Abstract
Aim:
Smartphone technology is increasingly used by the public to assess sleep. Specific features of some sleep-tracking applications are comparable to actigraphy in objectively monitoring sleep. The clinical utility of smartphone apps should be investigated further to increase access to convenient means of monitoring sleep.
Methods:
Smartphone and subjective sleep measures were administered to 29 community-dwelling healthy adults [aged 20–67, Mean (M) = 26.8; 18 women, 11 men], and actigraphy to 19 of them. Total sleep time (TST) and sleep efficiency were measured with actigraphy and the Sleep Time App (Azumio Inc.). Sleep diaries captured subjective TST and sleep efficiency, and the Epworth Sleepiness Scale and Pittsburgh Sleep Quality Index provided self-report data. An exit questionnaire was administered to examine App feasibility and likelihood of future use.
Results:
The App significantly overestimated TST when compared to actigraphy. There was no significant difference in sleep efficiency between methodologies. There was also no significant difference between TST recorded through the App and through sleep diaries. Participants’ self-reported ease of use of the smartphone App positively correlated with likelihood of future use.
Conclusions:
Based on the current findings, future research is needed to investigate the utility and feasibility of multiple smartphone applications in monitoring sleep in healthy and clinical populations.
Keywords
Sleep-tracking, smartphone, technology, actigraphy, wearables, MhealthIntroduction
The National Heart, Lung, and Blood Institute (2012) states that sleep duration and sleep quality protect and improve physical and mental health, safety, and overall quality of life [1]. It is therefore of concern that sleep disorders are prevalent across the world [2]. Adults often report difficulties with daytime functioning due to sleep disturbances [3], such as sleepiness during driving. Deleterious health outcomes such as diabetes and obesity are also associated with sleep quality and duration [4]. Without proper sleep, the likelihood of daytime drowsiness, adverse cognitive and motor effects [5, 6] and falling asleep at the wheel are markedly increased [7–9]. Difficulty with emotion regulation and exacerbation of depression and anxiety can also result from inadequate sleep and lack of self-monitoring [5, 10–12], ultimately reducing quality of life [13].
Monitoring sleep could become an integral element of medical treatment and improvement of daily functioning. A number of sleep assessment methods have been developed, such as paper sleep diaries regularly utilized in clinical practice to subjectively measure sleep patterns [14]. The standard methods to objectively measure sleep variables such as total sleep time (TST, total number of undisturbed minutes that a person is asleep), sleep efficiency (percent of time asleep without fragmentation or restlessness), and stages of sleep began with polysomnography (PSG) throughout the 1960s and 1970s [15], actigraphy in 1988 for the United States military [16], and finally the integration of accelerometer technology in smartphones during recent years.
Self-report diaries are limited in utility as they are often filled out weekly rather than nightly or are often lost, destroyed, or difficult to read [14]. Several studies comparing the efficacious metrics of PSG and actigraphy (as well as wrist-worn electronic sleep diaries) to paper sleep diaries and self-report sleep questionnaires, found that objective measurements of TST were generally shorter in duration than those recorded subjectively [14, 17, 18]. Others have found that subjectively recorded TST is generally overestimated within clinical populations such as those with insomnia [19]. These findings emphasize a need for valid and reliable objective measures of sleep that can be implemented in the home.
Today, PSG continues to be the gold standard for objective measurement of sleep. PSG consists of continuous monitoring of variables of neurophysiology and cardio respiration via electrodes that are connected to the body. PSG sleep studies are often conducted over the span of one night to monitor both disturbed and normal sleep in the context of obstructive sleep apnea and other sleep-related breathing disorders [20]; however, there are constraints on the wide use of PSG given the question of whether laboratory settings can impact the validity of study results, as well as the expense and impracticality of the method for research and clinical purposes [2, 21].
