Abstract
Aim:
Popularity of electronic cigarettes (i.e. e-cigarettes) is soaring in Canada. Understanding person-level correlates of current e-cigarette use (vaping) is crucial to guide tobacco policy, but prior studies have not fully identified these correlates due to model overfitting caused by multicollinearity. This study addressed this issue by using classification tree, a machine learning algorithm.
Methods:
This population-based cross-sectional study used the Canadian Tobacco, Alcohol, and Drugs Survey (CTADS) from 2017 that targeted residents aged 15 or older. Forty-six person-level characteristics were first screened in a logistic mixed-effects regression procedure for their strength in predicting vaper type (current vs. former vaper) among people who reported to have ever vaped. A 9:1 ratio was used to randomly split the data into a training set and a validation set. A classification tree model was developed using the cross-validation method on the training set using the selected predictors and assessed on the validation set using sensitivity, specificity and accuracy.
Results:
Of the 3,059 people with an experience of vaping, the average age was 24.4 years (standard deviation = 11.0), with 41.9% of them being female and 8.5% of them being aboriginal. There were 556 (18.2%) current vapers. The classification tree model performed relatively well and suggested attraction to e-cigarette flavors was the most important correlate of current vaping, followed by young age (< 18) and believing vaping to be less harmful to oneself than cigarette smoking.
Conclusions:
People who vape due to flavors are associated with very high risk of becoming current vapers. The findings of this study provide evidence that supports the ongoing ban on flavored vaping products in the US and suggests a similar regulatory intervention may be effective in Canada.
Keywords
Electronic cigarettes, vaping, machine learning, classification treeIntroduction
Canada and the US have recently witnessed exponential growth of e-cigarette use (vaping), raising worldwide public health concern of a new nicotine epidemic [1, 2]. While some studies have shown the benefit of vaping in assisting with smoking cessation [3–5], evidence has also directly linked vaping to health conditions including respiratory irritations [6] and lung damage [7]. Indeed, the US Centers for Disease Control and Prevention reports 2,807 cases of vaping-related lung injury, including 68 deaths, as of February 2020 [8]. In September 2019, Canada confirmed its first case of severe pulmonary illness related to vaping, which involved a high school student [9].
The majority of people who have tried vaping do not continue to use the device in the long run [10, 11]. It is therefore crucial to identify the small group of users who are likely to become long-term vapers as this may indicate vaping dependency that could lead to chronic health effects. Prior studies have suggested a set of characteristics that may be unique to current vapers, including female, younger age, use of a certain type of vaping device as well as initiating vaping due to attraction to flavors and lower cost [11–15]. However, these results were yielded primarily by regression where multicollinearity is a concern. As person-level variables are usually correlated, e.g., younger people are easily attracted to e-cigarette flavors and are more likely to perceive vaping to have lower risks [16], it is difficult to isolate a set of independent predictors of current vaping using just regression. Hence, this issue warrants the use of more advanced statistical techniques.
Identifying current vapers from people with an experience of vaping represents a supervised binary classification task in machine learning, a discipline of computer science with increasing popularity in health research [17–19]. Compared with conventional regression, machine learning leverages computational power to reduce multicollinearity and improve the overall model performance. Applications of machine learning in tobacco research are emerging in recent years [20–25], but so far, only one such application has been on vaping behaviours. In this Holland-based study, a random forest model was used in conjunction with cross-sectional survey data to classify adult exclusive vapers from dual users of both cigarettes and e-cigarettes [25]. Here we present a simpler and more intuitive machine learning model-a classification tree-to identify and understand the importance of person-level correlates of current vaping. In other fields of tobacco research, classification trees have demonstrated good performance in predicting the status of lab-verified smoking cessation status [20], adherence to nicotine replacement therapy [23] and use of tobacco within 30-min of waking up [21]. Hence, we aimed to verify the performance of classification tree in vaping research and to provide actionable implications on policy interventions regarding e-cigarettes in a Canadian context.
