO of the leaves of Bengal quince (Aegle marmelos) and nystatin against C. albicans. The method of simplex-centroid design was applied against C. tropicalis [76]. Khan and co-workers screened 17 EOs from common food spices (grapefruit, orange, sweet fennel parsley, celery, rosemary, sandalwood, corn, ginger, cinnamon, lemon, nutmeg, eucalyptus, peppermint lemongrass palmrosa and clove) and four pure (eugenol, cinnamaldehyde, citral and geraniol) against 18 isolates of clinical origin and two reference strains of C. albicans [84]. A checkerboard microtiter test was performed to evaluate the interaction of EOs or active compounds with fluconazole or amphotericin B. Cinnamaldehyde and EO of palmarosa resulted in the most active against all strains and geraniol (a moderate anti-candidal itself) showed a significant level of synergy with both antibiotics as shown by 16- to 32-fold reductions of their MICs [84]. Hleba and co-workers investigated the antifungal potential of twelve EOs and their combinations against four Candida species: C. albicans, C. glabrata, C. tropicalis, and C. parapsilosis [85]. The chemical composition of the EOs was determined using GC-MS and GC-MS flame ionization detector (FID) techniques. The antifungal susceptibility of the Candida strains was assessed using the VITEK-2XL system and the checkerboard method to calculate the fractional inhibitory concentration indexes. The results indicated that ginger, ho-sho (Cinnamomum camphora Nees and Eberm var. Linaloolifera fujita), absinth, dill, fennel, star anise, and cardamom EOs exhibited the highest antifungal activity. Among them, synergistic effects were observed for combinations of ginger/fennel and absinth/fennel against multiple Candida species [85]. In a similar study, synergistic effects of the cinnamon/fluconazole and rose geranium/fluconazole combinations on C. albicans were unveiled, and the mechanism of action revealed that cinnamon EO reduced the synthesis of ergosterol by 80% whilst rose geranium disturbs the permeability barrier of the fungal cell wall [86].
For less recent examples of such synergies, the reader is referred to the excellent review by Bhattacharya et al. [82]. These findings suggest that the combination of EOs may offer a promising strategy to combat antifungal drug resistance.
The application of in silico methods to predict the antibiotic effects of complex NPs against C. albicans started with the pioneering work of Buciński and co-workers [87]. Our work using a significantly higher number of inputs allowed us to make more informed predictions, but (as observed for E. coli) the treatment outcomes were less accurate than for bacteria (Figure 5), indicating the higher biological complexity of fungi which also pose serious challenges for current therapeutic interventions in CVD [12].
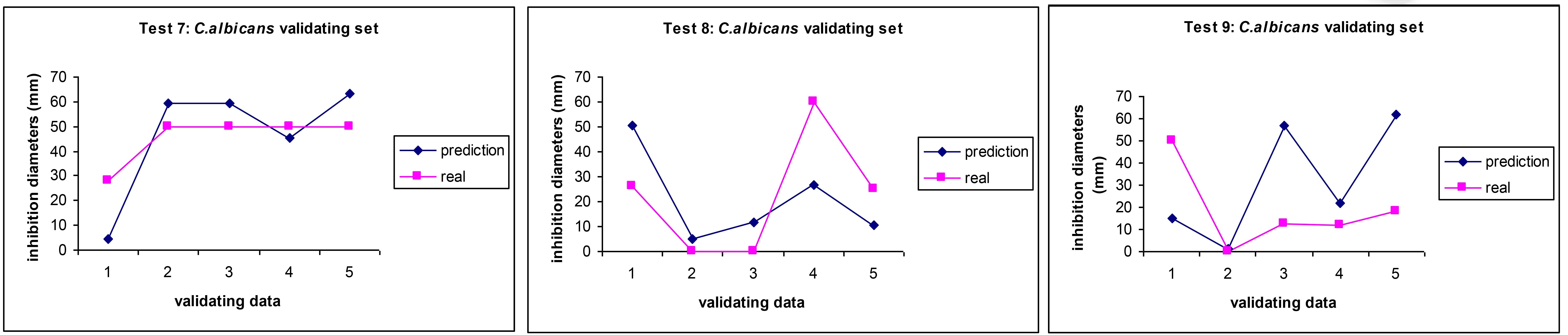
Comparison between experimental values (pink squares) and AI prediction (blue diamonds) for the antibiotic activity (MICs) of essential oils on C. albicans in three independent AI predictions. Reprinted from [33]. CC BY. MICs: minimum inhibitory concentration; ANN: artificial neural network; AI: artificial intelligence
Broad-spectrum EOs and their synergies with antibiotics: a “Swiss-army knife” against cardiovascular pathogens
