Abstract
In the last years, multiple efforts have been made to accurately predict neoantigens derived from somatic mutations in cancer patients, either to develop personalized therapeutic vaccines or to study immune responses after cancer immunotherapy. In this context, the increasing accessibility of paired whole-exome sequencing (WES) of tumor biopsies and matched normal tissue as well as RNA sequencing (RNA-Seq) has provided a basis for the development of bioinformatics tools that predict and prioritize neoantigen candidates. Most pipelines rely on the binding prediction of candidate peptides to the patient’s major histocompatibility complex (MHC), but these methods return a high number of false positives since they lack information related to other features that influence T cell responses to neoantigens. This review explores available computational methods that incorporate information on T cell preferences to predict their activation after encountering a peptide-MHC complex. Specifically, methods that predict i) biological features that may increase the availability of a neopeptide to be exposed to the immune system, ii) metrics of self-similarity representing the chances of a neoantigen to break immune tolerance, iii) pathogen immunogenicity, and iv) tumor immunogenicity. Also, this review describes the characteristics of these tools and addresses their performance in the context of a novel benchmark dataset of experimentally validated neoantigens from patients treated with a melanoma vaccine (VACCIMEL) in a phase II clinical study. The overall results of the evaluation indicate that current tools have a limited ability to predict the activation of a cytotoxic response against neoantigens. Based on this result, the limitations that make this problem an unsolved challenge in immunoinformatics are discussed.
Keywords
Neoantigen, cancer vaccine, melanoma, machine learning, neoepitope predictionIntroduction
Neoantigens are defined as patient-specific antigens that arise from tumor-specific genetic variations such as somatic mutations, gene fusions, and alternative splicing variants [1]. Other variants that expand the repertoire of targetable neoantigens for cancer immunotherapy are derived from aberrant transcription-induced chimeric RNAs, generated from trans-splicing of precursor mRNAs or via cis-splicing of adjacent genes [2, 3], post-translational modifications [4, 5], and transposable elements [6]. In consequence, neoantigens are only expressed in tumor tissues and thus, the immune response against them is highly tumor-specific. Multiple studies have demonstrated that T cells can recognize these neoantigens and distinguish tumor cells from normal cells [7]. In this scenario, targeting strong immunogenic neoepitopes has relevant therapeutic potential. Highly mutated tumors allow the emergence of more neoepitopes to be recognized by T cells and indeed, have a better response to immunotherapy with monoclonal antibodies that block immune checkpoints (ICI). This has been demonstrated in melanoma as well as in lung cancer, urothelial cancer [8], and in mismatch repair-deficient tumors [9]. But even if tumor mutational burden (TMB) is high, major histocompatibility complex (MHC) allele homozygosis or the expression of MHCs with highly similar recognition motifs can limit the number of presented peptides in a given individual [10].
However, the generation of immunogenic neoepitopes is not the only factor that influences whether a variant results in clinically relevant tumor cell recognition and lysis by T cells. Proteins containing mutated peptides must be efficiently transcribed, translated, processed by the antigen-processing cells, and loaded onto MHC molecules for presentation on the cell surface to be recognized by a T cell (Figure 1). Alterations in genes that modulate these processes, as well as downregulation of MHC expression in tumor cells, can abrogate the immunogenicity of neoepitopes in cancer patients [11, 12].
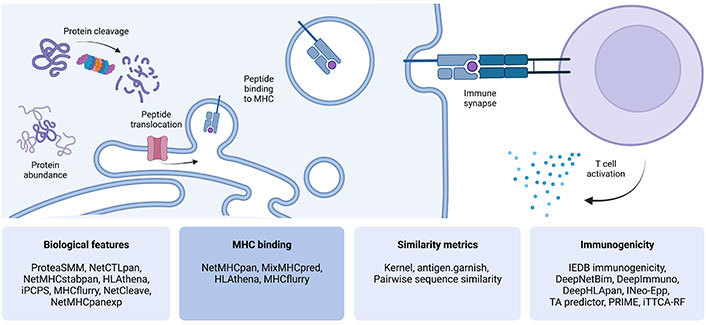
Steps involved in antigen processing, presentation, and T cell recognition. Four categories of computational predictive tools that address each aspect were defined, which are displayed in the lower panels. This figure was created with BioRender.com. iPCPS: improved proteasome cleavage prediction server; IEDB: Immune Epitope Database; INeo-Epp: immunogenic epitope/neoepitope prediction; TA predictor: tumor antigen predictor; PRIME: Predictor of Immunogenic Epitopes; iTTCA-RF: Identification of Tumor T cell Antigens-Random Forest
Accurate neoepitope prediction pursues several purposes: i) to design personalized cancer treatments, such as neoantigen-targeted vaccines [13–15] and adoptive cell therapies [16], where the immune system is stimulated to recognize neoantigens, increasing the frequency of specific CD8+ T cells and potentially eliciting the selective destruction of tumor cells; ii) to develop tools for the screening of neoantigen-specific T cells in samples of patients receiving immunotherapies, such as cancer vaccines [17], tumor infiltrating lymphocytes (TILs) [18] or ICI [19]. As such, immunoinformatic tools are currently applied to guide scientists to select the best potential neoepitope candidates, for instance, to elaborate vaccines, however, their immunogenicity must be experimentally verified after patient treatment.
Since the introduction of paired whole-exome sequencing (WES) of tumor/normal tissue and RNA sequencing (RNA-Seq) technologies, along with the development of refined bioinformatics tools applied to predict candidate neoepitopes, the field has accelerated considerably fueling precision oncology. The neoepitope prediction strategy involves somatic alteration identification and annotation from paired sequencing data of tumor and normal DNA or RNA, prediction of neopeptides presentation in the context of the patient’s MHC alleles, and prioritization among candidate neopeptides [20]. Examples of well-established pipelines that perform these tasks for mutation-based neoantigens are personalized variant antigens by cancer sequencing (pVAC-Seq) [21], mutant peptide extractor and informer (MuPeXi) [22], prioritizing tumor neoantigens (pTuneos) [23], and Neopepsee [24]. Further, there are methods such as INTEGRATE-neo [25], NeoFuse [26], nextflow neoantigen prediction pipeline (nextNEOpi) [27], and deFuse-Trinity [28] designed to detect gene fusion and/or aberrant transcription-induced chimeric RNAs-based neoantigens. In particular, methods developed to predict the binding of peptides to MHC molecules are very precise and have contributed to facilitating neoantigen prediction. The binding of these neopeptides to MHC molecules is critical to inducing an effective immune response but it is not the only factor determining immunogenicity. For this reason, pipelines relying on MHC binding still return a considerable amount of false positive predictions [29, 30]. In addition, every single mutation can generate different peptides that vary in length and frame, which may bind to any of the six patient’s MHC class I molecules [31]. In this context, prioritization of reliable neoepitope candidates becomes critical.