Materials and methods
Study design and sample
This population-based cross-sectional study used data from the 2017 Canadian Tobacco, Alcohol, and Drugs Survey (CTADS) that included 16,349 Canadian residents aged 15+ (excluding institutional residents) from ten provinces (excluding Yukon, Northwest Territories and Nunavut) [26]. The CTADS is a well-validated survey with a range of studies being published using the 2017 data [27–30]. We used the question, “Have you ever tried an electronic cigarette, also known as e-cigarette?” to identify all of the 3,059 respondents with an experience of vaping.
Outcome
A binary outcome variable was created to represent vaper type (current vs. former vaper). Respondents were defined as a current vaper if they answered, “every day” or “occasionally” to the question, “At the present time, do you use an electronic cigarette, also known as an e-cigarette every day, occasionally or not at all?” People responding “not at all” to the same question were former vapers.
Candidate correlates
A wide range of person-level characteristics were explored as potential correlates of vaper type. These 46 variables were mostly categorical, except for age and years of smoking that were continuous. These variables described demographics, socioeconomic factors, household information, health, vaping behaviours, substance use and perceived risk of vaping and smoking (see below).
Statistical analysis
We summarized the characteristics of current vs. former vapers and used two-sample tests (Fisher’s exact test, t-test or the Mann-Whitney U-test) to compare their distributions.
Variable selection has been shown to be a necessary procedure prior to classification tree analysis to reduce the risk of model overfitting and spurious associations [20]. Hence, we used each candidate correlate to predict the odds of being a current vaper in a logistic mixed-effects model with a random intercept indicating provincially based random effects. This model estimates an unadjusted fixed effect for each correlate on all individuals and allows this effect to vary across provinces. Correlates associated with a significant fixed effect (using a 2-sided P-value < 0.05) were selected into the machine learning analysis. This procedure was performed on R using the “lme4” package [31].
We followed the Classification and Regression Tree (CART) algorithm to develop a tree model to classify current and former vapers [32] using the R package “rpart” [33]. CART is a non-parametric method that develops a classification tree by recursive partitioning. In this tree, a node is where a splitting variable, X, and one of its level of value, c, divides the dataset into two regions, X ≤ c and X > c (or X = 0 and X = 1 for binary X), that correspond to the predicted classes of current and former vapers. An optimal splitting variable X and value c minimize the Gini Index at a node, which is an impurity criterion that measures how well a split correctly separates true current vapers from true former vapers (i.e. how “pure” the separation is). The splitting procedure terminates when a node contains < 5 data. “Pruning” of a tree is often necessary as a full tree may be excessively large and complicated that leads to model overfitting. Hence, a cross-validation method is used to identify an optimal number of splits that minimizes a cost complexity function of total misclassified cases with a penalized term for larger tree size. This procedure yields a tree with manageable size and interpretable structure while maintaining its performance.
We used a ratio of 9:1 to randomly split the dataset into a training set (n = 2,753) and a validation set (n = 306). The training set was used to develop and to internally validate the model, while the validation set was used to establish model performance externally as it comprised independent data not used in model construction. Using the data from the training set, we first performed oversampling as the number of former vapers significantly exceeded that of current vapers (with a ratio of 4:1), causing concerns on outcome imbalance that may deter model performance. Hence, a random oversampling with replacement was conducted on current vapers on the training set so that their size was increased to be that of former vapers. We then developed a full tree using data from the oversampled training set to classify current and former vapers with the set of correlates selected from the logistic mixed-effects regression analysis. After that, the pruning procedure was performed using a ten-fold cross-validation method. Classification accuracy, specificity and sensitivity of the pruned tree were calculated during the cross-validation process to establish performance of the model on the training set. Finally, the pruned tree was applied to data from the validation set and accuracy, specificity and sensitivity were computed to demonstrate the external performance of the model.