Actigraphy bracelets have been valuable in calculating both TST and sleep efficiency [22], having been employed by researchers to measure sleep for decades [23, 24]. They are constructed with an accelerometer that can reliably translate physical motion and exerted energy into a numeric representation measured in 30 or 60-second epochs [25, 26] and are often waterproof, allowing continuous data collection over days or weeks. Data collected via actigraphy technology have been found comparable to those collected via PSG in both young and older adults [27, 28].
Today, there are a variety of smartphone Apps and wearable technologies that are used to monitor diet, fitness, and sleep, setting in motion a new frontline of clinical practice and research [29]. Smartphone Apps that monitor and evaluate sleep duration and quality through accelerometer technology are increasingly marketed, though their clinical utility has yet to be investigated across various methodologies. Some research has compared the use of specific smartphone applications to measure sleep with PSG, and several studies have found differences in sleep measurements [30–32]. For example, Bhat and colleagues [30] found that data collected through the Sleep Time smartphone App [33], when compared to PSG, showed weak correlations between sleep efficiency (r = –0.127, P = 0.592), sleep latency (Pearson’s r = 0.384, P = 0.094), and light sleep/deep sleep percentages (r = 0.024, P = 0.921; r = 0.181, P = 0.444). To date there has been less work comparing smartphone and actigraphy methods. Natale and colleagues [2] compared raw sleep data collected directly from iPhone accelerometers (tri-axial accelerometer LIS302DL) to data collected via actigraphy, finding overall agreement in measurements of TST, wake after sleep onset, and sleep efficiency. It remains to be seen if smartphone applications that use proprietary metrics to convert accelerometer data into sleep metrics are reliable and valid. If validated through comparison to actigraphy, Apps such as Sleep Time could be a cost-efficient and innovative alternative to the expensive and invasive use of overnight PSG monitoring or the use of clinical devices such as actigraphy. Both healthy and clinical populations could benefit from such sleep tracking alternatives, in light of the ubiquity of smartphones.
The goal of the current project was to compare objectively collected data from the Sleep Time App to data recorded through actigraphy bracelets, and to self-reported sleep diary data. We hypothesized that 1) consistent with prior studies using actigraphy, self-reported TST obtained through sleep diaries would differ from TST collected by the App, and 2) there would be a significant difference between means of TST and sleep efficiency recorded by the App and actigraphy, because App use requires the smartphone face-down on the mattress near one’s head (i.e. mattress type, bed partners, and pets have the potential to affect sleep measurement) whereas actigraphy is a wearable technology that is continuously worn on the wrist each day. To investigate feasibility and acceptability of the App, we also conducted a Pearson’s correlation analysis, examining potential relationships between ease of App use and likelihood of future use.
Materials and methods
Subjective and objective data were collected from 29 healthy adult volunteers [18 females; age range 20–67, M = 26.8, standard deviation (SD) = 11.7]. The project was publicized through word-of-mouth and social media; participants were recruited from the community. Inclusion criteria specified that participants be free of acute or chronic medical conditions and sleep disorders and that they were not taking any prescription or over-the-counter sleep medications. Participants were also required to have access to an iPhone with IOS 8.0 or later for the daily use of the Sleep Time App (a free download).
This study was approved by the Institutional Review Board (IRB) of Boston University. During the first study visit, participants provided written informed consent. They were given a paper sleep diary from the National Sleep Foundation and downloaded the Sleep Time App on their smartphone device. Participants were also instructed to wear an actigraphy bracelet for one week. Due to battery malfunctions with the first set of actigraphy bracelets distributed, sleep data were obtained from a subset of the total sample (19 total participants). All 29 participants returned for a second study visit after one week, when three self-report questionnaires were administered. Among the three questionnaires was an exit questionnaire that assessed App feasibility and likelihood of individual use in the future.