Assuming high-affinity binding to MHC alleles for mutated peptides, evaluation of additional features, such as gene expression and detection of variants in RNA-Seq, variant allele frequencies, neoepitope enrichment within a particular intracellular compartment, microbial sequence similarity, neopeptide binding and stability to MHC and/or peptide processing, may increase the specificity and sensitivity of neoantigen prediction [32]. To address the suppressive response that may be activated if the recognized antigen shares similarity to a self antigen, self-similarity metrics have been also proposed to improve neoantigen prediction [33]. Additionally, only a few neopeptides are actually recognized by T cells [13, 34, 35] at the immune synapse and the prediction of the multiple requirements for the effective interaction between the peptide-MHC (pMHC) and T cell receptor (TCR) is still challenging.
This review explores and compares some of the most relevant or recent computational methods that account for T cell preferences to predict their activation after the encounter with a pMHC complex, and these methods could be used after the application of the mentioned WES pipelines to refine neoantigen candidate selection. This review also includes features that may impact on the immune response, such as protein processing or abundance. In each section, reviewed tools will appear in order by date of publication. Of note, it is not intended to give an exhaustive revision covering all tools, but rather focus on covering some of the most representative tools within each domain. This article focuses on MHC class I presented peptides (usually 8–11 mers) since the role of CD8+ T cells is central in antitumor immune response and peptide binding prediction to MHC class I can be more accurately established with the methodology currently developed [36]. Finally, with a novel dataset of experimentally validated neoepitopes from melanoma patients that were treated with a therapeutic vaccine (VACCIMEL) in a phase II clinical study [37, 38], the tools were evaluated in an unbiased way.
Biological and immunological features associated with neopeptide immunogenicity
The availability of neopeptides at the surface of target cells and/or antigen-presenting cells (APC) is essential for T cell recognition. For this to happen, a mutated protein must be transcribed and translated by the tumor cell, cleaved by specific proteases, and processed through the antigen-processing machinery of the cell to produce peptides that are translocated to the endoplasmic reticulum lumen by transporter associated with antigen-processing (TAP) molecules, to form a complex with the MHC molecule. In the cases of MHC class I antigen cross-presentation and exogenous MHC class II antigen presentation, mutated proteins from tumor cells must be captured by the APC and then presented to activate peptide-specific naive T cells. Thus, the use of bioinformatics tools that predict proteasome cleavage, immunoproteasome processing, TAP affinity, and MHC binding may help to select better candidate neopeptides [39]. The initial limitation to the generation of peptides during antigen processing consists of the source protein degradation into smaller peptides. Each processing pathway generates its specific peptidome by means of different proteolytic enzymes, and consequently, research has focused on different approaches to predict proteasome cleavage sites [40, 41]. In this regard, the class I pathway involves the proteasome (and immunoproteasome) machinery, while the class II involves, for instance, cathepsins activity. Besides, antigen abundance (the expression level of the mutated gene) [42] and pMHC stability (pMHC-I complex half-life) [43] can be extremely diverse and strongly influence neopeptide availability to specific T cells. Another feature to consider is the inherent tumor heterogeneity, meaning that poorly represented variants in the tumor would have a lower probability to elicit an effective immune response against them. In that sense, variant allele frequency or clonality can be considered to prioritize the best neopeptide candidates [44]. Specific tools accounting for these features may serve to better select candidate neopeptides and some are reviewed below (Table 1).
Predictive tools reviewed in this study
Model | Category | Performance AUC | Year/Citation |
---|---|---|---|
ProteaSMM c/i | i | 0.71/0.74 | 2005 [39] |
NetCTLpan | i | 0.94 | 2010 [40] |
NetMHCstabpan | i | 0.97 | 2016 [43] |
HLAthena | i | N/A | 2020 [44] |
iPCPS | i | N/A | 2020 [45] |
MHCflurry BA/AP | i | 0.91/0.85 | 2020 [46] |
NetCleave | i | 0.58 | 2021 [47] |
NetMHCpanexp | i | 0.82 | 2022 [50] |
NetMHCpan | N/A | 0.99 | 2017 [55] |
MixMHCpred | N/A | 0.98 | 2017 [54] |
Kernel | ii | 0.8 | 2012 [64] |
Antigen.garnish dissimilarity/IEDB score | ii | 0.85/0.70 | 2019 [65] |
Pairwise sequence similarity | ii | N/A | 2019 [20] |
IEDB immunogenicity | iii | 0.61 | 2013 [66] |
DeepNetBim | iii | 0.94 | 2021 [70] |
DeepImmuno | iii | 0.85 | 2021 [72] |
DeepHLApan | iv | 0.81 | 2019 [75] |
INeo-Epp | iv | 0.78 | 2020 [76] |
TA predictor | iv | 0.82 | 2021 [77] |
PRIME | iv | 0.81 | 2021 [80] |
iTTCA-RF | iv | 0.78 | 2021 [81] |
Tools are grouped by categories established in this article (i: biological features; ii: similarity metrics; iii: pathogen immunogenicity; iv: tumor immunogenicity), and sorted by year of publication. The AUCs were reported by the authors in the original articles. The performance corresponds to independent evaluations on epitope or neoepitope datasets, if available. If multiple evaluations were made, the average AUC is displayed. *: These methods have been evaluated with datasets that contain immunogenic peptides as positives, and other peptides as negatives. The latter may not bind to MHC molecules; †: These methods have been evaluated with datasets that contain immunogenic peptides as positives and non-immunogenic peptides as negatives, but both categories may have the same likelihood of binding to MHC. This approach is comparable to the evaluation performed in this work; ‡: This method was evaluated and included in the pTuneos pipeline. For this reason, its performance can not be assessed individually; §: The AUC corresponds to performance in cross validation; N/A: not applicable; AUC: area under the curve
ProteaSMM: For most MHC I ligands, proteasomal cleavage at the C-terminus is the first step in antigen processing [45]. ProteaSMM [46] is a tool that uses the stabilized matrix method (SMM) algorithm for predicting proteasomal cleavages. The authors constructed two different matrices that account for digests with the constitutive proteasome and the immunoproteasome respectively. The methods were developed based on in vitro experiments characterizing proteasomal cleavage, transport by TAP molecules, and MHC class I binding. Validation of the predictive algorithms was performed using a set of 390 endogenously processed MHC class I ligands, which were identified by elution mass spectrometry (MS) from different renal cell carcinomas and cell lines with known proteasome composition. ProteaSMM predicts cleavages of small peptides, whole-protein digests or the C-termini of MHC I ligands. The combined prediction model is available at http://tools.iedb.org/processing/.