We assessed the performance of two parsimonious trees, including the one that only had the first split of the full tree and another one with the first two splits. This procedure was adopted from a recent machine learning paper that also used a classification tree to predict smoking cessation status in efforts of quantifying the significance of the top predictors in this model [20]. For both parsimonious tree models, we calculated their accuracy, sensitivity and specificity using data from the oversampled training set and from the validation set separately.
Multiple imputation by chained equation [34] was used to address the very small portion of data missing from the dataset (totaled 1.0%). After visually confirming the assumption of missing at random, five imputed data copies were generated independently, and all analytical procedures were repeated on each of these data copies to compare results with our primary findings (Supplementary Material 1). Analyses were performed on R (version 3.5.1).
Results
Sample characteristics
Of the 3,059 Canadians aged 15+ who reported to have tried vaping, their average age was 24.4 [standard deviation (SD) 11.0] years, with 41.9% of them being female, 8.5% of them being aboriginal and 74.3% residing in urban areas (Table 1). A total of 2,503 (81.8%) were former vapers and 556 (18.2%) reported to be current vapers.
Comparing the characteristics of former and current vapers
Characteristics | Former vapers n = 2,503, 81.8% | Current vapers n = 556, 18.2% | Total n = 3,059 | P-value |
---|---|---|---|---|
Age, yr | ||||
Mean ± SD | 24.9 ± 11.1 | 22.5 ± 10.7 | 24.4 ± 11.0 | < 0.001 |
Median (IQR) | 21 (5) | 19 (5) | 21 (6) | < 0.001 |
Female sex | 1,075 (42.9%) | 207 (37.2%) | 1,282 (41.9%) | 0.02 |
Province | 0.09 | |||
Ontario | 461 (18.4%) | 73 (13.1%) | 534 (17.5%) | |
Quebec | 352 (14.1%) | 74 (13.3%) | 426 (13.9%) | |
Manitoba | 227 (9.1%) | 55 (9.9%) | 282 (9.2%) | |
Alberta | 232 (9.3%) | 67 (12.1%) | 299 (9.8%) | |
British Columbia | 191 (7.6%) | 48 (8.6%) | 239 (7.8%) | |
Nova Scotia | 206 (8.2%) | 46 (8.3%) | 252 (8.2%) | |
Saskatchewan | 216 (8.6%) | 42 (7.6%) | 258 (8.4%) | |
New Brunswick | 221 (8.8%) | 54 (9.7%) | 275 (9.0%) | |
Prince Edward Island | 148 (5.9%) | 42 (7.6%) | 190 (6.2%) | |
Newfoundland | 249 (9.9%) | 55 (9.9%) | 304 (9.9%) | |
Aboriginal | 214 (8.7%) | 46 (8.4%) | 260 (8.5%) | 0.91 |
Urban residency | 1,855 (74.1%) | 418 (75.2%) | 2,273 (74.3%) | 0.64 |
Highest education | < 0.001 | |||
Less than high school | 465 (18.6%) | 210 (37.8%) | 675 (22.1%) | |
High school | 1,139 (45.5%) | 221 (39.7%) | 1,360 (44.4%) | |