Self-report questionnaires
Epworth Sleepiness Scale (ESS). The ESS [34] is a questionnaire that has a rating scale of 0 (“would never doze”) to 3 (“high chance of dozing”) across eight situations of everyday life, such as reading, watching TV, or being in a car during traffic. The points are totaled to calculate a total raw score with a higher score indicating higher likelihood of dozing, and if high enough, indicating the need to see a health professional about their sleep habits.
Pittsburgh Sleep Quality Index (PSQI). The PSQI [35] is a 19-item self-report questionnaire about sleep quality over the course of the past month. The global score range is 0–21 with a higher score indicating poorer sleep quality.
Objective measures of sleep
ActiGraph GT9X Link by Actigraph. For one week, 19 participants wore the Actigraph GT9X Link watch on their non-dominant wrist. Movement during wake and sleep time was recorded through accelerometer technology for 24 hours a day. The dependent measures examined were averages of each participant’s seven days of calculated TST (in minutes) and sleep efficiency (percentage).
Sleep Time smartphone application. The current free version of the Sleep Time App [33] is 2.21 (updated 02/27/2019). TST and sleep efficiency were monitored and recorded; daily TST minutes and sleep efficiency percentages were averaged for each participant.
Subjective measures of sleep
National Sleep Foundation sleep diary [36]. Sleep diaries were administered to all participants to fill out each morning and night for seven days to account for actigraphy and App malfunctioning and noncompliance. Self-reported TST and sleep quality were also compared with objective measures of sleep.
Ease of use and likelihood of future use
Exit questionnaire. Participants filled out an exit questionnaire during their second study visit that asked about personal lifestyle factors such as the type of mattress used and whether they sleep with pets in their beds. Participants were asked to rate the App’s ease of use as well as likelihood of future use. Analyses were conducted to examine whether ease of use correlated with likelihood of future use. The effect of age on ease of use was also investigated.
Data analysis
TST and sleep efficiency data were extracted from the actigraphy watches and the Sleep Time App and each participant’s seven days of data were averaged for each methodology. Paired samples t-tests were performed, and effect sizes were calculated to determine significant differences between TST and sleep efficiency as recorded through actigraphy and the smartphone application. Pearson correlations were conducted to identify potential relations between App feasibility and likelihood of future use.
Results
There was no significant difference between TST recorded by the App (M = 451.6, SD = 70.07) and TST recorded in the sleep diaries (M = 441.9, SD = 75.67); however, it is notable that this could be a result of participants recording App data into their sleep diaries each day, despite being instructed not to (see limitations).
TST (in minutes) as measured by the smartphone App (M = 444.3, SD = 44.52) was significantly longer in duration than that measured by actigraphy (M = 341.0, SD = 57.3; t[18] = 6.6, P = 0.01; Figure 1). Sleep efficiency (percentage) as measured by the App (M = 83.1, SD = 4.93) was not significantly different from sleep efficiency as measured by actigraphy (M = 78.8, SD = 6.9; t[18] = 2.06, P = 0.06; Figure 2). TST was overestimated 100% of the time (seven days) for 13/19 participants. For the remaining participants, the App overestimated TST 20–86% of the time (Table 1). On average, the App overestimated TST by 117 minutes (range 80–148, SD = 21.0) per day (across all participants). Further, we calculated overestimation averages of TST for each participant (Table 2) and amount of discrepancy in daily TST calculations between the App and actigraphy for one representative participant (Figure 3a).