NetCTLpan: This method [47] is a pan-specific MHC class I epitope predictor that integrates predictions of proteasomal cleavage from NetChop 3.0 [41], TAP transport efficiency from [48], and MHC class I binding affinity from NetMHCpan 2.3, into a MHC class I pathway likelihood score. NetCTLpan performs predictions for all MHC molecules with known protein sequences and allows predictions for 8–11 mer peptides. The predictive performance of NetCTLpan method is validated on datasets that include ligands from the SYFPEITHI [49] and Los Alamos human immunodeficiency virus (HIV) (http://www.hiv.lanl.gov/) databases. The method is available to download and as a web server at https://services.healthtech.dtu.dk/service.php?NetCTLpan-1.1.
NetMHCstabpan: Both the strength of the interaction between peptide and MHC class I, and the stability of the pMHC complex contribute to peptide immunogenicity. NetMHCstabpan [50] was developed to combine both features and improve peptide immunogenicity prediction. The neural network-based pan-specific predictor of pMHC complex stability was trained with 28,166 novel measures of pMHC stability. This tool integrates the stability predictor with NetMHCpan-2.8 in the final model with an 80% weight on affinity and 20% weight on stability. The model achieved a better prediction of MHC ligands and T cell epitopes, as compared to any of the two features alone. The method is available to download and as a web server at https://services.healthtech.dtu.dk/service.php?NetMHCstabpan-1.0.
HLAthena: Sarkizova et al. [51] used MS to obtain a large dataset of > 185,000 eluted ligands representing numerous MHC class I alleles. From this data, the authors identified peptide motifs per MHC allele and developed neural network-based models to predict MS intrinsic peptide features. HLAthena also integrates peptide cleavability, transcript abundance, and gene presentation bias in logistic regression models. Predictions with these tools were validated with data from MHC-bound peptides that were observed experimentally in 11 patient-derived tumor cell lines of various origins, verifying the correct identification of > 75% of bound peptides. The HLAthena predictors are available to use online for research purposes at http://hlathena.tools/.
iPCPS: iPCPS, is a web-based tool developed to predict proteasome cleavage sites [52]. Modeling cleavage sites resemble modeling grammatical rules and thus iPCPS used n-grams to model and predict immunoproteasome cleavage sites [40]. The proteasome model was trained on eluted MHC class I ligands, and the immunoproteasome model was trained with epitopes. The latter was evaluated for its ability to discriminate T cell epitopes using a dataset consisting of 844 unique virus-specific CD8+ T cell epitopes and their source proteins. iPCPS is available as a web server for free public use at http://imed.med.ucm.es/Tools/pcps/.
MHCflurry: In addition to elucidating MHC binding motifs, MHC ligands also reflect the antigen processing steps that occur prior to MHC binding. MHCflurry is an integrated predictor of MHC class I presentation that incorporates models for MHC class I binding and antigen processing [53]. The authors first trained a pan-allele MHC class I binding predictor on available MHC class I ligand data, including both affinity measurements and MS datasets. This data was also used as a training set for a model of antigen processing by combining MS-identified peptides (hits) with unobserved peptides (decoys), where both hits and decoys are predicted by the binding predictor to bind the relevant MHC class I alleles. The antigen processing predictor thus models the residual allele-independent sequence properties that were not learned by the first predictor. The processing predictor favored peptides consistent with previously documented motifs [53] for key antigen processing steps. Both predictors were integrated into a logistic regression model, resulting in a presentation score. All the models were evaluated on curated MS datasets. MHCflurry 2.0 is available to download at https://github.com/openvax/mhcflurry or it can be executed without installation at https://colab.research.google.com/github/openvax/mhcflurry/blob/master/notebooks/mhcflurry-colab.ipynb.
NetCleave: This is an open-source algorithm for predicting C-terminal antigen processing for MHC class I and class II molecules [54]. NetCleave architecture consists of a feed-forward neural network trained with 46 different physicochemical publicly available descriptors of the cleavage site amino acids [55, 56]. NetCleave predictions achieved great predictive power towards class I isotypes although a more modest performance was found for class II isotypes. This method is freely available at https://github.com/pepamengual/NetCleave.
NetMHCpanExp: One feature that can strongly influence the repertoire of MHC-presented ligands is antigen abundance. For this aim, NetMHCpanExp was developed integrating MHC binding and gene expression values derived from RNA-Seq experiments [57]. This model demonstrated that the incorporation of antigen abundance improved prediction accuracy for both MHC class I ligands and cancer neoantigen epitopes. Although better results are obtained by use of sample-specific abundance information, also reference expression data (i.e. The Human Protein Atlas) can be applied without a significant decrease in prediction efficiency. The tool is available at https://services.healthtech.dtu.dk/service.php?NetMHCpanExp-1.0.
Prediction of MHC binding and MHC antigen presentation
Binding of peptides to MHC molecules is the single most selective step in the recognition and processing of antigens by the cellular immune system. It has been estimated that only 1 in 200 peptides will bind to a given MHC class I molecule with sufficient strength to elicit an immune response [58]. Given this essential role, most neoepitope selection pipelines rely on the prediction of binding of neopeptides to the MHCs present in a given individual. The field of peptide binding to MHC prediction is vast, and has been reviewed in several recent publications [59, 60]. In short, prediction methods for MHC binding can broadly be divided into two methods: methods trained on in vitro binding data and methods trained on MS eluted ligands. The former methods are capable of predicting peptide binding affinity, whereas the latter methods, due to the nature of the MS eluted ligands, predict a likelihood of MHC antigen presentation integrating information related to antigen processing, MHC binding and stability. In general, methods trained on eluted ligand data have been demonstrated to be superior compared to binding affinity methods predicting epitopes [61, 62]. It is beyond the scope of this manuscript to give a comprehensive review of all methods, and some of them are described below.
NetMHCpan: This is one of the earlier developed tools that are still within the top performing [63]. The current version of NetMHCpan [36] is trained to integrate binding affinity and eluted ligand data, allowing to predict both peptide binding affinity and MHC antigen presentation likelihood with the performance of both prediction modes being boosted by the information leveraging between the two data types [61]. The output from the tool comprises raw prediction values and associated percentile rank scores. In the original article, NetMHCpan achieved a high performance when evaluated with epitopes and their source proteins. Benchmark studies have demonstrated that a percentage rank threshold of 0.5% “will identify ~70% of the epitopes while discarding up to 99.5% of non-immunogenic peptides” independent of the human leukocyte antigen (HLA) type [61, 64, 65]. Most neoepitope selections are thus conducted at this threshold (examples include [66, 67]). The method is available to download, and as a web server, at https://services.healthtech.dtu.dk/service.php?NetMHCpan-4.1.