Non-bachelor certificate | 611 (24.4%) | 90 (16.2%) | 701 (22.9%) | |
Bachelor’s degree or above | 251 (10.0%) | 25 (4.5%) | 276 (9.0%) | |
Currently working | 1,718 (68.6%) | 340 (61.2%) | 2,058 (67.3%) | 0.001 |
Married currently/previously | 475 (19.0%) | 76 (13.7%) | 551 (18.0%) | 0.004 |
Living with a child under 15 | 579 (23.1%) | 154 (27.7%) | 733 (24.0%) | 0.03 |
Smoking allowed inside home | 179 (7.2%) | 41 (7.4%) | 220 (7.2%) | 0.93 |
Vaping allowed inside home | 458 (18.3%) | 153 (27.5%) | 611 (20.0%) | < 0.001 |
Physical health | 0.30 | |||
Excellent | 598 (23.9%) | 116 (20.9%) | 714 (23.3%) | |
Very good or good | 1,740 (69.5%) | 401 (72.1%) | 2,141 (70.0%) | |
Fair or poor | 162 (6.5%) | 39 (7.0%) | 201 (6.6%) | |
Mental health | 0.22 | |||
Excellent | 670 (26.8%) | 148 (26.6%) | 818 (26.7%) | |
Very good or good | 1,551 (62.0%) | 332 (59.7%) | 1,883 (61.6%) | |
Fair or poor | 277 (11.1%) | 76 (13.7%) | 353 (11.5%) | |
Last vaping involved nicotine | < 0.001 | |||
Yes | 1,158 (46.3%) | 342 (61.5%) | 1,500 (49.0%) | |
No | 940 (37.6%) | 182 (32.7%) | 1,122 (36.7%) | |
Unsure | 405 (16.2%) | 32 (5.8%) | 437 (14.3%) | |
Reasons of vaping | ||||
Affordable | 223 (9.0%) | 109 (19.7%) | 332 (10.9%) | < 0.001 |
Allowed at home | 318 (12.8%) | 144 (26.0%) | 462 (15.1%) | < 0.001 |
Less harmful to oneself | 628 (25.3%) | 261 (47.2%) | 889 (29.1%) | < 0.001 |
Less harmful to others | 633 (25.5%) | 256 (46.3%) | 889 (29.1%) | < 0.001 |
Attraction to flavors | 786 (31.7%) | 327 (59.1%) | 1,113 (36.4%) | < 0.001 |
Help to quit smoking | 510 (20.6%) | 202 (36.5%) | 712 (23.3%) | < 0.001 |
Don’t smell | 333 (13.4%) | 146 (26.4%) | 479 (15.7%) | < 0.001 |
Similar to smoking | 219 (8.8%) | 76 (13.7%) | 295 (9.6%) | 0.001 |
Acceptable by non-smokers | 575 (23.2%) | 226 (40.9%) | 801 (26.2%) | < 0.001 |
Curious | 1,995 (80.5%) | 359 (64.9%) | 2,354 (77.0%) | < 0.001 |
Others | 262 (10.5%) | 82 (15.0%) | 345 (11.3%) | 0.003 |
Usual place to get e-cigarettes | < 0.001 | |||
Purchase by oneself | 628 (25.1%) | 246 (44.2%) | 874 (28.6%) | |
Borrow/buy from friends/family | 1,839 (73.5%) | 307 (55.2%) | 2,146 (70.2%) | |
Ever tried one of seven drugs | 829 (33.1%) | 189 (34.0%) | 1,018 (33.3%) | 0.73 |
Recreational use of prescription medications | ||||
Pain reliever | 105 (4.3%) | 35 (6.4%) | 140 (4.6%) | 0.04 |
Sedatives | 320 (12.9%) | 79 (14.4%) | 399 (13.0%) | 0.40 |
Stimulants | 35 (1.4%) | 21 (3.8%) | 56 (1.8%) | < 0.001 |
Having smoked 100 cigarettes in lifetime | 902 (36.0%) | 186 (33.5%) | 1,088 (33.3%) | 0.27 |
History of smoking, yr | ||||
Mean ± SD | 4.1 ± 9.9 | 3.7 ± 9.1 | 4.0 ± 9.8 | 0.30 |