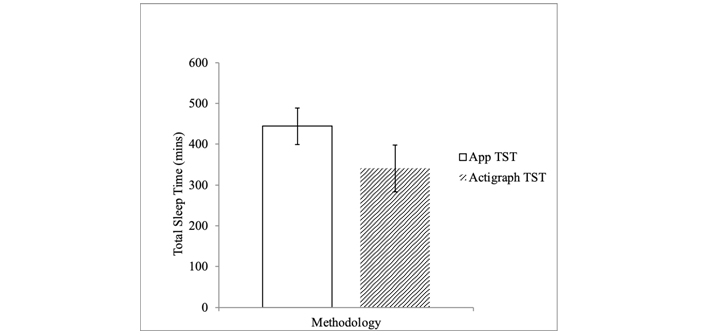
App vs. actigraph. Graph comparing TST in minutes as measured by the Sleep Time App (M = 444.3, SD = 44.52) to TST measured by actigraphy (M = 341.0, SD = 57.30)
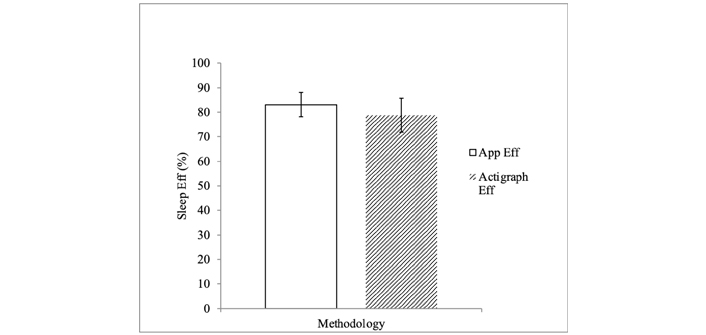
App vs. actigraph. Sleep Efficiency (Eff) Graph comparing sleep efficiency as measured by the Sleep Time App (M = 83.1, SD = 4.93) to sleep efficiency as measured by the actigraph (M = 78.8, SD = 6.90)
Percentage of time App overestimated TST and sleep efficiency
Participant ID | TST (%) | Sleep efficiency (%) |
---|---|---|
1 | 100 | 50 |
2 | 86 | 14 |
3 | 83 | 17 |
4 | 100 | 100 |
5 | 100 | 100 |
6 | 100 | 100 |
7 | 100 | 80 |
8 | 75 | 25 |
9 | 75 | 50 |
10 | 80 | 80 |
11 | 100 | 100 |
12 | 100 | 25 |
13 | 100 | 57 |
14 | 100 | 60 |
15 | 20 | 40 |
16 | 100 | 80 |
17 | 100 | 100 |
18 | 100 | 60 |
19 | 100 | 43 |
Overestimation averages of TST (minutes) and sleep efficiency (%) by participant
Participant ID | TST (minutes) | Sleep efficiency (%) |
---|---|---|
1 | 132.0 | 6.0 |
2 | 77.4 | 6.9 |
3 | 63.3 | 13.8 |
4 | 244.3 | 17.6 |
5 | 160.0 | 15.5 |
6 | 185.1 | 17.3 |
7 | 129.0 | 8.4 |
8 | 79.3 | 6.8 |
9 | 90.8 | 15.0 |
10 | 25.6 | 4.6 |
11 | 141.4 | 15.6 |
12 | 102.0 | 7.0 |
13 | 94.3 | 7.6 |
14 | 89.0 | 10.8 |
15 | 113.8 | 12.4 |
16 | 295.8 | 22.4 |
17 | 158.8 | 10.0 |
18 | 81.4 | 7.2 |
19 | 64.7 | 5.4 |
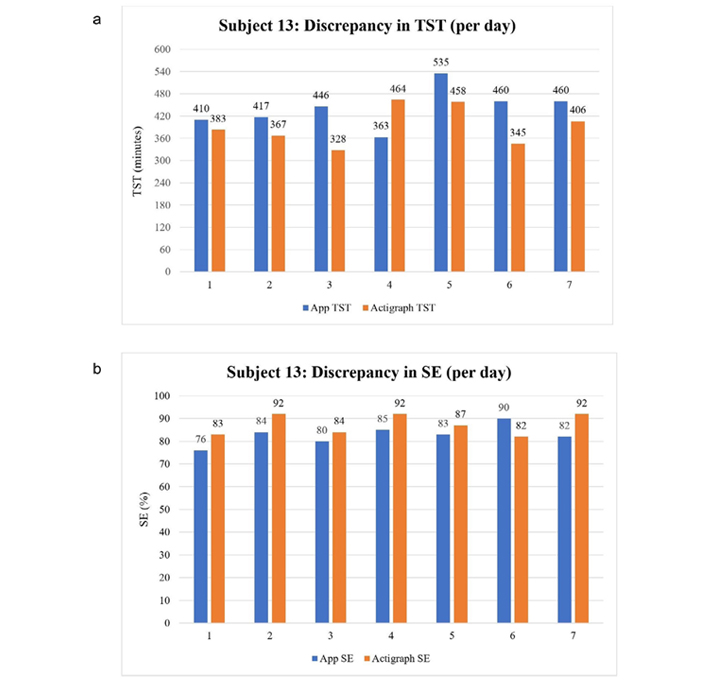
Subject 13: Sleep daily discrepancy calculations between App and actigraph in one representative participant. (a) Discrepancy in TST (per day) Graph depicting daily discrepancy in TST calculations; (b) discrepancy in SE (per day) Graph depicting daily discrepancy in sleep efficiency calculations
There was a non-significant trend for sleep efficiency as measured by the App (M = 83.1, SD = 4.93) to be higher than that found by actigraphy (M = 78.8, SD = 6.9; t[18] = 2.06, P = 0.06, Figure 2). On average, the App overestimated sleep efficiency by about 11% each day (range 4–22%, SD = 5.08, across all participants). We additionally calculated overestimation averages of sleep efficiency for each participant (Table 2), and amount of discrepancy in daily sleep efficiency calculations between the two methodologies for one representative participant (Figure 3b).
Sleep efficiency was overestimated 100% of the time for 5/19 participants, 80% of the time for 3 participants, and 14–60% of the time for the remaining 11 participants.
Feasibility