Many EOs can kill or inhibit the growth of multiple types of microorganisms, therefore qualifying as “broad-spectrum antibiotics”. For example, cuminaldehyde, a major component of cumin EO, but also found in the EOs of eucalyptus, myrrh, and cassia is able to inhibit the growth of S. aureus (ATCC 6538) and E. coli 042 (EAEC 042) as well as some of their clinical isolates with MICs 12–24 mg/mL. Although this may seem a modest value, its ADME (absorption, distribution, metabolism, and excretion) in silico profile was deemed quite promising [88]. A similar dual S. aureus-E. coli antibiotic effect was reported for ginger EO with zingiberene and α-curcumene as the main components [89]. Interestingly, the EO of Dalbergia pinnata (composed of 91% elemicin, and 13 other minor compounds) scavenges free radicals as well as inhibiting the growth of S. aureus (MIC = 0.78 μL/mL), and C. albicans (MIC = 12.5 μL/mL) without any effect on E. coli, thus acting as both antibiotic and antioxidant at the same time [90]. Kamble and Phadke [83] described the synergy between Moroccan thyme (Thymus satureioides), mirtle (Myrtus communis), and white wormwood (Artemisia herba-alba) EOs against E. coli, S. aureus, and C. tropicalis using the classic checkerboard approach. In vivo studies suggested that thyme EO also possesses multiple protective effects, including anti-inflammatory, antioxidant, and immunomodulatory activities [78].
Whilst, most EOs have been reported to have potential therapeutic applications due to their low MICs, others with negligible antibiotic activity have been reported to enhance the effect of clinically used antibiotics but acting in synergy with them. One such NP is the Schinus terebinthifolius (turpentine) EOs (which contains alpha-pinene, gamma-muurolene, and myrcene as its major components) is devoid of clinically effective antibacterial activity but enhances the activity of norfloxacin and gentamicin against both E. coli and S. aureus [91]. Synergistic antibacterial effects were also observed with combinations of thymol and carvacrol, eugenol, cinnamaldehyde, thymol, and carvacrol against E. coli [77] and fluconazole with cinnamaldehyde/eugenol/limonene/eucalyptol showed a synergistic effect on C. albicans [92].
We demonstrated that ANNs can also predict the activities of EOs on two or more bacteria at the same time when properly trained, acting as a predictor tool for the antibiotic spectrum of complex NPs [33]. Moreover, our model only needed a representative number of the components (22 out of 179 different compounds). We are certain that predicting several bioactivities at the same time is also possible using AI, thus creating a shortcut to faster drug discovery in the CVD domain and others to be accurate enough to predict the activity of these complex chemical products (Figure 6).
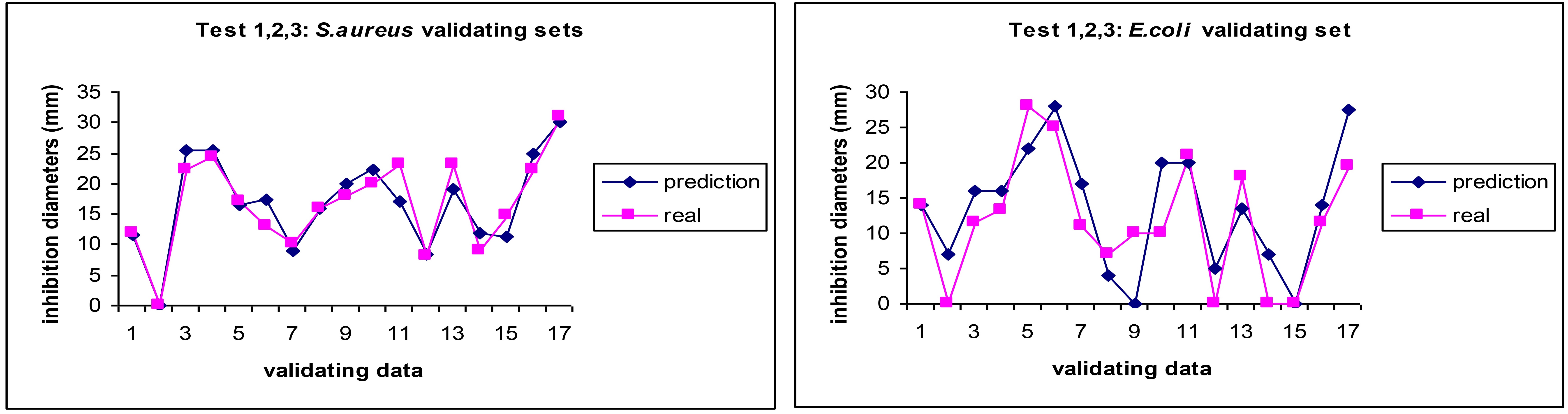
Comparison between experimental values (pink squares) and prediction (blue diamonds) by an ANN trained to predict the antibiotic activity (MICs) of essential oils on both S. aureus and E. coli. Reprinted from [33]. CC BY. MICs: minimum inhibitory concentration; ANN: artificial neural network; AI: artificial intelligence
Harnessing the power of EOs for oxidative damage mitigation and anti-inflammatory activities