Median (IQR) | 0 (3) | 0 (3) | 0 (3) | 0.02 |
Type of cigarette smoker | < 0.001 | |||
Never smoked | 1,496 (59.8%) | 299 (53.8%) | 1,795 (58.7%) | |
Former smoker | 737 (29.4%) | 215 (38.7%) | 952 (31.1%) | |
Current daily/occasional smoker | 270 (10.8%) | 42 (7.6%) | 312 (10.2%) | |
Ever used other tobacco products | ||||
Cigar or little cigar | 1,556 (62.2%) | 362 (65.1%) | 1,918 (62.7%) | 0.21 |
Tobacco pipe | 304 (12.1%) | 82 (14.7%) | 386 (12.6%) | 0.11 |
Water pipe | 778 (31.1%) | 185 (33.3%) | 963 (31.5%) | 0.34 |
Chewing tobacco | 496 (19.8%) | 147 (26.4%) | 643 (21.0%) | 0.001 |
Type of alcohol drinker | < 0.001 | |||
Never/former drinker | 260 (10.4%) | 45 (8.1%) | 305 (10.0%) | |
Current light drinker | 1,431 (57.2%) | 268 (48.2%) | 1,699 (55.5%) | |
Current heavy drinker | 683 (27.3%) | 203 (36.5%) | 886 (29.0%) | |
Type of cannabis user | < 0.001 | |||
Never used cannabis | 585 (23.4%) | 131 (23.6%) | 716 (23.4%) | |
Former user | 1,222 (48.8%) | 329 (59.2%) | 1,551 (50.7%) | |
Current user | 673 (26.9%) | 89 (16.0%) | 762 (24.9%) | |
Perceived vaping/smoking behaviours to have moderate/great health risk | ||||
Occasional smoking | 1,382 (55.2%) | 297 (53.4%) | 1,679 (54.9%) | 0.49 |
Regular smoking | 2,421 (96.7%) | 545 (98.2%) | 2,966 (97.0%) | 0.11 |
Occasional vaping | 845 (33.8%) | 125 (22.5%) | 970 (31.7%) | < 0.001 |
Regular vaping | 1,758 (70.2%) | 306 (55.0%) | 2,064 (67.5%) | < 0.001 |
Smoking during pregnancy | 2,435 (97.3%) | 537 (96.6%) | 2,972 (97.2%) | 0.85 |
Vaping during pregnancy | 2,119 (84.7%) | 434 (78.1%) | 2,553 (83.5%) | < 0.001 |
The seven drugs include cocaine, heroin, salvia, hallucinogen, ecstasy, solvent and methamphetamine; IQR: inter-quartile range; yr: year
The two groups of vapers differed significantly in their characteristics. Notably, current vapers were younger by 2-year on average (mean age = 22.5 vs. 24.9 years) and had lower education (high school or above: 60.4% vs. 79.9%). They were less likely to be female (37.2% vs. 42.9%), currently working (61.2% vs. 68.6%), having been or currently married (13.7% vs. 19.0%) or living with a child (27.7% vs. 23.1%). The most striking difference between the two groups was their perceived risk of vaping. Current vapers were less likely to consider vaping occasionally (22.5% vs. 33.8%), regularly (55.0% vs. 70.2%) or during pregnancy (78.1% vs. 84.7%) to have moderate/great risks rather than no/slight risks.
Performance of the classification tree
Twenty-nine correlates were identified by the logistic mixed-effects regression analysis to be potentially important (Table 2; full results see Supplementary Material 2).