MixMHCpred: Bassani-Sternberg et al. [62] developed MixMHCpred to identify MHC binding preferences from MS data. For this aim, the authors generated novel immunopeptidomic datasets and also collected publicly available data of this kind. Allele-specific position weight matrices (PWMs) were constructed and evaluated with cancer-derived neoantigens. The method is available to download at https://github.com/GfellerLab/MixMHCpred.
Other publicly available state-of-the-art tools within this field include MHCFlurry [53], and HLAthena [51] which are described in other sections of this paper.
Strategies to account for pathogen-similarity and self-similarity
Since self-reactive T cell clones are deleted at the thymus, neopeptides must be different to self-peptides to be recognized by circulating T cell clones. Since most neoantigens arise from mutated self-peptides, their sequences are more similar to the normal counterpart compared to pathogen-derived epitopes. Further, it is important to evaluate the similarity between the mutated peptide not only with the wild type counterpart, but also with the rest of the proteome. This is particularly important since T cell clones recognizing dissimilar peptides should escape from the central tolerance mechanism and thus be available to recognize them. On the contrary, cross-reactivity of T cells tolerant to neoepitopes similar to the wild-type peptides could trigger a harmful autoreactive immune response [68]. It has been further proposed that neoantigen similarity to pathogenic-derived epitopes could favor immunogenicity [69, 70]. Several methods have been proposed to assess these peptide similarity properties and some of them are described in Table 1.
Kernel: The Kernel metric is used to compare the degree of similarity between two peptides without performing a sequence alignment [71]. The method compares subsequences (or kmers) of all possible lengths within the peptides, which are weighted using a blocks of amino acid substitution matrix (BLOSUM). In the original article, the Kernel was evaluated with MHC ligands. This method was also applied by Bjerregaard et al. [65] to demonstrate that peptide similarity between mutant and wild-type peptides was a predictor for antigenicity in the cases where MHC binding potential was conserved between the two peptide variants.
Antigen.garnish: This method was designed to assess neoantigen quality towards likelihood of immunogenicity [72]. For this aim, Richman et al. [72] collected five clinical datasets from 318 cancer patients, and explored several quality metrics, such as pMHC binding affinity, differential agretopicity index (DAI, the ratio of the mutant peptide MHC binding to the non-mutated peptide MHC binding), similarity to known immunogenic epitopes, as well as the use of dissimilarity to the non-mutated proteome. Similarity and dissimilarity scores were calculated using Basic Local Alignment Search Tool (BLAST) over the human proteome and Immune Epitope Database (IEDB) derived epitopes respectively. The similarity metrics proposed in antigen.garnish demonstrated a good performance when evaluated with neoepitopes and non-immunogenic neopeptides, both being experimentally confirmed MHC ligands. Independent of the prediction of MHC binding, the authors found that the presence of neoantigens highly dissimilar to the human self proteome or enriched with hydrophobic amino acids, was associated with survival following anti-programmed cell death protein-1 (PD-1) checkpoint therapy in non-small cell lung cancer. This method is available at https://github.com/immune-health/antigen.garnish.
Pairwise sequence similarity: pTuneos [23] is a neoepitope prediction pipeline that incorporates pairwise sequence similarity to rank the best candidates, among other features. Its pairwise sequence similarity module uses the BLOSUM62 matrix to calculate a similarity score between paired tumors and normal peptides. Since these scores vary depending on the amino acid peptide composition, normalization is performed dividing the values derived from the comparison with BLOSUM62 by the similarity score of the neoantigen tested against itself. pTuneos and the code to execute the pairwise sequence similarity can be obtained from https://github.com/bm2-lab/pTuneos.
Prediction of pathogen-associated antigen immunogenicity
This section describes methods that predict peptide immunogenicity, but are not restricted to cancer antigens because the training was performed with epitopes from multiple sources, mostly viral pathogens. Based on peptide sequences, these methods are able to detect the binding preferences of TCRs to pathogen-associated pMHC. It could be hypothesized that these interaction rules are general and therefore, applicable to neoepitopes. Relevant examples of these tools are mentioned in Table 1.
IEDB Immunogenicity: Calis et al. [73] analyzed 2,509 peptides with predicted binding to MHC class I that were experimentally validated for T cell response. The dataset was obtained from IEDB [74] and complemented with peptides from Coxiella burnetii and Vaccinia virus. With the data, the authors validated that positions (P)4–6 within peptides as well as large aromatic side chains are associated with immunogenicity. Based on these findings, they developed a model by computing the sum of log enrichment amino acids in non-MHC anchor positions, weighted according to the importance of the position. This model was validated on Dengue-derived peptides tested for immune responses. IEDB Immunogenicity is one of the most used immunogenicity prediction models [32, 75, 76]. The method is available to download and as a web server at http://tools.iedb.org/immunogenicity/.
DeepNetBim: Yang et al. [77] combined MHC binding and T cell response of pMHC pairs to develop a convolutional neural network (CNN) based model. The binding (n = 88,913 pMHC) and immunogenicity (n = 24,193 pMHC) datasets used to train two separate models were obtained from IEDB [74]. The evaluation of the combined method was performed over a public neoepitope dataset from [78] and IEDB independent data. The method is available to download at https://github.com/Li-Lab-Proteomics/DeepNetBim.
DeepImmuno: Li et al. [79] developed a method to predict immunogenicity based on peptide sequence and MHC, but independent from predictions of MHC binding. To build the training dataset they retrieved ~9,000 peptides tested for immunogenicity from IEDB and used a beta-binomial probabilistic model to account for variable immunogenic potential of each peptide, which is determined by the number of subjects that developed an immune response against the given peptide. Afterwards, they developed a predictive model based on a CNN architecture, which was evaluated on experimentally tested immunogenic and non-immunogenic peptides from viral infections and cancer [80]. This model captures P4–6 as the most relevant positions within peptides for immunogenicity. The method is available as a web server at https://deepimmuno.research.cchmc.org and can be downloaded from https://github.com/frankligy/DeepImmuno.
Prediction of tumor-specific antigen immunogenicity
This section reviews tools that have been developed to detect tumor antigens (Table 1). Tumor antigens comprise neoantigens and tumor associated antigens (TAA) that are non-mutated but can be highly immunogenic due to their high or ectopic expression in tumors (i.e. melanocytic differentiation antigens and cancer testis) [81]. Since these tools are trained and/or validated with epitopes from cancer studies, they should be more accurate in defining specific rules derived from antitumoral immune responses. A critical limitation is the scarcity of this kind of data.