Oxidative stress, a condition characterized by an imbalance between oxidants and antioxidants, is implicated in the pathogenesis of CVD. This imbalance leads to increased oxidative damage, inflammation, and endothelial dysfunction [20]. Early studies suggested a protective role of dietary antioxidants like vitamin C, vitamin E, and beta-carotene against CVD. However, subsequent randomized trials often failed to demonstrate significant benefits. This discrepancy may be due to factors such as incorrect antioxidant choice, dose, or patient selection [93]. Nuclear factor erythroid 2-related factor 2 (Nrf2) is a transcription factor that plays a crucial role in cellular defense against oxidative stress. It activates the expression of various antioxidant and cytoprotective enzymes. NPs have gained attention as potential sources of bioactive compounds with Nrf2-activating properties. These compounds may offer a promising therapeutic approach for CVD prevention and treatment [94]. Another effect of “oxidative bursting” is the direct oxidation of structural and/or functional lipids (lipoperoxidation) such as phospholipids and cholesterol of the cell membranes, lipoproteins [95, 96], and eicosanoids [97].
Direct lipoperoxidation is modelled in vitro using omega 3 lipids such as linoleic acid [98] or plasma lipoproteins [99]. To our knowledge, the first report showing the possibility of applying ANNs to predict the antioxidant capacity of NPs was written by Bucinski and co-workers [100] and then our pioneering work to predict the antioxidant activity of EOs in the widely used in vitro model of linoleic acid oxidation achieving an average error of only 1–3% opened the way to predict the antioxidant capacities of EOs taking into account 30 components (Table 1) from a total of 80 [32].
Since the epidemiological discovery of the so-called “French Paradox”, many experimental studies and meta-analyses have mainly attributed this outcome to the presence in red wine of a great variety of polyphenolic compounds such as resveratrol, catechin, epicatechin, quercetin, and anthocyanins [101]. Since, dietary polyphenols have been enshrined as major protection factors to help prevent and potentially treat cardiovascular disorders, atherosclerosis, and keep the integrity of the vascular endothelium [102]. However, our findings indicate that their contribution is less relevant in EOs [32] thus, opening new avenues to unravel new mechanisms to prevent CVD disease with volatile terpenes such as those listed in Table 1.
There is also recent evidence of the link between EOs, reactive oxygen species (ROS), and antifungal effects. Contrarily to bacteria, fungi are eukaryotic cells and therefore, susceptible to experiencing mitochondrial dysfunction leading to deleterious ROS intracellular production leading to cell cycle arrest. Moreover, fungi use ROS to drive their transition to the hyphal state [103]. These mechanisms can be disrupted using EOs as shown in the model yeast Saccharomyces cerevisiae [104]. Shahina and co-workers demonstrated how rosemary EO and two of its main components (1,8-cineole and α-pinene) induce ROS-dependent lethality and ROS-independent virulence inhibition in C. albicans using a chemogenomic profiling approach, advanced quantitative laser scanning confocal and atomic force microscopy to ascertain these mechanisms [105].