Significant correlates yielded by the mixed-effects regression analysis
Variables | Reference level | OR | 95% CI | P-value | |
---|---|---|---|---|---|
Age | Per 1-year increase | 0.98 | 0.97–0.99 | < 0.001 | |
Sex | Female vs. male | 0.78 | 0.65–0.95 | < 0.001 | |
Marital status | Currently/previously married vs. never married | 0.67 | 0.52–0.87 | 0.003 | |
Education | High school | vs. less than high school | 0.44 | 0.36–0.55 | < 0.001 |
Non-bachelor certificate | 0.34 | 0.26–0.44 | < 0.001 | ||
Bachelor’s degree or above | 0.23 | 0.15–0.36 | < 0.001 | ||
Currently working | Yes vs. no | 0.72 | 0.60–0.87 | 0.001 | |
Living with children | Yes vs. no | 1.27 | 1.03–1.56 | 0.02 | |
Vaping allowed at home | Yes vs. no | 1.70 | 1.37–2.10 | < 0.001 | |
Type of cigarette smoker | Former smoker | vs. never smoked | 1.47 | 1.20–1.78 | < 0.001 |
Current smoker | 0.78 | 0.55–1.10 | 0.15 | ||
Using nicotine in last vape | Yes | vs. no | 1.52 | 1.24–1.85 | < 0.001 |
Unsure | 0.40 | 0.27–0.60 | < 0.001 | ||
Reasons of vaping | Affordable | Yes vs. no | 2.52 | 1.96–3.25 | < 0.001 |
Allowed at home | 2.48 | 1.97–3.11 | < 0.001 | ||
Less harmful than smoking to oneself | 2.74 | 2.25–3.32 | < 0.001 | ||
Less harmful than smoking to others | 2.61 | 2.15–3.17 | < 0.001 | ||
Attraction to flavors | 3.26 | 2.68–3.97 | < 0.001 | ||
Help to quit smoking | 2.25 | 1.84–2.74 | < 0.001 | ||
Don’t smell | 2.34 | 1.87–2.92 | < 0.001 | ||
Similar to smoking | 1.66 | 1.26–2.20 | < 0.001 | ||
Acceptable to non-smokers | 2.35 | 1.93–2.86 | < 0.001 | ||
Curious | 0.47 | 0.38–0.57 | < 0.001 | ||
Others | 1.50 | 1.15–1.96 | 0.003 | ||
Usual place to get e-cigarettes | Purchase by oneself vs. buy/borrow from family/friends | 2.40 | 1.98–2.90 | < 0.001 | |
Type of cannabis user | Current user | vs. never tried cannabis | 1.20 | 0.96–1.50 | 0.11 |
Former user | 0.59 | 0.44–0.78 | 0.001 | ||
Type of alcohol drinker | Current light drinker | vs. lifetime abstainer and former drinker | 0.85 | 0.65–1.12 | 0.25 |
Current heavy drinker | 1.36 | 1.02–1.80 | 0.04 | ||
Ever tried chewing tobacco | Yes vs. no | 1.45 | 1.17–1.80 | < 0.001 | |
Recreational use of pain medications | Yes vs. no | 1.54 | 1.04–2.28 | 0.03 | |
Recreational use of stimulants | Yes vs. no | 2.79 | 1.61–4.84 | < 0.001 | |
Perceived risks of vaping | Occasionally | Moderate/great risk vs. no/slight risk | 0.57 | 0.46–0.70 | < 0.001 |
Regularly | 0.52 | 0.43–0.62 | < 0.001 | ||
During pregnancy | 0.65 | 0.51–0.81 | < 0.001 |
The mixed-effects models used province of residence as a random intercept; OR: odds ratio; CI: confidence interval
Using these correlates, a classification tree (Figure 1) was developed and pruned with a final form comprising just three predictors-attraction to vaping flavor (yes/no), age (with 18-years being chosen as the optimal threshold; age < 18 or age ≥ 18) and believing vaping was less harmful than smoking to oneself (yes/no). Using cross-validation, the accuracy, sensitivity and specificity of this tree model on the training set was 0.71 (95% CI 0.65–0.77), 0.70 (95% CI 0.61–0.76) and 0.71 (95% CI 0.66–0.73), respectively. Applying this model to data from the validation set yielded accuracy, sensitivity and specificity of 0.72, 0.65 and 0.73.