DeepHLApan: This tool was developed to detect neoantigens [82]. Wu et al. [82] combined the prediction of MHC binding and immunogenicity into a recurrent neural network (RNN) with an attention module. Datasets were retrieved from IEDB, comprising 327,178 ligands to train a MHC binding model and 32,785 peptides validated for T cell response to train an immunogenicity predictor [74]. MS independent datasets and neoantigen data from [78] were obtained for the evaluation of DeepHLApan performance. The method is available as a web server at http://biopharm.zju.edu.cn/deephlapan, as a docker container and downloadable repository at https://github.com/jiujiezz/deephlapan.
INeo-Epp: Wang et al. [83] collected multiple peptidic features associated with immunogenicity to train a random forest classifier. These features are the frequency and type of amino acids within the center of the peptide, peptide entropy, and predicted binding to MHC. The authors also included features related to the impact of a mutation, to develop a separated specialized neoantigen prediction model. The training data comprised 8,316 T cell validated peptides of any disease from IEDB. Neoantigen validation datasets were collected from several published independent studies [65], as well as viral derived antigens, consisting of 577 non-immunogenic peptides and 85 immunogenic epitopes. Both methods are available as web servers at http://www.biostatistics.online/ineo-epp/neoantigen.php.
TA predictor: Herrera-Bravo et al. [84] developed a tool to predict antitumor antigens using a quadratic discriminant analysis (QDA) algorithm. For this aim, immunogenic tumor peptides were collected from TANTIGEN database [85], and curated non-tumor peptides from IEDB. TANTIGEN not only contains neoantigens, but also shared non-mutated tumor antigens that are expressed in normal tissue, and are highly immunogenic. This data was splitted to train and evaluate the models, and multiple machine learning algorithms were tested. The final model relies on amino acid properties extracted from the AAindex database [86].
PRIME: Schmidt et al. [87] developed a predictor of neoepitope immunogenicity. To detect relevant positions within peptides, the authors analyzed ligands obtained with MS and selected positions within the center of the peptides for further analysis. The authors identified that tryptophan (W), phenylalanine (F), and tyrosine (Y) were enriched within this region in immunogenic peptides. Based on the pMHC predicted affinity with MixMHCpred [62] and the frequencies of the 20 amino acids at selected positions, the authors trained a logistic regression model. The training dataset of PRIME combined peptides experimentally tested for T cell response derived from pathogens or cancer testis antigens (n = 1,629), as well as cancer mutations (n = 3,329), and random negatives. The predictive performance of PRIME was assessed in a mouse-derived neopeptide dataset which was experimentally tested for immune response. Additionally, the authors observed that structural determinants of TCR recognition of pMHC are correlated with PRIME predictions. The method is available as a web server at http://prime.gfellerlab.org and as a downloadable code at https://github.com/GfellerLab/PRIME.
iTTCA-RF: Jiao et al. [88] developed a method for the identification of tumor T cell antigens based on a random forest algorithm. Tumor epitopes were obtained from TANTIGEN and TANTIGEN 2.0 [89] and non-tumor epitopes from IEDB, and this data was randomly separated to train and evaluate the models. The authors included features based on amino acid properties, specifically: the global protein sequence descriptor, the grouped amino acid and peptide composition, the adaptive skip dipeptide composition, and the pseudo-amino acid composition, to train the model. The method is available as a web server at http://lab.malab.cn/~acy/iTTCA/.
Evaluation of methods with an independent neopeptide dataset
To suitably evaluate the performance of the reviewed methods, an in-house dataset was arranged, consisting of 94 mutation-based neopeptides (26 immunogenic and 68 non-immunogenic), which were experimentally validated for T cell responses (Table S1, Supplementary materials). These neopeptides were originated in melanoma tumors from 3 patients that participated in the VACCIMEL trial [37] (Figure 2A). The candidates were selected primarily based on MHC binding predicted by NetMHCpan 4.0, with the use of MuPeXI. Neopeptides from patients #005 and #032 are novel and first described here, and neopeptides from patient #006 were previously published [17].
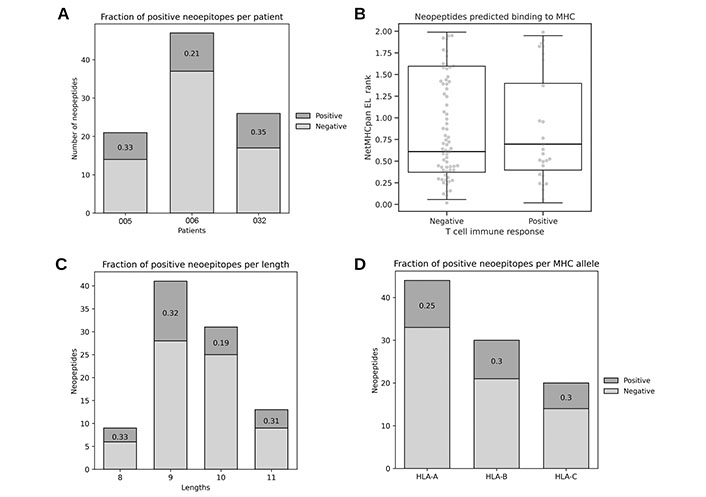
Characteristics of the in-house neoepitope dataset. (A) Immunogenic fraction of neoepitopes per patient; (B) predicted binding to corresponding patient’s MHC for immunogenic and non-immunogenic neopeptides (Mann Whitney U = 0.498, n.s.); (C) immunogenic fraction of neoepitopes per length; (D) immunogenic fraction of neoepitopes per MHC allele
As described earlier, the binding of the peptide to the MHC has been established as a necessary (but not sufficient) step to elicit an immune response, and for this reason it can be a confounding variable if trying to assess the performance of immunogenicity predictive methods. To avoid misinterpretation of the methods reviewed, the dataset therefore only included neopeptides with predicted binding to the corresponding patient’s MHC. Also it was validated that positive and negative neopeptides have comparable ranges of predicted binding to the cognate MHC using NetMHCpan EL 4.0 (Figure 2B). Given these observations and peptide selection bias, NetMHCpan EL 4.0 model was not included in this evaluation. Finally, neopeptides in the dataset have a length distribution expected for MHC class I natural presented ligands [34] (Figure 2C) and it was corroborated that the MHC allele locus did not influence immunogenicity in this dataset, since the fraction of positives is similar for each MHC class I locus (A, B, and C) (Figure 2D).