The modelling and prediction of purely chemical reactions involving natural molecules and ROS can be envisioned as relatively straightforward, even when considering a substantial number of reactants, the complexity of biological processes, such as inflammation, poses a far greater challenge. Antimicrobial activities, while involving intricate biological interactions, may still be less complex than those occurring within multicellular organisms. A first attempt to use ANNs in the prediction of the anti-inflammatory activities of complex natural (extracts from Asteraceae species) using untargeted metabolomics with no prior knowledge of their biological data achieved a good degree of prediction [106]. We recently established a multivariate statistical relationship between the inhibition of lipoperoxidation and anti-inflammatory effect in a number of plant extracts [16] and we are working on the ANN modelling of the interplay between infectious agents, lipoperoxidation and pro-inflammatory mediators shown above in Figure 1. Future efforts to achieve translational in silico predictions of in vitro processes should follow up in the manner indicated by the pioneering work by Parojcić and co-workers [107].
Conclusions
This review explored the potential of ANNs in predicting the bioactivities of complex chemical entities like NPs, particularly volatile compounds (EOs). The increasing number and diversity of research publications demonstrate the promising role of ANNs in addressing infectious diseases and related oxidative stress.
To fully harness the potential of ANNs, collaborative efforts between multidisciplinary teams are crucial to generate high-quality experimental data and analyse it effectively. The author envisions a future where omics technologies and systems biology will provide real-time data to cloud-based ANNs, enabling increasingly precise predictions and classifications of the beneficial activities of complex NPs. In conclusion, the integration of AI in the analysis and prediction of the effects of complex NPs could revolutionize the field, leading to more consistent, effective treatments. This approach (in combination with other computational tools such as biological networks to overcome some intrinsic limitations) could help address pressing health challenges, from antibiotic resistance to chronic cardiovascular conditions, offering significant benefits for public health and healthcare economics.
A significant challenge in translating AI-designed EOs into clinical practice lies in safeguarding intellectual property (IP) while ensuring the commercial viability of research and development (R & D). While a patent can effectively protect the specific composition of an “AI-designed EO”, the costs associated with clinical trials and regulatory approval may render a potentially inexpensive complex substance prohibitively expensive as a medicinal product.
Furthermore, attributing therapeutic efficacy to EOs from specific plant sources presents a significant hurdle. The inherent regional variability in EO composition, arising from factors such as plant origin and extraction methods, poses a substantial challenge to the stringent standardization requirements of the pharmaceutical industry.
To address this, the WHO could play a crucial role by establishing “minimum specifications and variability ranges” for promising EOs. These guidelines would ensure consistent efficacy while enabling pharmacists worldwide, including those in resource-limited settings such as developing countries and isolated communities, to prepare and dispense EOs according to standardized monographs, like the approach used for many other herbal medicines listed in the WHO Pharmacopoeia.
Therefore, future work needs to address the ethical considerations of the technological-scientific-clinical interface and decide if EOs should become proprietary or free to the world.
Abbreviations
AI: | artificial intelligence |
AN: | artificial neurone |
ANNs: | artificial neural networks |
CVD: | cardiovascular diseases |
EOs: | essential oils |
FDA: | Food and Drug Administration |
GC-MS: | gas-chromatography mass-spectrometry |
HDL: | high-density lipoprotein |
LC-MS: | liquid-chromatography mass-spectrometry |
LDL: | low-density lipoprotein |
LOX: | lipoxygenase |
LPS: | lipopolysaccharide |
LTB4: | leukotriene B4 |
MDA: | malondialdehyde |
MICs: | minimum inhibitory concentration |
MLF-ANN: | multilayer feed-forward artificial neural network |
MRSA: | methicillin-resistant S. aureus |
NPs: | natural products |
Nrf2: | nuclear factor erythroid 2-related factor 2 |
oxLDL: | oxidized low-density lipoprotein |
PGE2: | prostaglandin E2 |
ROS: | reactive oxygen species |
WHO: | World Health Organization |
XOD: | xanthine oxidase |
Declarations
Author contributions
JMPG: Conceptualization, Investigation, Writing—original draft, Writing—review & editing.
Conflicts of interest
The author declares that he has no conflicts of interest.
Ethical approval
Not applicable.
Consent to participate
Not applicable.
Consent to publication
Not applicable.
Availability of data and materials
Not applicable.
Funding
Not applicable.
Copyright
© The Author(s) 2025.
Publisher’s note
Open Exploration maintains a neutral stance on jurisdictional claims in published institutional affiliations and maps. All opinions expressed in this article are the personal views of the author(s) and do not represent the stance of the editorial team or the publisher.