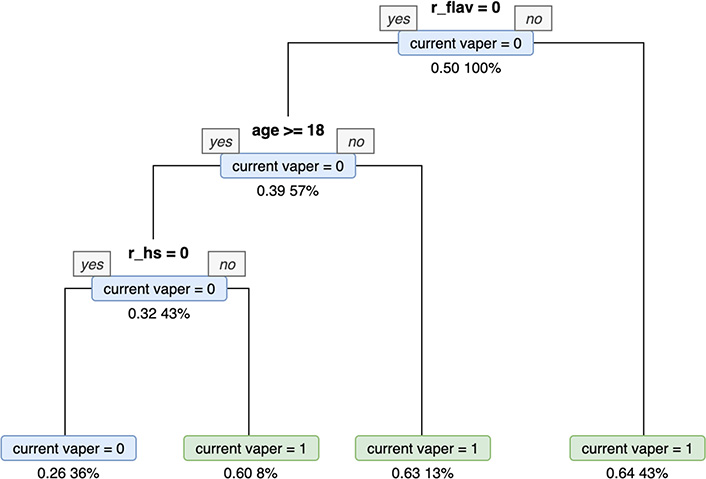
Classification tree. r_flav = 0: if the reason for using e-cigarette is not attraction to flavor, otherwise = 1; r_hs = 0: if the reason for using e-cigarette is not due to a belief that vaping is less harmful to oneself than cigarette smoking, otherwise = 1
Correlates of current vaping and importance
The tree model used attraction to flavor as the first splitting variable, followed by age as the second splitting variable and believing vaping to be less harmful than smoking to oneself as the third splitting variable. People who reported to vape due to flavors were predicted to have the highest probability (0.64) of being a current vaper. Among those who vaped for other reasons, minors with ages < 18 had the second highest probability (0.63) of vaping currently. For adults who did not vape for flavors, their probability of current vaping could reach a high of 0.60 if they believed vaping to be less harmful than cigarette smoking to users and otherwise was a low of 0.26 if they did not have such health belief.
In order to understand the importance of the top two correlates (attraction to flavor and age), we compared the performance of the full tree to two parsimonious trees that comprised only the first split (attraction to flavor) or the first two splits (attraction to flavor and age; Table 3). Using data from the oversampled training set, classification accuracy and specificity were the highest in the full tree, but sensitivity was the highest in the 2-split tree that used only attraction to flavor and age for prediction (sensitivity = 0.74 vs. 0.70). Similar results were observed on the validation set where the 2-split tree exceeded the full tree in terms of sensitivity (sensitivity = 0.67 vs. 0.65). However, in general, improvement of model performance from a 1-split tree to a 2-split tree to the full tree was minor (Table 3).
Performance of the classification tree models using only the first two splits
Performance of the classification tree | Oversampled training setn = 4,506, including 2,253 current vapers and 2,253 former vapers | Validation setn = 306, including 56 current vapers and 250 former vapers | ||||
---|---|---|---|---|---|---|
Tree composition | Accuracy | Sensitivity | Specificity | Accuracy | Sensitivity | Specificity |
First split only | 0.64 | 0.60 | 0.68 | 0.67 | 0.56 | 0.70 |
First two splits | 0.66 | 0.74 | 0.60 | 0.63 | 0.67 | 0.62 |
Full tree | 0.71 | 0.70 | 0.71 | 0.72 | 0.65 | 0.73 |
Sensitivity analysis
Five imputed data copies were generated using the multiple imputation by chained equation method after visual inspection confirmed the assumption of missing at random (Supplementary Material 1). All analytical procedures were repeated on the five imputed datasets and the same classification tree involving attraction to flavor (first-split), age (second-split) and believing vaping to be less harmful than smoking to oneself (third-split) was reached at each iteration. Hence, we conclude the tree model is generally insensitive to missing data.
Discussion
We applied machine learning to data collected from a nationally representative sample of Canadians aged 15+ with vaping experience to understand correlates of the current use of the device. A classification tree model was developed and validated with good performance. This model identified vaping due to attraction to flavors to be the most important correlate of current vaping, followed by young age < 18 and vaping with a belief that it was less harmful to oneself than cigarette smoking. Furthermore, we found strong predictive power of the first two correlates, as the 2-split tree demonstrated comparable performance with the full tree.