Participants’ self-reported ease of use of the smartphone App positively correlated with their likelihood of future use, Pearson’s r(27) = 0.47, P = 0.01. There were no other significant correlations between ease of use, likelihood of future use, or age (all P-values > 0.83).
Discussion
This study investigated the utility of a popular smartphone application, Sleep Time, in monitoring sleep in healthy adults by comparing it to sleep monitored through actigraphy technology.
There were no significant differences between subjective data recorded in sleep diaries and objective sleep data collected by the App. If participants did not write their daily App data into their sleep diaries as instructed, this finding suggests that the App, when compared to subjectively recorded TST, is a comparable predictor of TST in a healthy adult sample, at least in the age group reported here. Given these findings, the Sleep Time App could be a useful daily tool for tracking and recording one’s approximate TST, if preferred over written sleep diaries.
Like prior research comparing smartphone Apps to PSG [30–32], we found significant discrepancies between TST and sleep efficiency data collected through actigraphy and through the App. For example, Natale et al. [30] found weak correlations between the Sleep Time App and PSG while Patel et al. [31] did not find any correlations between TST measured through PSG and through another smartphone sleep App. Building upon previous studies that employed PSG, the present study tested the Sleep Time App by using it to monitor TST and sleep efficiency and comparing its data to data collected through actigraphy.
Although the App overestimated sleep in the healthy adults in the current study, relative to actigraphy, clinicians may still find utility in implementing such applications with clinical populations, particularly when other avenues including PSG and actigraphy are unavailable. Future research exploring this technology in populations with sleep disorders will provide additional information to clinicians.
We also examined whether there were relations between App feasibility and likelihood of future use, finding that ease of use positively correlated with likelihood of future use. This finding can inform both App developers and future research in this area as it is supportive of creating a simple and easy way to track one’s sleep habits and characteristics, and might be a desirable alternative to filling out paper sleep diaries or using actigraphy technology that does not allow for immediate viewable sleep data.