The amino acid composition of neopeptides has been previously associated with their immunogenicity [90]. For instance, IEDB immunogenicity [73] reported an enrichment of phenylalanine (F), isoleucine (I), and tryptophan (W) among viral epitopes, while PRIME [87] described an enrichment of tryptophan (W), phenylalanine (F), and tyrosine (Y) in viral and tumoral epitopes. Considering that multiple methods weigh the central positions within the peptide as determinants of the interaction with TCRs, the amino acid composition of neopeptides at these positions in the established in-house dataset was explored (Supplementary materials). The result of this analysis is shown in Figure 3A, and demonstrated that the neoepitopes of our dataset also showed an enrichment in phenylalanine (F) and tyrosine (Y). However, tryptophan (W) was found to be completely absent. It should be mentioned that leucine (L) was the most prevalent amino acid. To further explore this observation, the amino acid enrichment from large datasets of T cell tested peptides from viruses obtained from IEDB and neopeptides from Neoepitope Database (NEPdb) [91] and Cancer Epitope Database and Analysis Resource (CEDAR) [92] was compared. This analysis demonstrates that the enrichment of tryptophan (W) is present only in viral antigenic peptides and in general both groups have different preferences (Figure 3B and C).
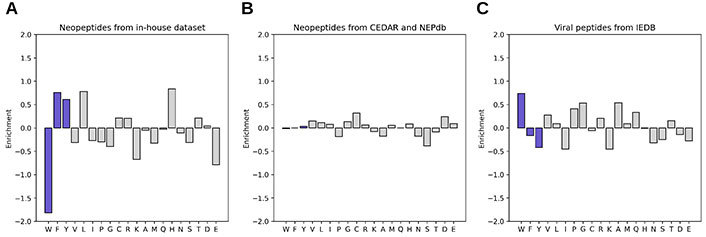
Amino acid enrichment in central positions of immunogenic (up) vs. non-immunogenic (down) peptides from different sources. The amino acids discussed are shown in blue. (A) Neopeptides from in-house dataset (immunogenic: 26, non-immunogenic: 68); (B) neopeptides from the CEDAR and NEPdb databases (immunogenic: 527, non-immunogenic: 2541); (C) viral peptides from the IEDB (immunogenic: 367, non-immunogenic: 7080)
NetMHCpanExp [57] allows the assessment of transcript expression values derived from the Human Protein Atlas (version 20.0) reference database. Since multiple methods reviewed in this work require expression values, and the obtention of RNAseq data was not possible for patient #032, this annotation was explored as a possible source of protein abundance information. By comparing it with the expression values of neopeptides source proteins from patients #005 and #006 obtained from RNAseq experiments, a strong positive correlation was found (Figure S1). Thus, these inferred values were used for further analysis for the three patients.
To avoid performance overestimation, it is essential that datasets destined to evaluate these methods are independent from the data used for training [93]. In this context, it was verified that the in-house neoepitope dataset has an overlap of identical peptides of 1% (1 of 96) with the training data of only DeepImmuno, DeepHLA, and NetHCpanExp and 4% with the training data of NetCleave. Excluding the overlapped peptides did not substantially change the performance of these methods (Table S2). Given this, it can be concluded that the reported performances of the individual methods are unbiased in terms of data redundancy between training and evaluation.
Next, the predictive performance of the reviewed methods (Table S1) was evaluated on the in-house dataset in terms of the area under the receiver operator curve (Table S3). This analysis describes how well the positives are separated from the negatives as their discrimination threshold varies, and allows the evaluation of predictions without a binary classification. An AUC of 1 describes a perfect classifier, and an AUC of 0.5 indicates there is no discrimination. The highest AUC obtained was 0.6, indicating a rather poor discrimination across all methods (Table 2). Among the best performing tools (AUC > 0.55), there are methods specifically developed to predict tumor antigens (category No. 1) and methods classified as predictors of biological features (category No. 4). Protein abundance (variant allele frequency, HLAthena), protein degradation (MHCflurry, HLAthena, ProteaSMM) and peptide association to TAP (NetCTLpan) are the features in this group that could contribute to immunogenicity prediction in this dataset.
AUC receiver operating characteristic (ROC) of best performing methods on in-house dataset
Category | Method | AUC ROC |
---|---|---|
i | MHCflurry processing | 0.609 |
iv | PRIME score | 0.604 |
i | Variant allele frequency | 0.6 |
iv | INeo-Epp neoantigen | 0.584 |
i | HLAthena MSiCE | 0.58 |
i | ProteaSMM c | 0.58 |
i | HLAthena MSiC | 0.576 |
i | MHCflurry PS | 0.571 |
i | NetCTLpan TAP | 0.568 |
i | ProteaSMM i | 0.561 |
N/A | MixMHCpred | 0.556 |
iv | TA predictor | 0.552 |
Tools are grouped by categories established in this article (i: biological features; iv: tumor immunogenicity)
The interferon gamma (IFNγ) enzyme-linked immunospot (ELISPOT) assays were used to assess the immune responses against our in-house neopeptide dataset. This technique quantifies the number of specific T cell clones recognizing a certain sample [94]. Considering the number of observed spots relative to the unspecific background, a quantitative value which reflects the strength of the immunogenicity was set (Supplementary materials). It can be hypothesized that some of the tools may better predict the most immunogenic neoepitopes, which are capable of eliciting the highest number of IFNγ producing cells. To identify such tools, the correlation between the quantitative values of ELISPOT and the estimations of reviewed methods was calculated. For the immunogenic neoepitopes, a positive correlation with predictions from NetMHCpan 4.0 and MHCflurry presentation was observed (Figure 4). With the entire dataset (which also contains non-immunogenic neopeptides), no significant association was found (Table S1). These results suggest that, although it is difficult to predict the presence or absence of an immune response, in cases where there is indeed an immune activation, it is possible to infer the intensity of that response from the predicted MHC presentation likelihood.