Our findings confirmed the vital role of e-cigarette flavors on vaping behaviours. In our sample, attraction to flavors was the second most commonly reported reason for vaping (36.4%), following curiosity (77.0%). This observation coincided with a large body of literature that suggested flavors recruited people, especially young people, to start vaping [12, 15, 35–38]. Furthermore, we found some evidence that the attraction to flavors may motivate long-term vaping, which added to the findings of a recent US study that established flavors to be a key part of vaping addiction [15]. These results provide support for bans on flavored e-cigarettes, as seen in some states in the US. In comparison, Canada is slow to action on curbing the epidemic of flavored vaping. Federal-level regulations have prohibited certain e-cigarette flavors, including those with non-descriptive names (e.g., “Miami Heat”) and are suggestive of health benefits (e.g., vitamin flavor) [39]. A few provinces, including Ontario and Prince Edward Island, have announced plans to ban flavored e-cigarettes in 2021, but at this moment the sale of these products is largely legal in Canada [40, 41]. Our findings suggested a similar ban of all flavored vaping products may be effective in Canada at reducing the uptake and continued use of e-cigarettes.
The classification tree algorithm determined 18-years to be an optimal cut-off value for the age variable and suggested that young people < 18 were associated with high probability of being a current vaper. There are two explanations for this finding: first, it is possible that some of these young people had just started vaping and were thereby more likely to be captured as current vapers in the survey. This speculation could be tested by controlling for a variable that measures the history of vaping, such as the age when started to vape. However, only 17.5% of our sample reported the age at vaping initiation, which impeded us to conduct any additional analysis. Second, it is possible that young age, or in our case, being a youth (aged 15–17), is indeed an important risk factor for long-term vaping. If so, our findings provided new insights into the profile of chronic vapers, which was previously deemed to comprise older, heavy smokers who wished to quit [42]. As youth may also continue vaping in the long run, effective programs that help youth vapers to quit at early phase of e-cigarette use are warranted to reduce their risks of progression to established vaping.
Limitations
Due to the cross-sectional nature of the survey data, we were unable to identify true predictors of current vaping, but rather important correlates. Future study with access to longitudinal data could outcome this limitation. Next, our analysis depends entirely on self-reported measures, which may introduce recall bias. However, we believe that the CTADS survey mechanism has been carefully constructed to ensure sufficient answer time for interviewees to adequately recall long-term memory. Third, there are other factors that we do not have access to, such as household income and neighborhood characteristics, that may influence the pattern of vaping. Future researchers with a more comprehensive tracking of people with vaping experiences, preferably through the use of linked administrative dataset, may provide additional insights. Finally, the data used for this study was collected in 2017, which was before the enactment of the Tobacco and Vaping Products Act (in May 2018) [43] and the legalization of recreational cannabis (in October 2018) [44] in Canada. Future researchers may leverage more recent data to explore the impact of these new regulations on vaping behaviours.
In conclusion, by using a classification tree, we identified attraction to flavors to be one of the most important correlates of current vaping. This finding is relevant to future development of regulations on e-cigarettes as most flavored vaping products are still legal in Canada. Furthermore, interventions that target youths are needed to prevent their e-cigarette uptake and help those who have already initiated vaping to quit.
Abbreviations
CART: | Classification and Regression Tree |
CI: | confidence interval |
CTADS: | Canadian Tobacco, Alcohol, and Drugs Survey |
SD: | standard deviation |
Supplementary materials
The supplementary materials for this article are available at:
Declarations
Author contributions
RF and MC contributed conception and design of the study; RF obtained access to the dataset, performed the statistical analysis and wrote the first draft of the manuscript; NM contributed to methodology. All authors contributed to manuscript revision, read and approved the submitted version.
Conflicts of interest
The authors declare that they have no conflicts of interest.
Ethical approval
Not applicable.
Consent to participate
Not applicable.
Consent to publication
Not applicable.
Availability of data and materials
The dataset analyzed for this study is maintained by the Computing in the Humanities and Social Science (CHASS) at the University of Toronto available from: http://datacentre.chass.utoronto.ca/
Funding
This work was supported by the Canadian Institutes of Health Research, Catalyst Grant #172898. The funder had no role in the study design, collection, analysis or interpretation of the data, writing the manuscript, or the decision to submit the paper for publication.
Copyright
© The Author(s) 2021.