There were several limitations to the present study, including the small sample size and limited range of age, race, sex, and sleep habits. The primary technological limitation, which affected sample size, arose when the initial set of actigraphy bracelets distributed to participants had limited battery capacity, which was unknown to the researchers, and therefore were not collecting valid data. This model of actigraphy watch required being recharged by the company and we employed a more efficient strategy of using a newer model of actigraphy for the remainder of the participants and study. Methodological limitations include the possibility that although instructed not to, some participants may have filled out their paper sleep diaries according to what the Sleep Time App calculated. It should also be noted that our sleep diary data were limited in a way that prevented us from measuring subjective sleep efficiency. Furthermore, because the App captures movement through accelerometer technology and consequently, the smartphone must be placed face down on the mattress near one’s head to measure movement, it is notable that type of mattress (e.g., spring, memory foam and gel) may influence data accuracy. Similarly, having a partner or pet sleeping in the same bed could also influence the movement data recorded through the accelerometer; in our sample, however, these factors did not significantly relate with TST or sleep efficiency. Actigraphy technology likewise faces barriers to data accuracy in clinical populations such as those with restless leg syndrome, who show excessive movement, or those with insomnia, who are awake for several hours of the night but who remain motionless in bed and are apparently ‘asleep’. Taken together, it is evident that follow-up studies are needed, and researchers should continue to investigate the utility of commercial sleep tracking devices in larger, more diverse samples, and in controlled environments.
Our findings indicate that, while potentially appealing to some users, smartphone application technology (at least, the App assessed here) does not currently provide a metric of at least one common sleep variable, TST, in healthy adults that is comparable to clinically validated devices. Once additional studies are conducted in controlled settings and with larger and more diverse samples, sleep professionals may be able to reliably use data recorded through the App. It would also be advantageous to examine the App’s utility within clinical populations and across other models of smartphones. Objective measurement of sleep using other wearable movement and sleep-tracking devices should be further explored so that cost-efficient and expedient means of monitoring sleep in both research and clinical settings can be available. Validating and implementing affordable devices or applications that track one’s sleep habits and patterns could create an easy way to promote sleep hygiene. In turn, this can improve overall health throughout the lifespan.
Currently, there is insufficient evidence to support that commercial measures of objective sleep (such as Apps and smartwatches) possess the validated utility for application in clinical settings [37]. Although mobile health (Mhealth) companies do not market their mobile applications or smartwatches as clinical tools, meaning that they should not be used to self-diagnose sleep disorders, mobile sleep-tracker Apps have become very popular and widely used. It is possible that both consumers and clinicians could find value in using these products to monitor sleep habits and identify behavioral patterns while being aware of their limitations. Future directions in smartphone and wearable, sleep-tracking technology should include adopting standard metrics for validation of these devices in order to inform both clinical researchers and companies interested in marketing their devices for research and clinical applications. To that end, as articulated by De Zambotti et al. [38], there is a pressing need for active communication between the sleep research community and companies that market wearable technology, so that marketing output of these devices can be optimized to provide cost-efficient options for researchers, clinicians, and users to assess sleep.
Abbreviations
M: | mean |
PSG: | polysomnography |
SD: | standard deviation |
TST: | total sleep time |
Declarations
Acknowledgments
The authors would like to acknowledge and thank the Boston University’s Vision and Cognition Laboratory for helping with some of the research process such as providing the actigraph technology.
Author contributions
TM and EA contributed to study design and implementation, database organization and assessment, and data analysis. TM wrote the first draft of the manuscript. EA, SN and ACG provided confirmation and advice on data analysis, interpretation, and manuscript revision.
Conflicts of interest
The authors declare that they have no conflicts of interest.
Ethical approval
Not applicable.
Consent to participate
Informed consent to participate in the study was obtained from all participants.
Consent to publication
Not applicable.
Availability of data and materials
Not applicable.
Funding
This work was supported by the Bridgewater State University Adrian Tinsley Program Summer Research Grant. The funding source had no influence on study design or decision to submit for publication or presentation at professional meetings.
Copyright
© The Author(s) 2021.