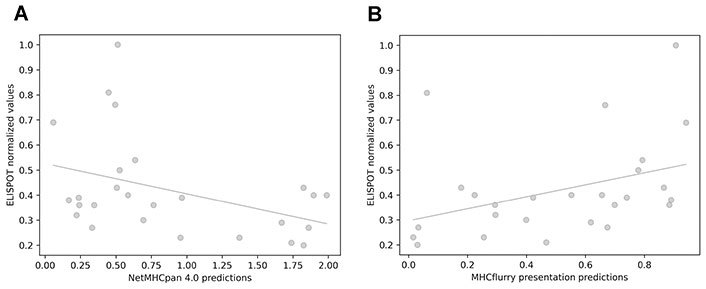
Correlation between immunogenicity values obtained from IFNγ ELISPOT assays and values obtained with predictive methods. (A) NetMHCpan 4.0 EL rank (Pearson’s correlation test, r = −0.399; Spearman’s correlation test, ρ = −0.31); (B) MHCflurry presentation score (Pearson’s correlation test, r = 0.38; Spearman’s correlation test, ρ = 0.47)
Discussion
This article has reviewed multiple bioinformatic and immunoinformatic tools proposed to contribute to the prediction of immunogenic neopeptide candidates, besides MHC binding. A common characteristic of these tools is that the sequence of the mutated peptide is the most relevant information considered. Other characteristics derived or complementary to peptide sequence are: i) peptide availability (e.g., processing, presentation, and abundance); ii) T cell availability (e.g., self-similarity and foreignness); iii, iv) TCR preferences [e.g., location and type (charge, size, etc.) of amino acids in mutated peptides].
To evaluate the methods reviewed here, a novel neoepitope dataset was assembled. In the evaluation, it was observed that most of the methods misclassify immunogenic and non-immunogenic neopeptides. The authors acknowledge that the small number of peptides in this dataset (especially those from the immunogenic fraction) may impose a limitation that could lead to underestimating the performance of the tools. Besides, an important factor that may explain these results is the rationale behind neoepitope selection. In biased datasets, composed of peptides preselected by some criteria (e.g., antigen presentation, peptide binding to MHC, and antigen expression), the specific feature used for selection will in general not show any predictive performance. In our in-house neoantigen dataset, the main selection criterion was the predicted binding to MHC by using the NetMHCpan 4.0 EL model. This imposes a bias towards not only NetMHCpan but also all models that directly or indirectly predict binding and antigen presentation by MHC.
Pathogen-associated epitope datasets are the most abundant among validated peptides for T cell immune response, and for this reason, methods specific to predict cancer antigens in general suffer from being trained on small datasets. Several tools described in this review have been trained with epitopes derived from pathogens (mostly viral). For instance, IEDB immunogenicity [73] and PRIME [87] are methods that rely on the amino acid composition of immunogenic peptides, and both were trained with pathogen-derived data (for PRIME this data was complemented by a minor proportion of neoepitopes). To test the hypothesis that T cell recognition rules are general, the preferences for neoepitopes and viral epitopes were analyzed. An enrichment in aromatic residues among our neoepitopes was observed, in line with what was reported by the authors [73, 87]. However, tryptophan (W) was found to be completely absent in our data, as well as in a large neoepitope dataset (combining CEDAR and NEPdb), although it was highly abundant in viral epitopes (IEDB). Also, it was observed that neoepitopes do not have a clear amino acid preference, compared to viral antigens. This fact may represent a limitation for how much information can be transferred into the field of neoantigen prediction. This is remarkable, since the models of both of these earlier tools have learned primarily this latter preference. In agreement with this concept, here it was observed that these types of methods in general failed to predict our dataset. It must be realized that viral antigens are generally presented in an inflammatory context, quite different from the cancer neopeptides presented in a mostly immunosuppressed tumor microenvironment (TME).
It was also asked whether any method would be able to predict the intensity of the immune response elicited by immunogenic neoantigens. Here, a positive correlation between quantitative ELISPOT and predictions associated with MHC binding was found for the immunogenic neopeptides. This observation indicates that the likelihood of binding to MHC is not only important to determine the peptide immunogenicity, but that binding can also play a role in defining the intensity of the T cell response. In addition, these results suggest that other than simply classifying peptides into immunogenic vs. non-immunogenic, it could be of value to work on quantitative data, allowing to rank peptides within different degrees of immunogenicity.
It is important to stress that the experimental validation of the neoantigens defined here was performed by testing IFNγ production after stimulation of the patient’s peripheral blood mononuclear cells (PBMCs) with synthetic candidate peptides. PBMCs provide a higher number of lymphocytes readily available, compared to TILs samples. Thus, since T cell clones recognizing neoantigens can be marginated from the periphery to infiltrate tumor lesions, it is possible that the lack of reactivity in ELISPOT assays performed with PBMC is underestimating neoantigen immunogenicity. Other studies have successfully performed neoantigen identification using TILs in in vitro assays [95, 96].
In our opinion, more experimentally validated neoantigens are awaited to train and refine neoepitope predictors. As said, the limited data available is likely to have biases that confuse machine learning algorithms. Having a large volume of data may allow the models to learn the general rules and discriminate the biases that some subsets of data could have. On the other hand, benchmark studies like this one, must be performed with data different from the one used to develop the methods. This imposes another level of difficulty in finding and generating appropriate datasets. These facts highlight the great value of making publicly available neoantigen data in order to improve the tools that make possible the development of more specific and efficient therapeutic strategies.
It should be noted that only a small portion of the many candidate neoantigens are the targets of immune responses, and this might be related to the phenomenon of immunodominance and immune ignorance. Linette et al. [97] demonstrated that, despite the expression of neoantigens in multiple tumors of melanoma patients, spontaneous neoantigen-specific T cells were absent or limited to a very low frequency. In contrast, upon vaccination with a mature-dendritic cells (DCs) vaccine transfected with neoantigens and gp100 peptides, a higher and more diverse neoantigen-specific CD8+ T cell repertoire was detectable in TILs and PBMC. In accordance with these results, Zeng et al. [98] reported that an immune response to predicted neoepitopes of collecting duct carcinoma, a rare tumor with low TMB, could be detected only after vaccination with a peptidic vaccine. Thus, well predicted neoantigen candidates can be experimentally assigned as non-immunogenic when in fact, representing false negatives due to immune ignorance. Similarly, comparing post-vaccination to pre-vaccination T cell samples, we observed a great increase in the immune response against shared non-mutated melanoma associated antigens that were highly expressed in our vaccine VACCIMEL, as well as to private neoantigens [17], supporting the antigen ignorance hypothesis. This evidence highlights the limited diversity of neoantigen-specific natural T cell responses observed in cancer metastases [99]. This suggests that the majority of T cells are spontaneously ignorant of most neoantigens until specific vaccination may facilitate neoantigen cross-presentation and/or the administration of anti-immune checkpoint therapies increase the activity of T cells.
The immunodominance and immune ignorance of neoantigens cannot be predicted yet. In this sense, it is well known that the same immunogenic viral peptides can elicit or not a specific immune response in different patients sharing the epitope HLA restriction element [100], reflecting other causes beyond the immune features of a given epitope. In the context of cancer neoepitope, this is highly relevant since the immune response against neoantigens in general can not be assessed in multiple patients due to their private nature. Thus, this fact poses an intrinsic limitation to epitope prediction that cannot be anticipated. The presence of false negatives in neoepitope datasets may be one of the main confounding factors in neoantigen prediction algorithms that are trained with these data, and one of the main causes of the generally low performance that we observed when predicting immunogenicity of cancer neopeptides.
Given that the immune synapse between the pMHC and TCR is the center of T cell activation, many authors propose the integration of TCR recognition prediction into pipelines of neoepitope selection, considering that the combination of pMHC-TCR interaction and immunogenicity predictions may improve the efficacy of immunotherapies, such as tumor antigen-specific TCR-T cell therapies. Accurately performing this task represents a computational and experimental major challenge. It has been stated that TCR paired α and β chains are required to accurately predict T cell targets [101]. To obtain this information, single cell TCR sequencing should be performed, but this technique can only screen a limited amount of T cells, having low chances to recover low frequency clones [102]. For this reason, TIL samples would be more desirable for this analysis compared to PBMC although they are not always available. In this sense, the scientific community is currently introducing new tools that predict the interaction of pMHC-TCR [103–105], and it is expected that the accuracy and coverage of these methods will increase, as high-quality and quantity data becomes available. We envision that such methods combined with a deep characterization of the TCR repertoire will allow us to resolve, at least in part, the phenomenon of immune-ignorance and dominance.
Additionally, there is still limited knowledge about the multiple factors associated with the efficiency of the complex immune response elicited against neoantigens. Indeed, even when the best neoantigens can be predicted, different characteristics of each patient can determine the fate of the immune response. Immune escape mechanisms adopted by tumors, such as downregulation of MHC expression at the surface of cancer cells [106], tumor editing to eliminate cells that express neoantigens [33], expression of ligands that induce T cell exhaustion, namely PD-L1 and PD-L2 [107, 108], and the production of immunosuppressive cytokines which modulate the composition and phenotype of the immune infiltrate that can penetrate the tumor [109], will affect the cytotoxicity against cancer cells. Several of these factors can be influenced by the genetic background of the patients, as in the case of polymorphisms of genes that determine the expression levels of cytokines [110, 111] and MHC homozygosity [112], among others.
Conclusions
Over the last years, much progress has been made in the selection of tumor neoepitopes that have clinical applications such as the development of personalized therapies. This was made possible by two major technological developments: i) next generation sequencing (NGS) to obtain tumor sequences in reasonable short time and low cost and ii) improvements in bioinformatic and immunoinformatic algorithms to obtain highly accurate variant calls and predictions of neopeptides binding to MHC. Although very powerful, the combination of these two technologies still yields a large number of neoepitope candidates that are very expensive and laborious to test. The present work evaluated tools that could refine the selection of these candidates, and the results indicate that there is still work ahead to accurately achieve this purpose. Mutated peptide sequences indeed contain relevant information, but it is not enough to accurately predict its immunogenicity. In our opinion, the lack of neoantigen data is a major challenge. Also, there is still a need to integrate the complexity of the immune response in cancer patients, in particular, the generation of T cell repertoires capable of recognizing neoepitopes. Solving this issue will require a technological improvement of great magnitude such as the striking development of NGS and bioinformatics, which is expected to be developed in the years to come. Finally, the phenomenon of immunological ignorance, which is partially determined by patient-specific factors, causes good neoepitope candidates (in terms of the features reviewed in this article) to be detected as negatives in in vitro assays. This imposes an intrinsic limitation on the prediction of neoantigens, which at the moment remains to be solved.
Abbreviations
AUC: | area under the curve |
BLOSUM: | blocks of amino acid substitution matrix |
CEDAR: | Cancer Epitope Database and Analysis Resource |
ELISPOT: | enzyme-linked immunospot |
IEDB: | Immune Epitope Database |
IFNγ: | interferon gamma |
INeo-Epp: | immunogenic epitope/neoepitope prediction |
iPCPS: | improved proteasome cleavage prediction server |
iTTCA-RF: | Identification of Tumor T cell Antigens-Random Forest |
MHC: | major histocompatibility complex |
MS: | mass spectrometry |
NEPdb: | Neoepitope Database |
PBMCs: | peripheral blood mononuclear cells |
pMHC: | peptide-major histocompatibility complex |
PRIME: | Predictor of Immunogenic Epitopes |
RNA-Seq: | RNA sequencing |
TA predictor: | tumor antigen predictor |
TAP: | transporter associated with antigen-processing |
TCR: | T cell receptor |
TILs: | tumor infiltrating lymphocytes |
WES: | whole-exome sequencing |
Supplementary materials
The supplementary Figure, Tables, and Supplementary methods in Supplementary materials for this article are available at: https://www.explorationpub.com/uploads/Article/file/100391_sup_1.xlsx and https://www.explorationpub.com/uploads/Article/file/100391_sup_2.pdf.
Declarations
Acknowledgments
We dedicate this work to our patients. This work has been performed using the Danish National Life Science Supercomputing Center, Computerome. We thank Emilio Fenoy for insightful discussions about this research.
Author contributions
IC, MN, and MMB: Conceptualization, Writing—original draft. IC: Formal analysis. IC, ES, EP, and HMGA: Investigation. IC and HMGA: Software. IC: Visualization. JM, MN, and MMB: Funding acquisition, Resources. MMB: Supervision. IC, ES, EP, HMGA, JM, MN, and MMB: Writing—review & editing. All authors read and approved the submitted version.
Conflicts of interest
The authors declare that they have no conflicts of interest.
Ethical approval
The CASVAC-0401 study was carried out after approval of the Ethics Committee of the Instituto Alexander Fleming. The study was also approved by the Argentine Regulatory Agency (ANMAT, Disposition 1299/09).
Consent to participate
Informed written consent to participate in the CASVAC study and for the use of their samples in the research projects associated with the vaccination protocol was obtained from all participants.
Consent to publication
Not applicable.
Availability of data and materials
The dataset generated for this study is included in the supplementary files.
Funding
This work was supported by grants from CONICET, Agencia Nacional de Promoción Científica y Tecnológica (ANPCyT), Instituto Nacional del Cáncer—Ministerio de Salud de la Nación Argentina (INC-MSal), Fundación Sales, Fundación Cáncer, and Fundación Pedro F. Mosoteguy, Argentina. The CASVAC-0401 Phase II clinical study (Clinical Trials.gov, NCT 01729663) was sponsored by Laboratorio Pablo Cassará S.R.L. The funders had no role in study design, data collection and analysis, decision to publish, or preparation of the manuscript.
Copyright
© The Author(s) 2023.